Deep and Broad Learning on Content-Aware POI Recommendation
2017 IEEE 3rd International Conference on Collaboration and Internet Computing (CIC)(2017)
摘要
POI recommendation has attracted lots of research attentions recently. There are several key factors that need to be modeled towards effective POI recommendation - POI properties, user preference and sequential momentum of check- ins. The challenge lies in how to synergistically learn multi-source heterogeneous data. Previous work tries to model multi-source information in a flat manner, using either embedding based methods or sequential prediction models in a cross-related space, which cannot generate mutually reinforce results. In this paper, a deep and broad learning approach based on a Deep Context- aware POI Recommendation (DCPR) model was proposed to structurally learn POI and user characteristics. The proposed DCPR model includes three collaborative layers, a CNN layer for POI feature mining, an RNN layer for sequential dependency and user preference modeling, and an interactive layer based on matrix factorization to jointly optimize the overall model. Experiments over three data sets demonstrate that DCPR model achieves significant improvement over state-of-the-art POI recommendation algorithms and other deep recommendation models.
更多查看译文
关键词
Spatial Temporal Modeling,Embedding,POI Recommendation
AI 理解论文
溯源树
样例
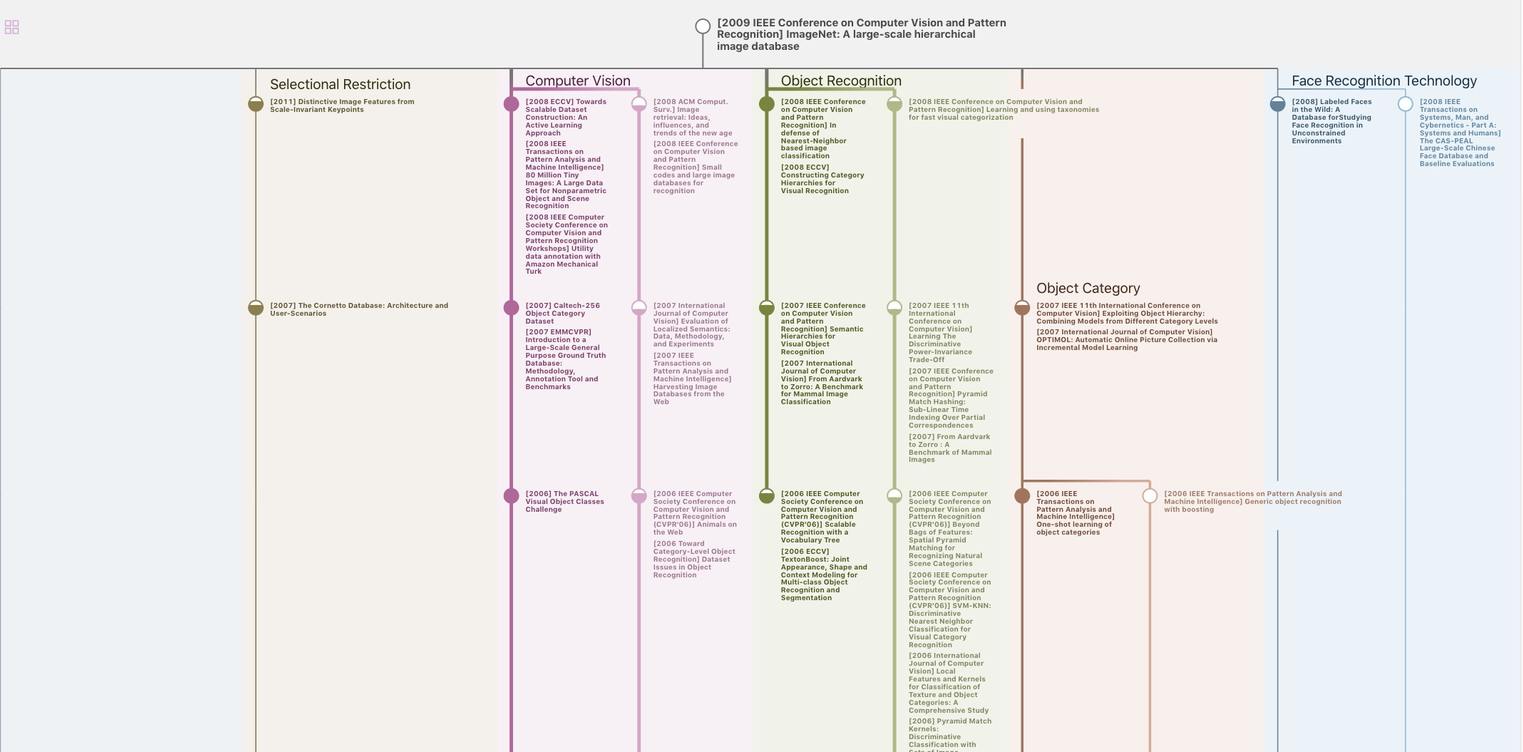
生成溯源树,研究论文发展脉络
Chat Paper
正在生成论文摘要