Traffic Prediction Based Power Saving In Cellular Networks: A Machine Learning Method
25TH ACM SIGSPATIAL INTERNATIONAL CONFERENCE ON ADVANCES IN GEOGRAPHIC INFORMATION SYSTEMS (ACM SIGSPATIAL GIS 2017)(2017)
摘要
In smart cities, green cellular networks play a crucial role to support wireless access for numerous devices anywhere and anytime with efficiency and sustainability. Because base stations (BSes) consume more than 70% of overall cellular network infrastructure energy, saving the power consumption of BSes is the key task to build a green cellular network. Except for low power design of the BS hardware and software, the traffic-driven BS sleeping operation is an economical way to improve existing cellular networks, which can reduce the BS power consumption at low traffic load. However, prior BS sleeping strategies establish on the static temporal characteristics of traffic load, which ignore the fact that network traffic is influenced by many factors such as time, human mobility, holiday, weather, etc. Hence, prior traffic estimation is coarse, and the BS sleeping strategies cannot apply to the changing network traffic. In this paper, we exploit a machine learning method to estimate the BS traffic and propose a BS sleeping strategy based on predicted traffic for power saving in the cellular network. We analyze network traffic in multi-views: temporal influence, spatial influence, and event influence. Then, we propose a multi-view ensemble learning model to predict network traffic load, which learns the traffic in multi-views and combine the results with ensemble. Furthermore, we formulate a BS sleeping strategy based on the predicted traffic load. Finally, we evaluate our traffic prediction algorithm on real cellular network data. The evaluation shows that our traffic prediction algorithm improves about 40% than state-of-the-art machine learning methods. Also, we evaluate the proposed BS sleeping strategy, which yields about 10% more energy savings and less device damage than the competitors in the simulated environment.
更多查看译文
关键词
Smart City,Green Cellular Network,Network Traffic Prediction,Multi-view Learning,Spatio-Temporal Data Analysis
AI 理解论文
溯源树
样例
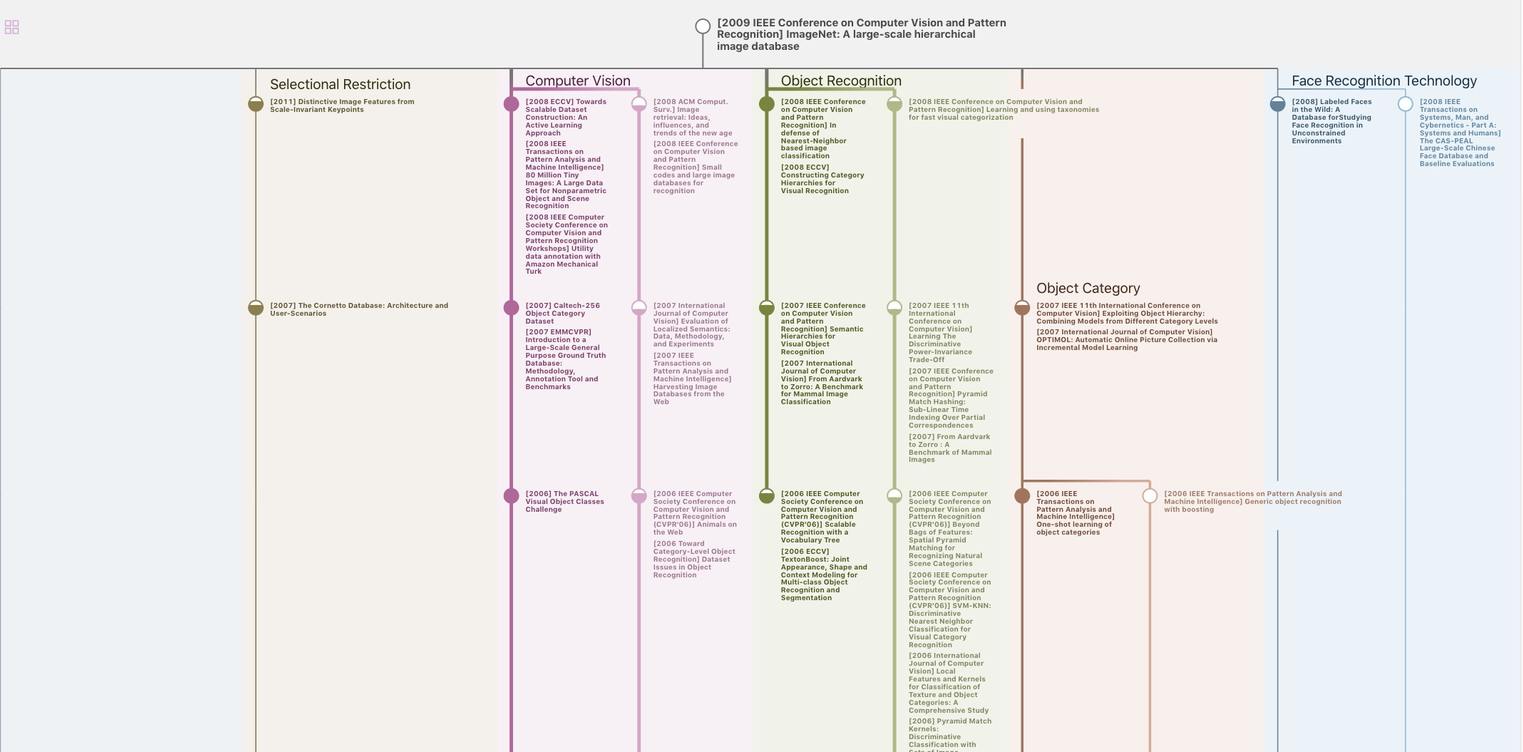
生成溯源树,研究论文发展脉络
Chat Paper
正在生成论文摘要