Scalable Constrained Spectral Clustering Via The Randomized Projected Power Method
2017 17TH IEEE INTERNATIONAL CONFERENCE ON DATA MINING (ICDM)(2017)
摘要
Constrained spectral clustering is an important area with many applications. However, most previous work has only been applied to relatively small data sets: graphs with thousands of points. This prevents this work from being applied to the large data sets found in application domains such as medical imaging and document data. Recent work on constrained and unconstrained spectral clustering has explored scalability of these methods via data approximations such as the Nystrom method which requires the selection of landmarks. However, compressing a graph may lead to undesirable results and poses the additional problem of how to chose landmarks. Instead in this paper, we propose a fast and scalable numerical algorithmic solution lOr the constrained clustering problem. We show the convergence and stability of our approach by proving its rate of convergence and demonstrate the effectiveness of our algorithm with empirical results on several real data sets. Our approach achieved comparable accuracy as popular constrained spectral clustering algorithms but taking several hundred times less time.
更多查看译文
关键词
Clustering,Spectral,Constraints,Constrained Clustering
AI 理解论文
溯源树
样例
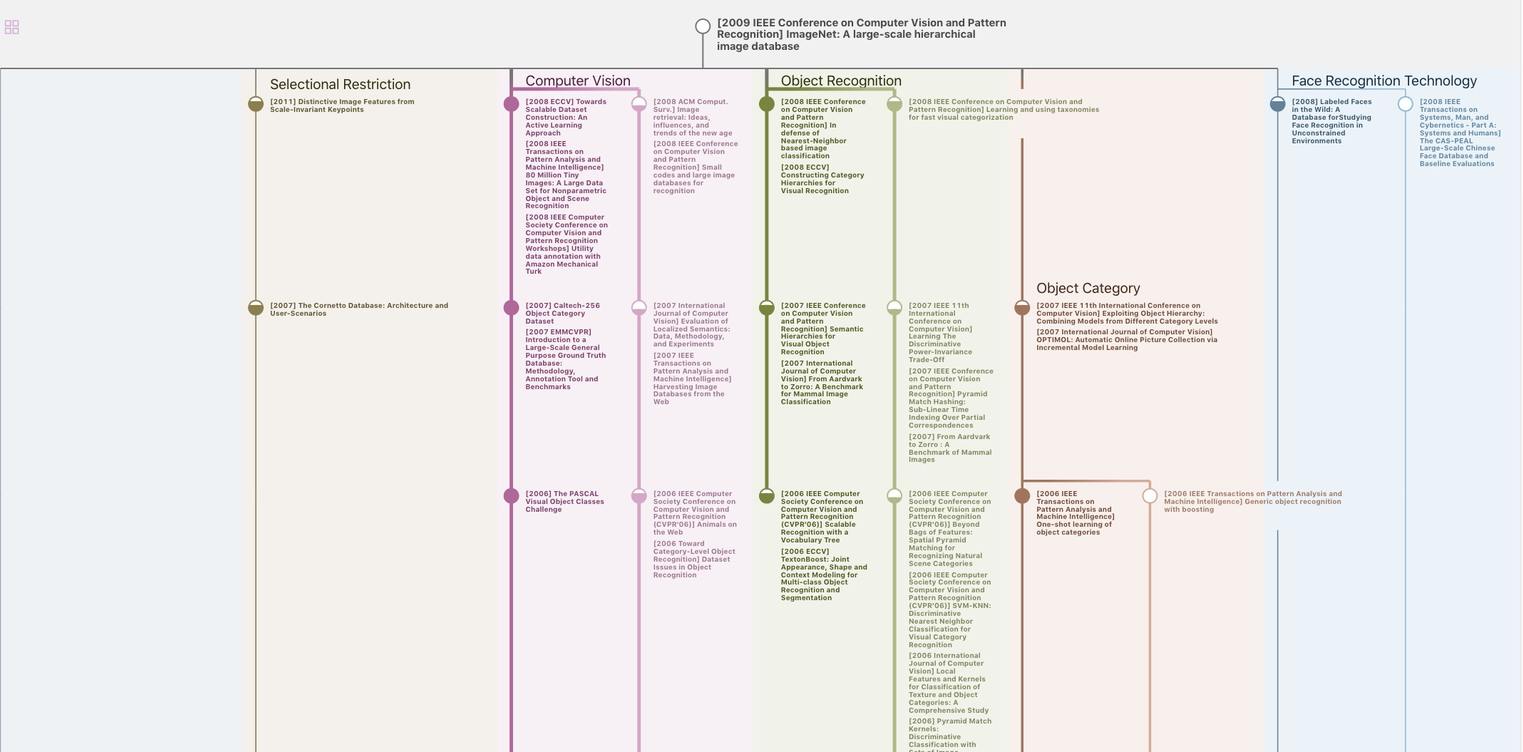
生成溯源树,研究论文发展脉络
Chat Paper
正在生成论文摘要