Nonnegative Sparse Autoencoder For Robust Endmember Extraction From Remotely Sensed Hyperspectral Images
2017 IEEE INTERNATIONAL GEOSCIENCE AND REMOTE SENSING SYMPOSIUM (IGARSS)(2017)
摘要
Endmember extraction is a fundamental task in spectral un-mixing of remotely sensed hyperspectral images. In this work, we develop a new robust algorithm for endmember extraction which is based on a nonnegative sparse autoencoder. The proposed approach is based on two main steps. First, it uses an automatic sampler approach with local outlier factor and affinity propagation to intelligently gather a set of training samples. Then, a set of endmember signatures are extracted from the selected training samples by the nonnegative sparse autoencoder. Taking advantage from both automatic sampling and nonnegative sparse autoencoding, the proposed method can tackle problems with outliers. The effectiveness of the proposed method is verified by using simulated data. In our comparison with other state-of-the-art endmember extraction methods, the proposed approach demonstrates highly competitive performance.
更多查看译文
关键词
Hyperspectral unmixing, endmember extraction, automatic sampler, nonnegative sparse autoencoder
AI 理解论文
溯源树
样例
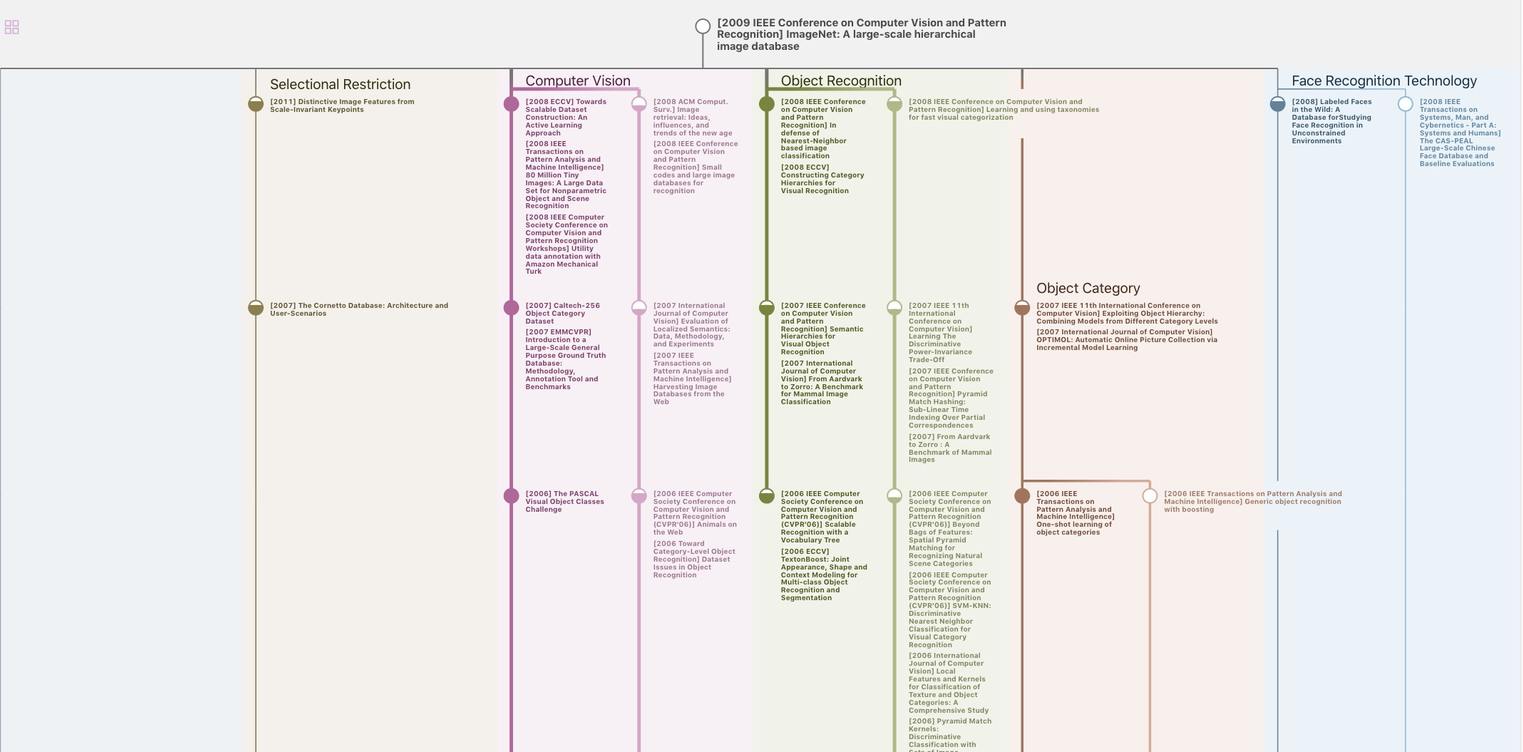
生成溯源树,研究论文发展脉络
Chat Paper
正在生成论文摘要