Sensor Fusion for Robot Control through Deep Reinforcement Learning
2017 IEEE/RSJ INTERNATIONAL CONFERENCE ON INTELLIGENT ROBOTS AND SYSTEMS (IROS)(2017)
摘要
Deep reinforcement learning is becoming increasingly popular for robot control algorithms, with the aim for a robot to self-learn useful feature representations from unstructured sensory input leading to the optimal actuation policy. In addition to sensors mounted on the robot, sensors might also be deployed in the environment, although these might need to be accessed via an unreliable wireless connection. In this paper, we demonstrate deep neural network architectures that are able to fuse information coming from multiple sensors and are robust to sensor failures at runtime. We evaluate our method on a search and pick task for a robot both in simulation and the real world.
更多查看译文
关键词
feature representations,multiple sensors,deep neural network architectures,optimal actuation policy,unstructured sensory input,robot control algorithms,deep reinforcement learning,sensor fusion
AI 理解论文
溯源树
样例
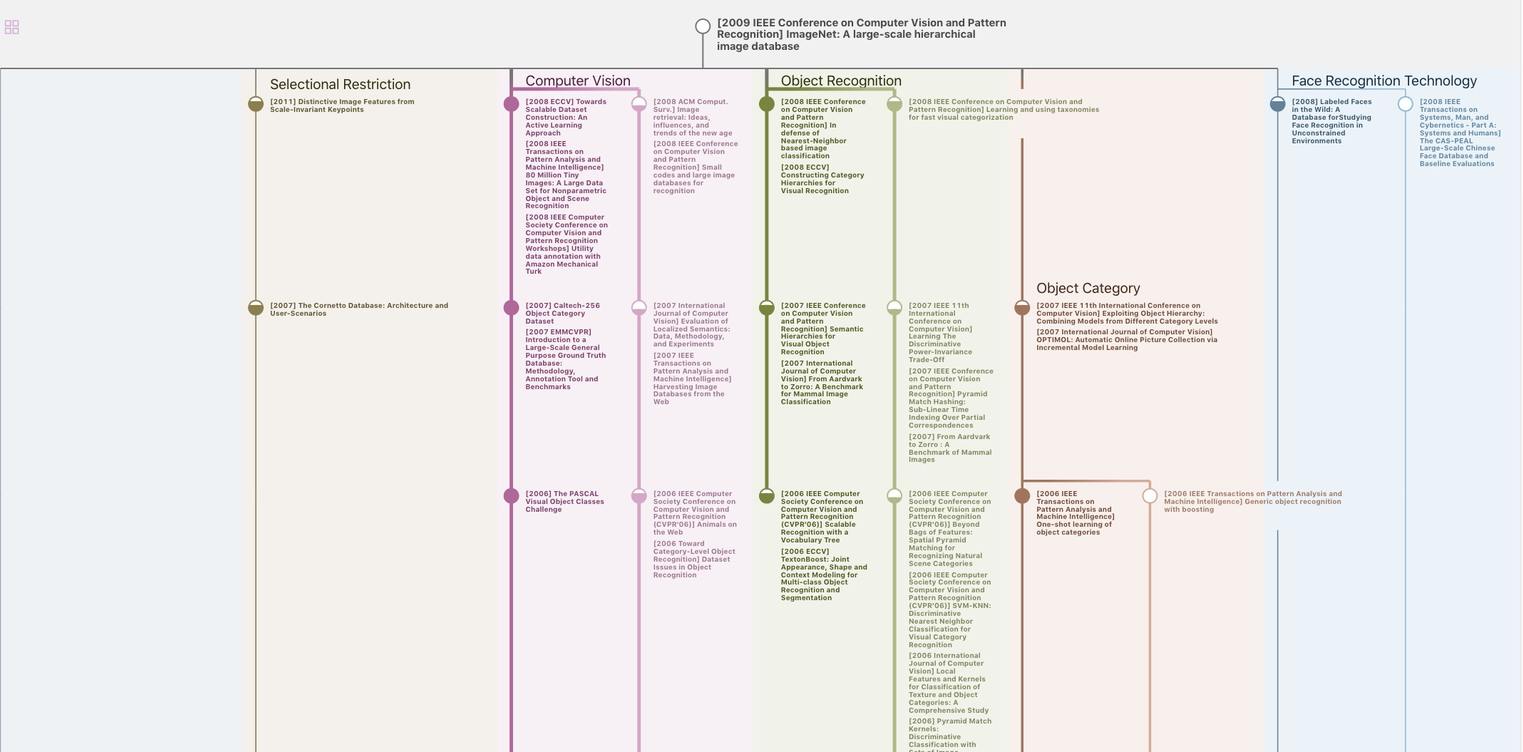
生成溯源树,研究论文发展脉络
Chat Paper
正在生成论文摘要