Chemical-Induced Disease Extraction Via Convolutional Neural Networks With Attention
2017 IEEE INTERNATIONAL CONFERENCE ON BIOINFORMATICS AND BIOMEDICINE (BIBM)(2017)
摘要
Extracting relationships between chemicals and diseases from unstructured literature is very important for many biomedical applications such as pharmacovigilance and drug repositioning. Automatic chemical-induced disease extraction is usually recognized as a classification task, and several systems have been proposed for this task recently due to some annotated corpora publicly available. Most of the systems are based on machine learning methods with many manually-crafted features. In recent years, deep learning that does not only can avoid verbose feature engineering but also shows competitive performance has been widely used in various types of tasks, including classification task. Therefore, deep learning has great potential on chemical-induced disease extraction. In this paper, we proposed an architecture of convolutional neural networks (CNN) with attention mechanism for chemical-induced disease extraction, which does not only avoid verbose feature engineering but also integrates domain knowledge in a simple way. Experiments on a benchmark dataset demonstrate that the proposed CNN-based chemical-induced disease extraction system is competitive with other state-of-the-art systems.
更多查看译文
关键词
chemical-induced disease extraction, convolutional neural networks, attention mechanism Introduction
AI 理解论文
溯源树
样例
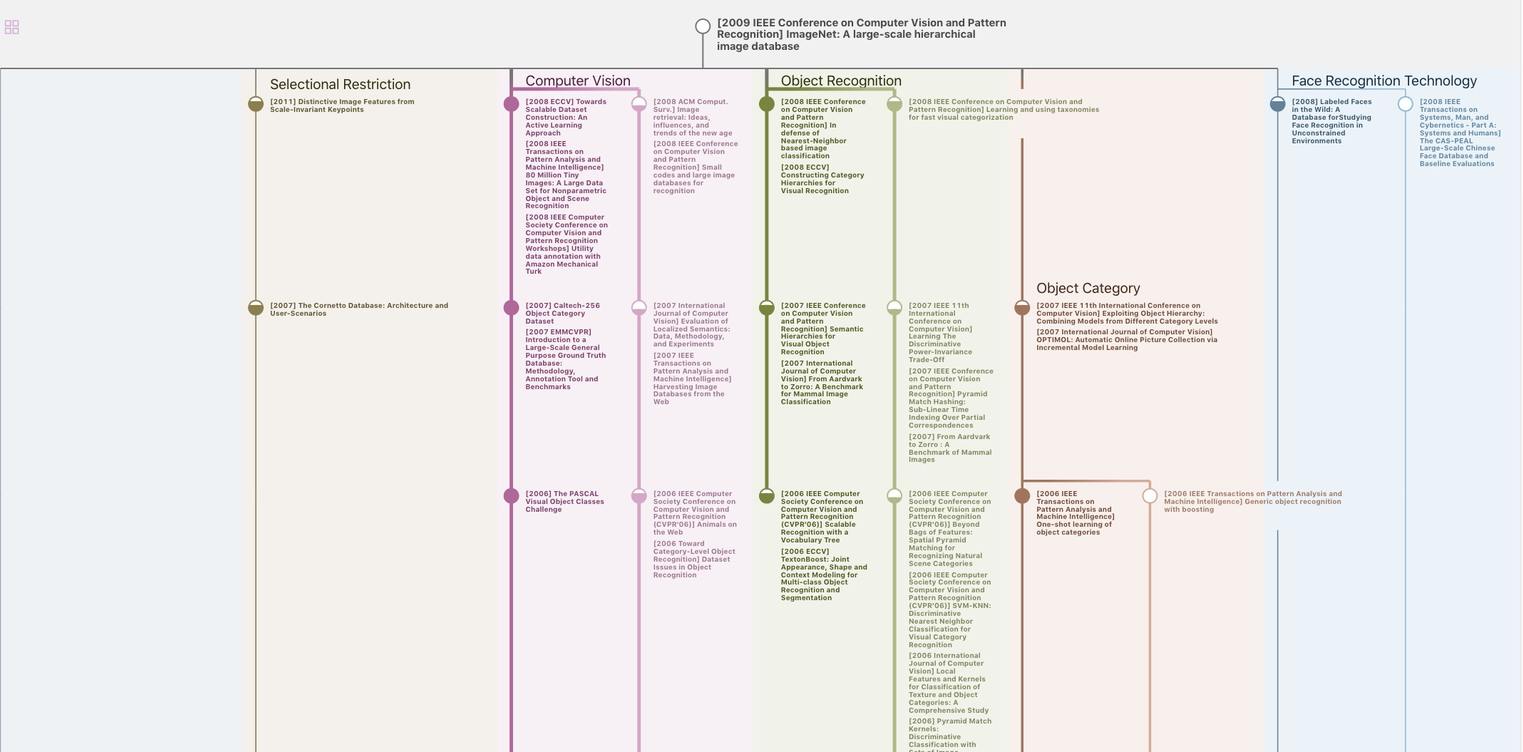
生成溯源树,研究论文发展脉络
Chat Paper
正在生成论文摘要