Prediction Of Lower-Limb Joint Kinematics From Surface Emg During Overground Locomotion
2017 IEEE INTERNATIONAL CONFERENCE ON SYSTEMS, MAN, AND CYBERNETICS (SMC)(2017)
摘要
Recent advancements in powered lower limb prostheses have led to the development of neural-machine interfaces for natural control during bipedal locomotion. In particular, electromyography (EMG) patterns recorded from the amputated limb can be leveraged to infer the intended gait pattern of the user. However, the optimal control strategy for translating the EMG patterns to kinematic space remains a challenge. In this study, six able bodied subjects were instrumented for mobile brain-body imaging and asked to walk on a multi-terrain gait course. A non-linear extension of the Kalman filter was used to predict knee and ankle joint kinematics from lower limb muscle activation patterns during overground locomotion. Specifically, muscles of the anterior and posterior thigh were used to predict both the knee and ankle joint position. The results revealed that muscles in the thigh can be used to predict the position of the knee and ankle with high accuracy. The highest mean r-value obtained for each of the six subjects was 0.92, 0.77, 0.38, 0.39, 0.63, and 0.77, with corresponding SNR values of 10.8 dB, 6.7 dB, 5.9 dB, 2.8 dB, 9.1 dB, and 9.2 dB, for each subject, respectively. This study is the first to demonstrate that continuous EMG can be used to predict the joint kinematics of the knee and ankle during overground locomotion. This approach may provide improvements during closed-loop control of a powered lower limb prosthesis when compared to other pattern-recognition based methods.
更多查看译文
关键词
Electromyography (EMG), myoelectric, lower-limb prosthesis, Unscented Kalman Filter (UKF)
AI 理解论文
溯源树
样例
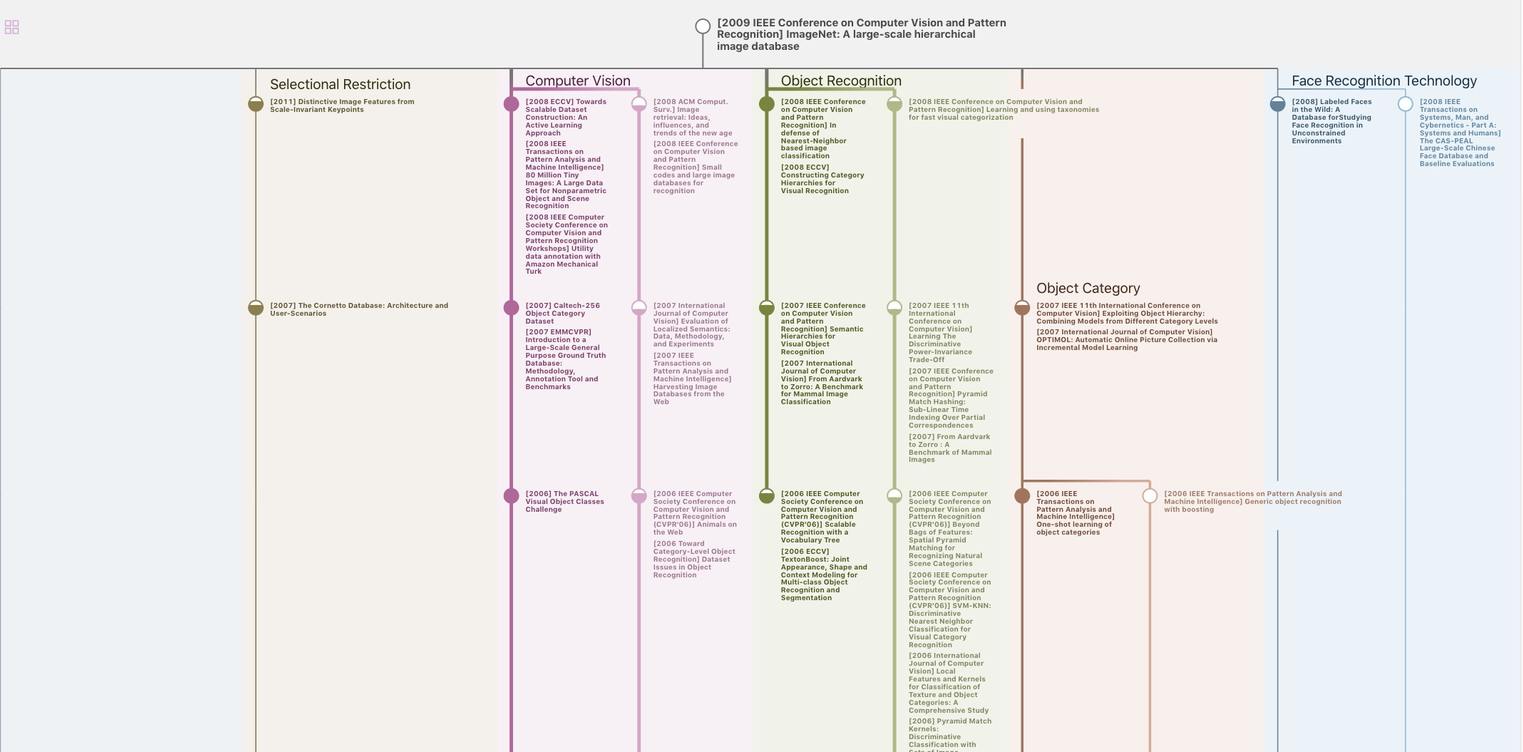
生成溯源树,研究论文发展脉络
Chat Paper
正在生成论文摘要