Using Gradients And Tensor Voting In 3d Local Geometric Descriptors For Feature Detection In Airborne Lidar Point Clouds In Urban Regions
2017 IEEE INTERNATIONAL GEOSCIENCE AND REMOTE SENSING SYMPOSIUM (IGARSS)(2017)
摘要
Structural or geometric classification of three-dimensional (3D) point clouds of urban regions from airborne LiDAR enables feature (object-based) classification, and 3D reconstruction. Here, we consider positive semidefinite symmetric second-order tensors as local geometric descriptors (LGDs), which gives structural classification. We compute LGDs using local neighborhood, and their eigenvalue-based features are conventionally used for object-based classification and 3D reconstruction. We combine derivative based 2D gradient energy tensor, and anisotropically diffused 3D voting tensor, using a multi-scale approach, to compute a LGD. We represent LGDs as second-order tensors, and compare the relevant eigenvalue-based features and saliency maps obtained from them. We visually compare the outcomes of our LGD with conventionally used covariance matrix.
更多查看译文
关键词
Structure tensor, Tensor voting, Eigenvalue-based features, 3D reconstruction
AI 理解论文
溯源树
样例
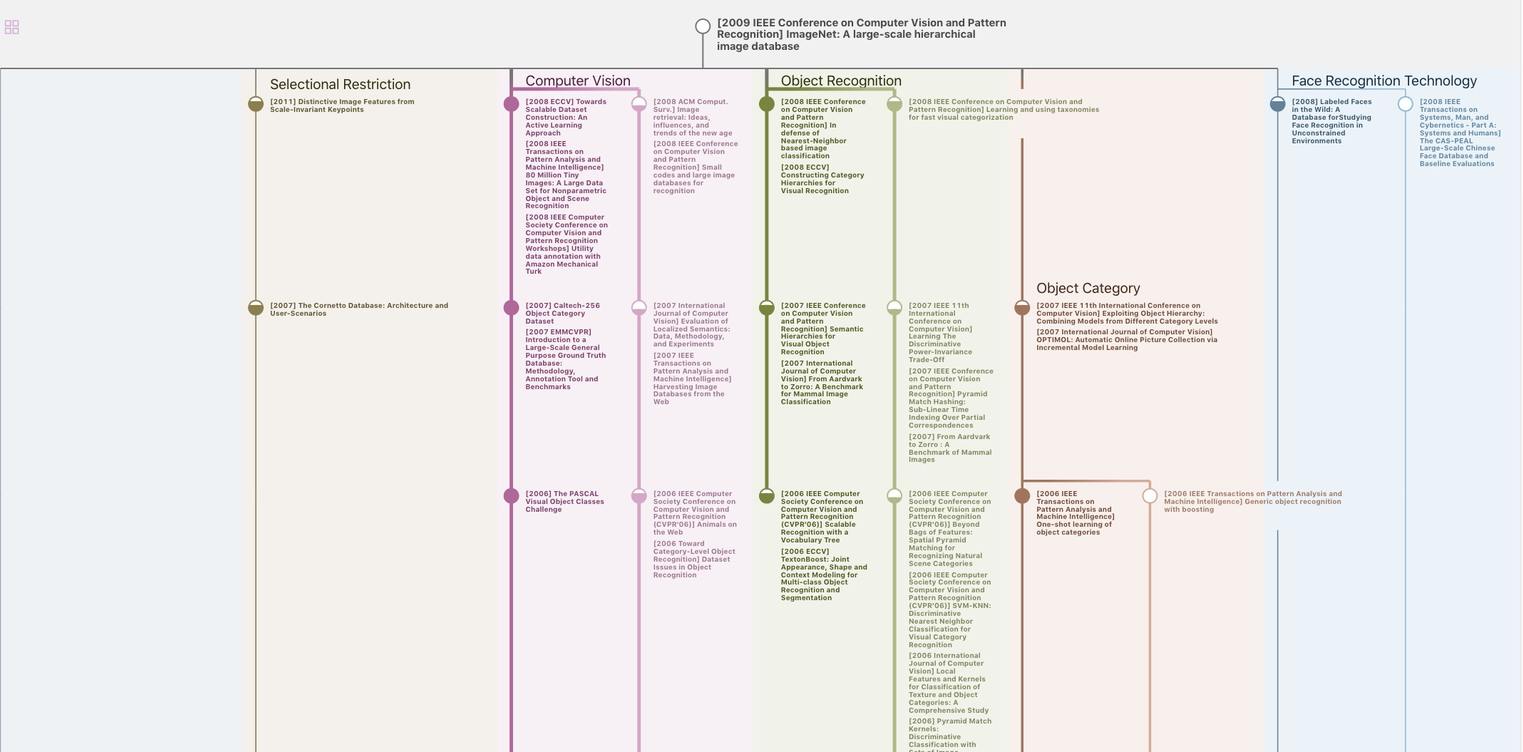
生成溯源树,研究论文发展脉络
Chat Paper
正在生成论文摘要