Transfer learning in neural networks: an experience report.
CASCON(2017)
摘要
Perhaps the most important characteristic of deep neural networks is their ability to discover and extract the necessary features for a particular machine learning task from a raw input representation. This requires a significant time commitment, both in terms of assembling the training dataset, and training the neural network. Reusing the knowledge inherent in a trained neural network for a machine learning task in a related domain can provide significant improvements in terms of the time required to complete the task.In this paper, we present our experience with such a transfer learning situation. We reuse a neural network that was trained on a real world image dataset, for the task of classifying music in terms of genre, instrumentation, composer etc. (audio files are converted to spectrograms for this purpose). Even though the image and music domains are not directly related, our experiments show that features extracted to recognize images allow for high accuracy in many music classification tasks.
更多查看译文
AI 理解论文
溯源树
样例
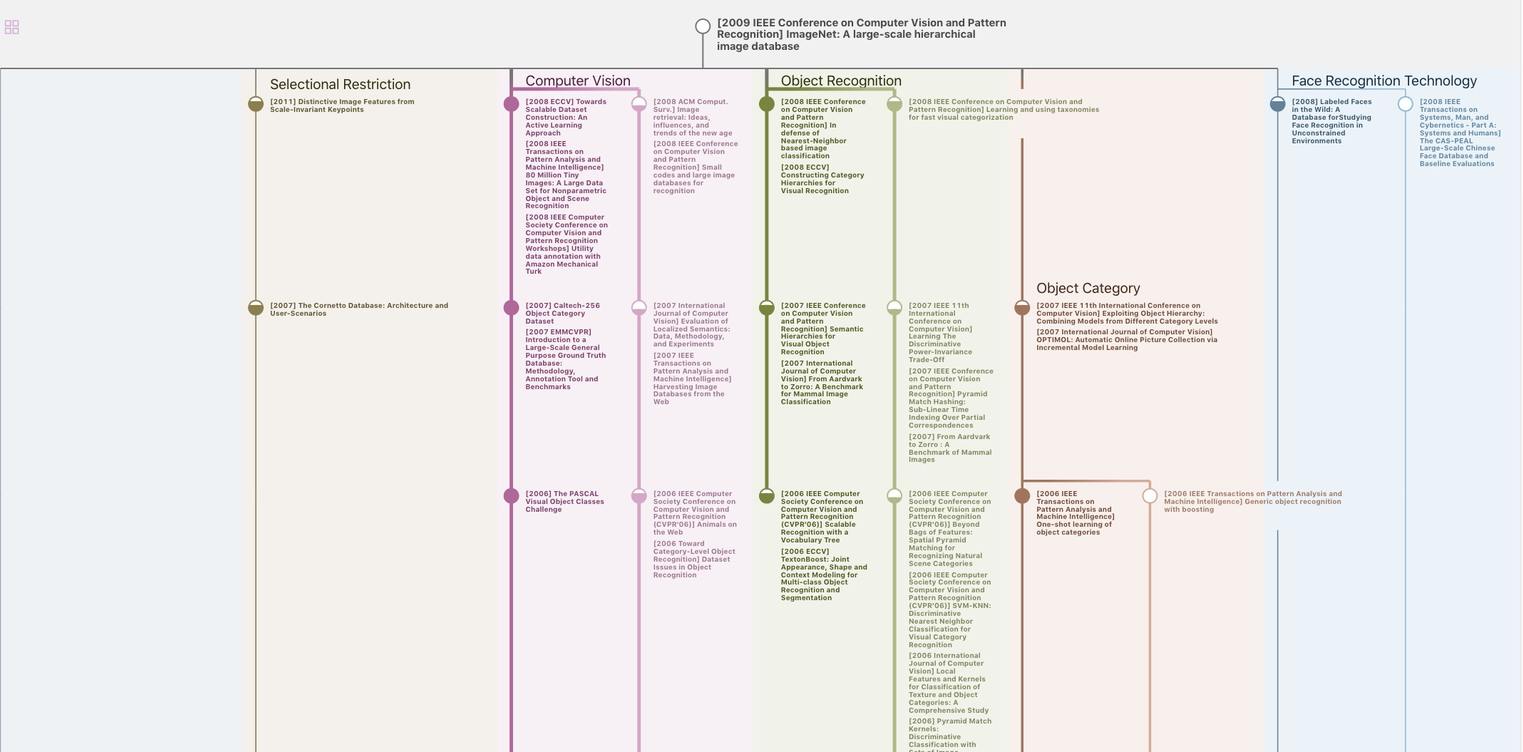
生成溯源树,研究论文发展脉络
Chat Paper
正在生成论文摘要