Long-Short Term Memory Network for RNA Structure Profiling Super-Resolution.
Lecture Notes in Computer Science(2017)
摘要
Profiling of RNAs improves understanding of cellular mechanisms, which can be essential to cure various diseases. It is estimated to take years to fully characterize the three-dimensional structure of around 200,000 RNAs in human using the mutate-and-map strategy. In order to speed up the profiling process, we propose a solution based on super-resolution. We applied five machine learning regression methods to perform RNA structure profiling super-resolution, i. e. to recover the whole data sets using self-similarity in low-resolution (undersampled) data sets. In particular, our novel Interaction Encoded Long-Short Term Memory (IELSTM) network can handle multiple distant interactions in the RNA sequences. When compared with ridge regression, LASSO regression, multilayer perceptron regression, and random forest regression, IELSTM network can reduce the mean squared error and the median absolute error by at least 33% and 31% respectively in three RNA structure profiling data sets.
更多查看译文
关键词
Long-short term memory,RNA structure,Machine learning regression methods
AI 理解论文
溯源树
样例
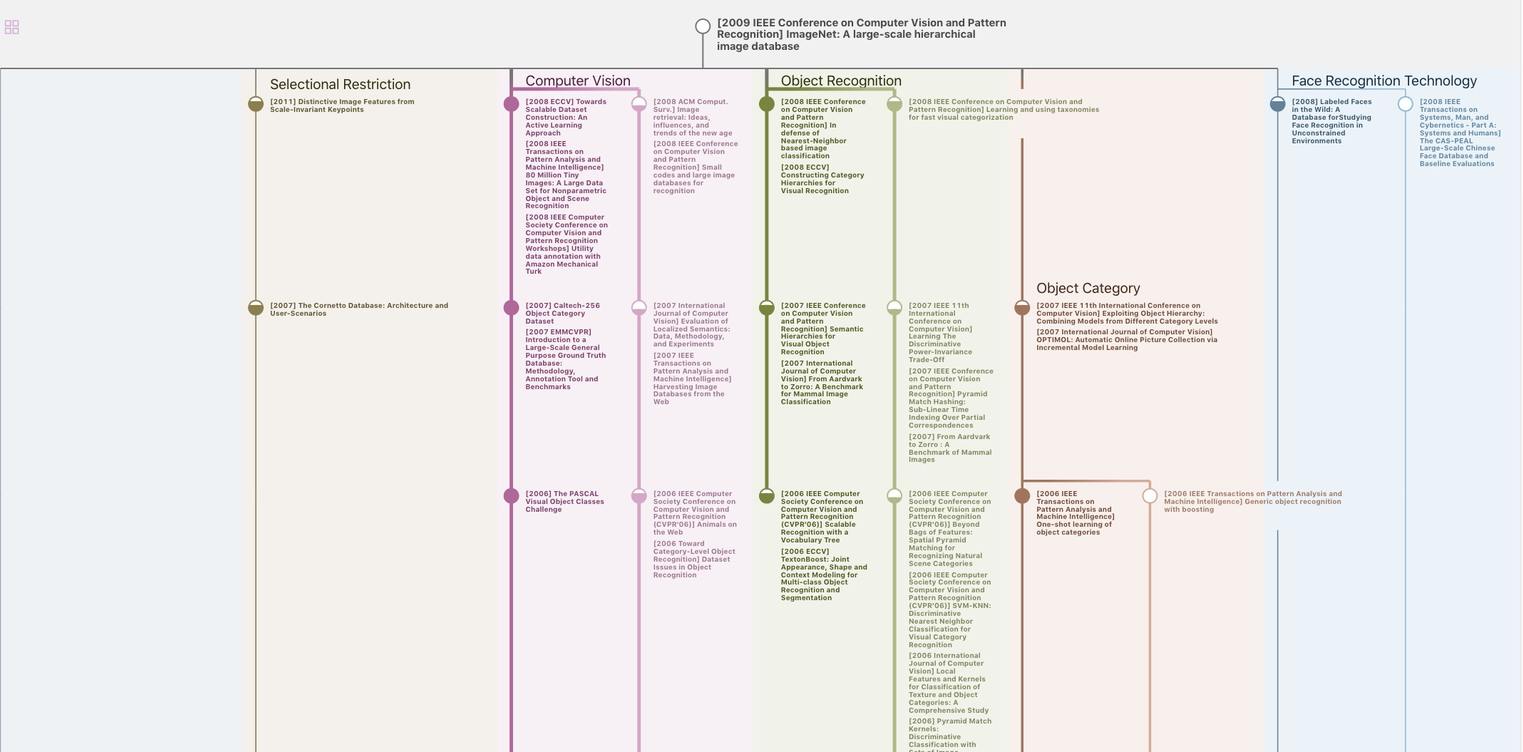
生成溯源树,研究论文发展脉络
Chat Paper
正在生成论文摘要