Nonlinear Statistical Retrieval Of Surface Emissivity From Iasi Data
2017 IEEE INTERNATIONAL GEOSCIENCE AND REMOTE SENSING SYMPOSIUM (IGARSS)(2017)
摘要
Emissivity is one of the most important parameters to improve the determination of the troposphere properties (thermodynamic properties, aerosols and trace gases concentration) and it is essential to estimate the radiative budget. With the second generation of infrared sounders, we can estimate emissivity spectra at high spectral resolution, which gives us a global view and long-term monitoring of continental surfaces. Statistically, this is an ill-posed retrieval problem, with as many output variables as inputs. We here propose nonlinear multi-output statistical regression based on kernel methods to estimate spectral emissivity given the radiances. Kernel methods can cope with high-dimensional input-output spaces efficiently. We give empirical evidence of models performance on Infrared Atmospheric Sounding Interferometer (IASI) simulated data. Kernel regression model largely improves previous least squares linear regression model quantitatively, with an average reduction of 25% in mean-square error.
更多查看译文
关键词
models performance,Infrared Atmospheric Sounding Interferometer simulated data,kernel regression model,nonlinear statistical retrieval,surface emissivity,IASI data,troposphere properties,aerosols,trace gases concentration,radiative budget,infrared sounders,emissivity spectra,high spectral resolution,long-term monitoring,continental surfaces,retrieval problem,output variables,multioutput statistical regression,kernel methods,spectral emissivity,high-dimensional input-output spaces
AI 理解论文
溯源树
样例
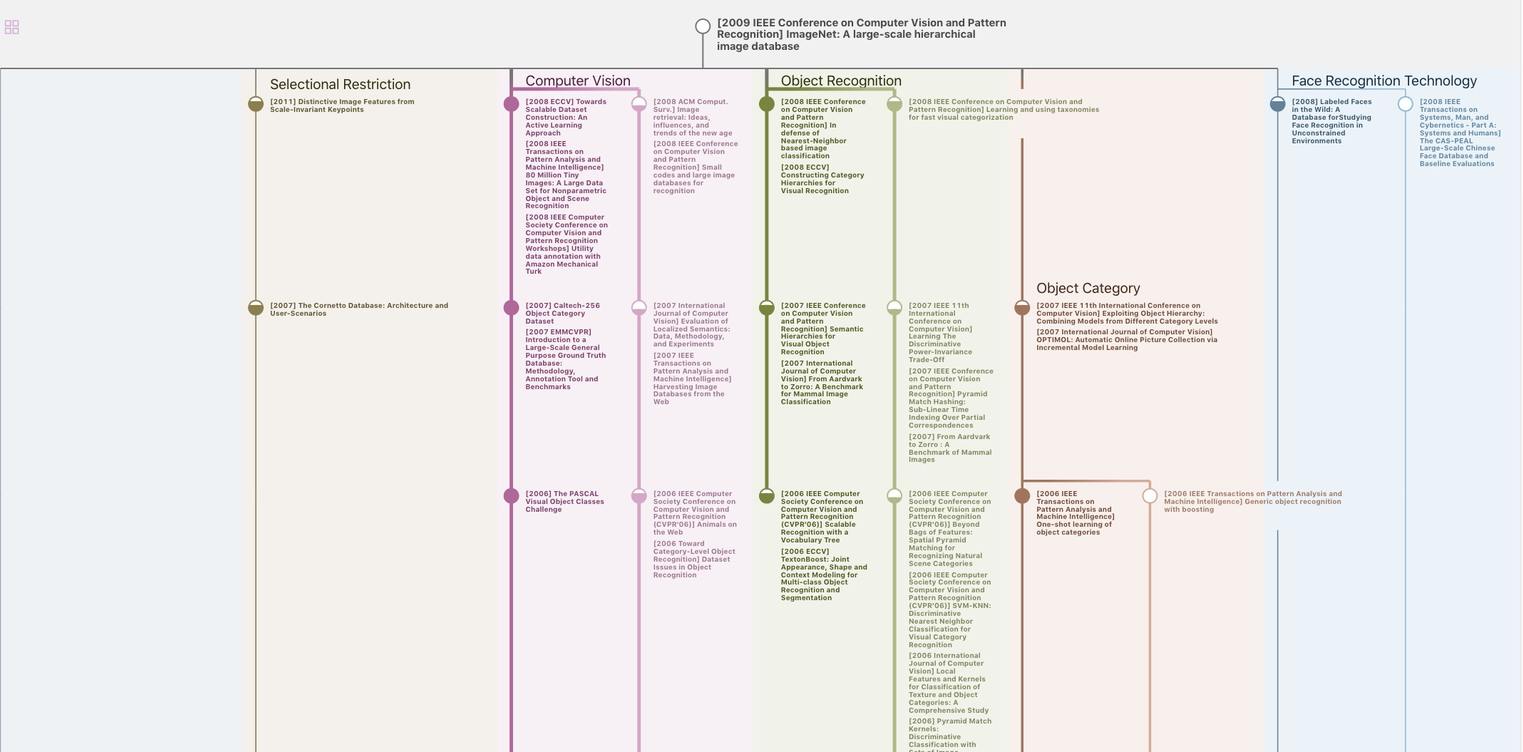
生成溯源树,研究论文发展脉络
Chat Paper
正在生成论文摘要