Validity Index For Gaussian-Distributed Clusters Of Different Sizes With Various Degrees Of Dispersion And Overlap
PROCEEDINGS OF 2017 INTERNATIONAL CONFERENCE ON MACHINE LEARNING AND CYBERNETICS (ICMLC), VOL 2(2017)
摘要
A cluster validity index is to evaluate the correct number of clusters when partitioning a dataset. In this paper, we propose a new cluster validity index based on two measures called dispersion and overlap for Gaussian-distributed clusters. The dispersion measure is used to estimate the situation of data spreading in a cluster. A small dispersion measure for a cluster means that data points are distributed closely in that cluster. The overlap measure represents the degree of overlap between any pair of clusters in the dataset. By combining these two metrics, we obtain a very effective new cluster validity index. Several experiments were conducted to demonstrate the effectiveness of our validity index by exercising eight synthetic datasets and four real datasets. The results show that our validity index can correctly find the optimal number of clusters that may widely differ in size, dispersion and overlapping. As compared to other ten cluster validity indices, our new index has the best performance in term of accuracy.
更多查看译文
关键词
Cluster Validity Index, Gaussian-distributed Cluster, Dispersion Measure, Overlap Measure
AI 理解论文
溯源树
样例
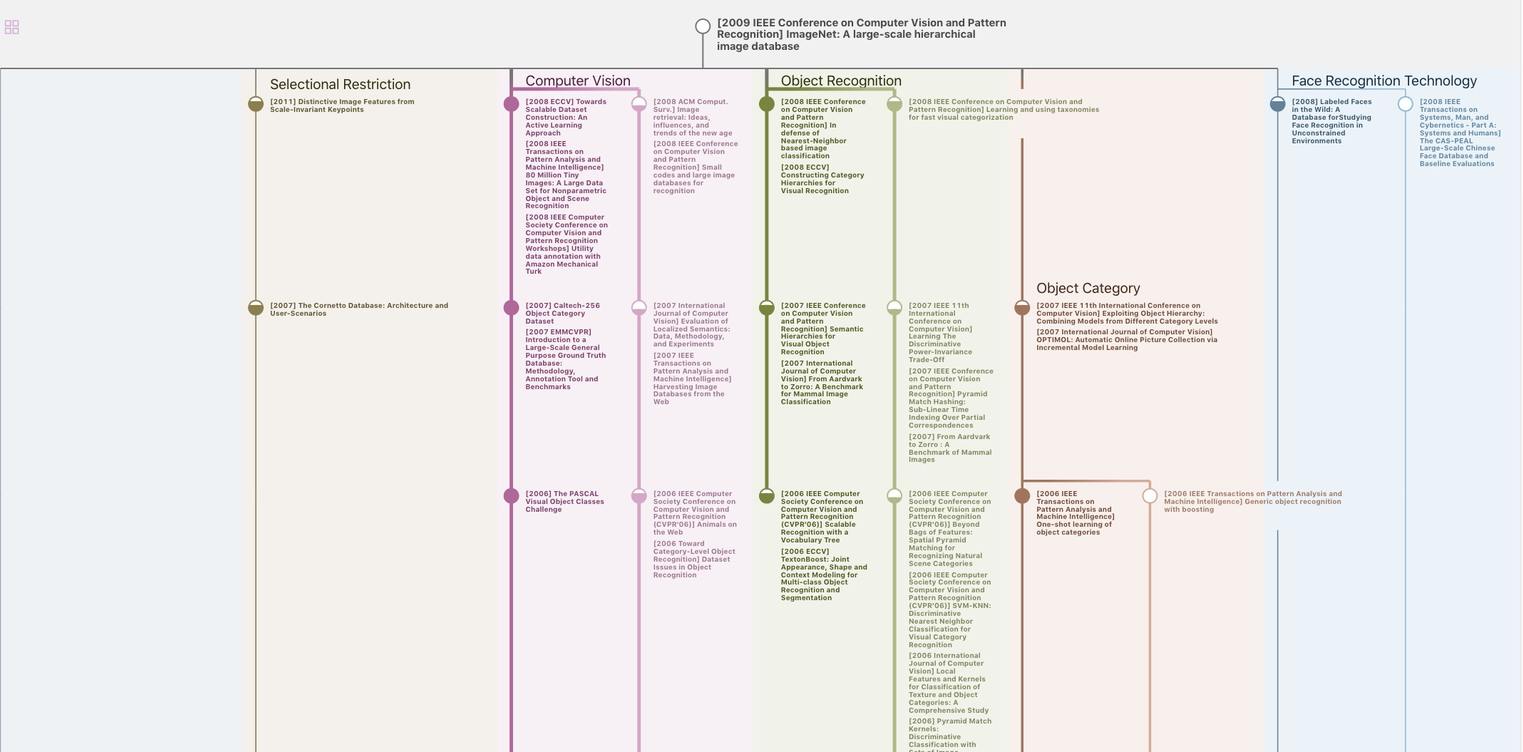
生成溯源树,研究论文发展脉络
Chat Paper
正在生成论文摘要