Collaborative learning for hyperspectral image classification.
NEUROCOMPUTING(2018)
摘要
Recently, collaborative learning (CL) is introduced to combine active learning (AL) with semi-supervised learning (SSL), and solve the problem of limited training samples. In this paper, we proposed a novel CL framework for hyperspectral image classification, in which AL and SSL are collaboratively integrated using clustering (CLUC). CLUC attempts to obtain more diversity and higher confidence of additional training samples in both AL and SSL. Note that clustering methods, which are used separately to enhance AL or SSL, are utilized to integrate these two learning process in CLUC. First, all unlabeled samples are assigned into clusters. Based on the clustering result, clustering-based query (CBQ) for both AL and SSL, and CBQ-based pseudo-labeling (CBQPL) for SSL are designed for CLUC. Second, the most and secondary uncertain samples in each cluster are selected by CBQ for AL and SSL, respectively, to ensure their informativeness. Third, CBQPL assigns the selected secondary uncertain samples with the same label as the most uncertain one, which is manually-labeled in AL within the same cluster. CBQPL makes the confidence of pseudo-labeling rely on the clustering results. We evaluate the performance of CLUC on three real hyperspectral images. The performance of the proposed method is tested under different numbers of labeled samples and compared with several approaches. We can observe from the experimental results that CLUC have superiority in classification maps and objective metrics with limited training samples. (C) 2017 Elsevier B.V. All rights reserved.
更多查看译文
关键词
Hyperspectral image classification,Collaborative learning,Active learning,Clustering,Semi-supervised learning
AI 理解论文
溯源树
样例
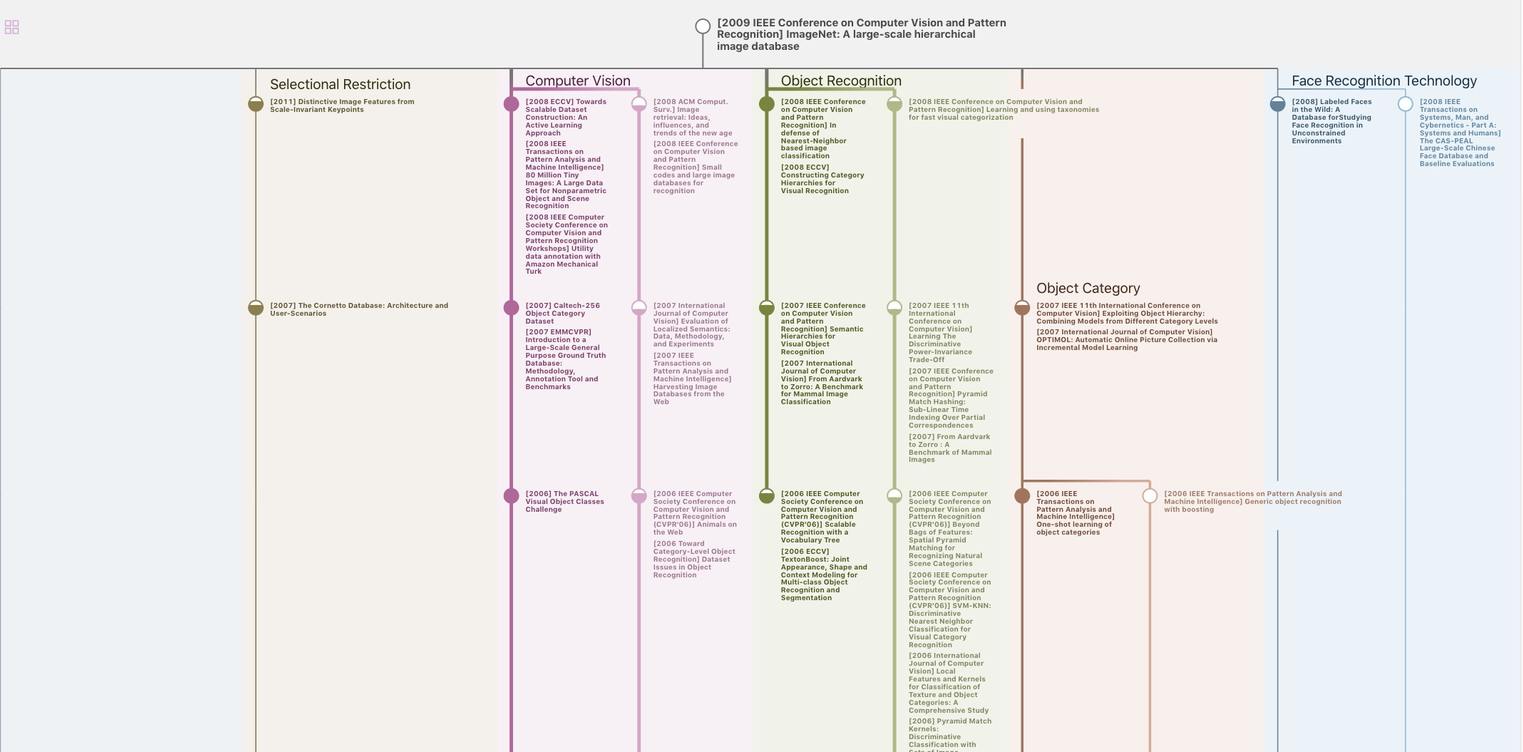
生成溯源树,研究论文发展脉络
Chat Paper
正在生成论文摘要