An overview on Restricted Boltzmann Machines.
NEUROCOMPUTING(2018)
摘要
The Restricted Boltzmann Machine (RBM) has aroused wide interest in machine learning fields during the past decade. This review aims to report the recent developments in theoretical research and applications of the RBM. We first give an overview of the general RBM from the theoretical perspective, including stochastic approximation methods, stochastic gradient methods, and preventing overfitting methods. And then this review focuses on the RBM variants which further improve the learning ability of the RBM under general or specific applications. The RBM has recently been extended for representational learning, document modeling, multi-label learning, weakly supervised learning and many other tasks. The RBM and RBM variants provide powerful tools for representing dependency in the data, and they can be used as the basic building blocks to create deep networks. Apart from the Deep Belief Network (DBN) and the Deep Boltzmann Machine (DBM), the RBM can also be combined with the Convolutional Neural Network (CNN) to create deep networks. This review provides a comprehensive view of these advances in the RBM together with its future perspectives. (C) 2017 Elsevier B.V. All rights reserved.
更多查看译文
关键词
Restricted Boltzmann Machine,Classification,Representational learning,Deep networks
AI 理解论文
溯源树
样例
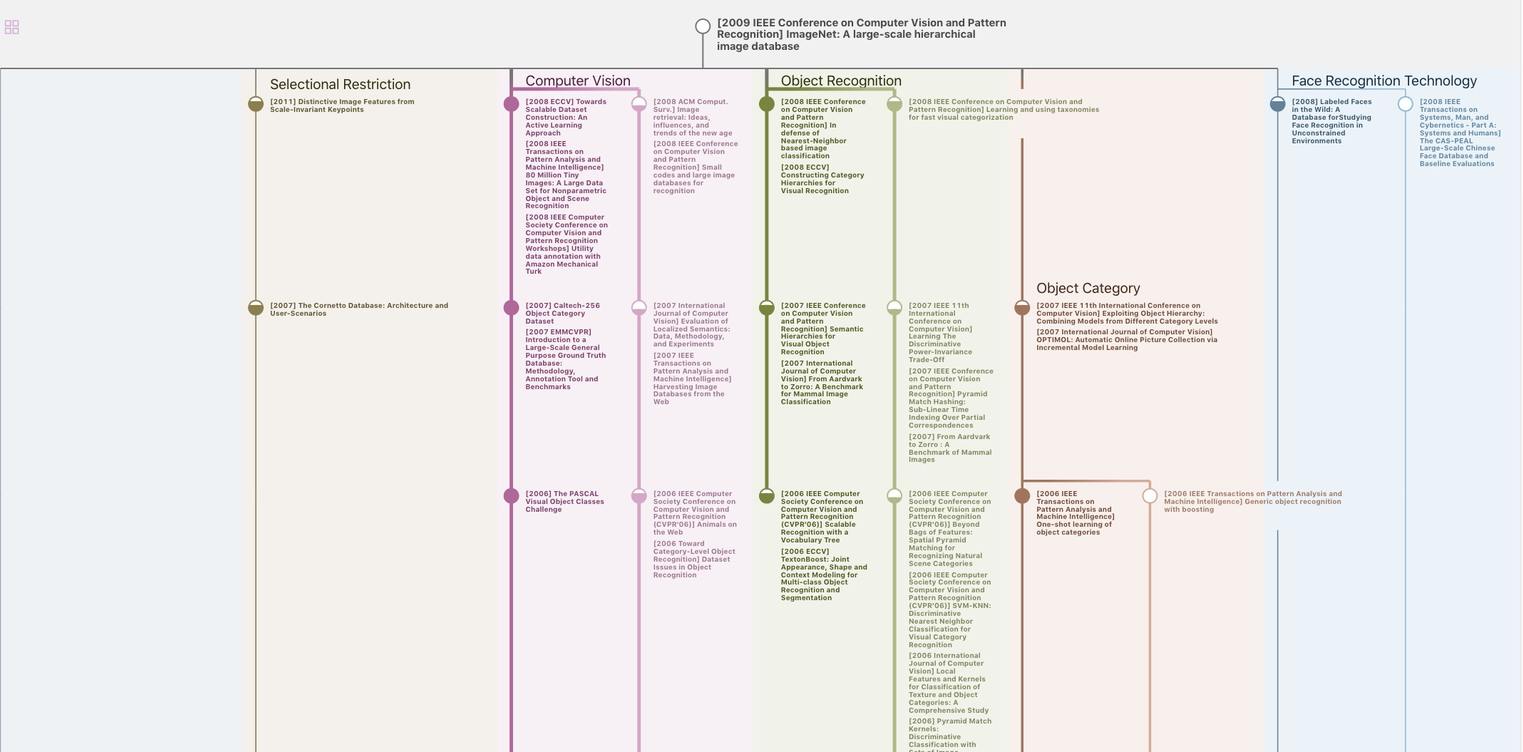
生成溯源树,研究论文发展脉络
Chat Paper
正在生成论文摘要