A Uniform Representation For Trajectory Learning Tasks
25TH ACM SIGSPATIAL INTERNATIONAL CONFERENCE ON ADVANCES IN GEOGRAPHIC INFORMATION SYSTEMS (ACM SIGSPATIAL GIS 2017)(2017)
摘要
Most trajectory data are collected with a constant sample rate (e.g. GPS data). However, the variance of velocities can be very large, which causes the non-uniformity of the sample points in trajectory dataset. That is, the trajectory dataset can be very sparse in some parts which cause most existing distance measures to get unexpected results. On the other hand, the dataset can be extremely dense in some other parts which results in unnecessarily high computational complexity. Due to the above phenomenon, choosing an appropriate sample rate becomes a difficult challenge. In order to address the dilemma, we propose a Step-Invariant Trajectory (SIT) representation that can provide a dynamic sample rate to represent any trajectories in a uniform way. The translation takes only linear time. We also propose an effective and scalable distance measure for SIT representation. We evaluate the effectiveness and efficiency of our representation along with its distance measure by performing multiple trajectory classification and clustering experiments. These results show that our distance measures on SIT representation is much more accurate and robust than other representations and distance measures on sparse trajectory datasets. Our approach can also achieve competitive accuracy compared with the state of the art model -based trajectory representations on dense datasets. However, the time required to translate the data to our representation is 2 orders of magnitude faster, on average, than translate to other model-based representations. Furthermore, our representation can also serve as a preprocessing step to provide high quality input to all trajectory learning methods.
更多查看译文
关键词
Trajectory Representation,Distance Measure,Classification,Clustering
AI 理解论文
溯源树
样例
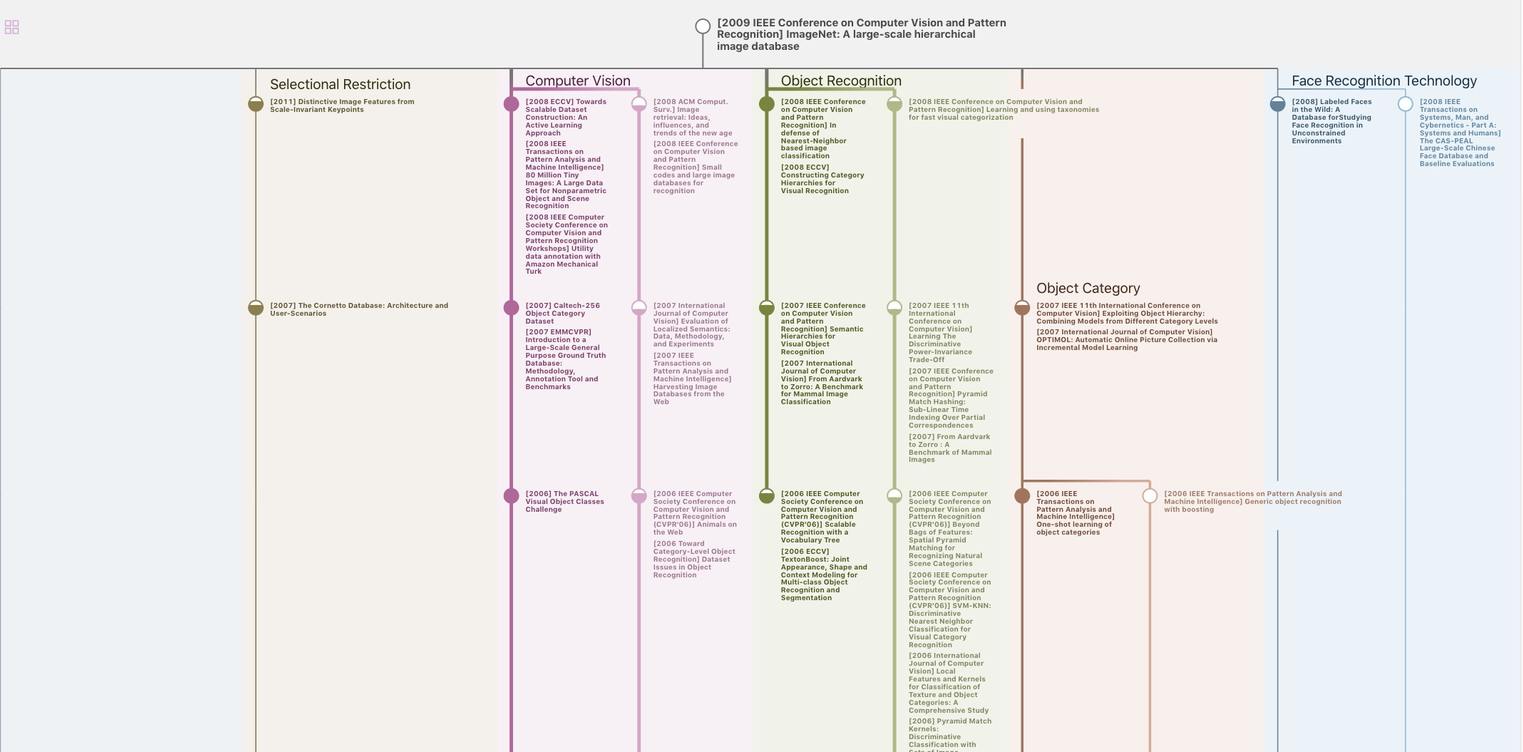
生成溯源树,研究论文发展脉络
Chat Paper
正在生成论文摘要