Looking Forward: A Semantic Mapping System for Scouting with Micro-Aerial Vehicles
2017 IEEE/RSJ INTERNATIONAL CONFERENCE ON INTELLIGENT ROBOTS AND SYSTEMS (IROS)(2017)
摘要
The last decade has seen a massive growth in applications for Micro-Aerial Vehicles (MAVs), due in large part to their versatility for data gathering with cameras, LiDAR and various other sensors. Their ability to quickly go from assessing large spaces from a high vantage points to flying in close to capture high-resolution data makes them invaluable for applications where we are interested in a specific target with an a priori unknown location, e.g. survivors in disaster response scenarios, vehicles in surveillance, animals in wildlife monitoring, etc., a task we will refer to scouting. Our ultimate goal is to enable MAVs to perform autonomous scouting. In this paper, we describe a semantic mapping system designed to support this goal. The system maintains a 2.5D map describing its belief about the location of semantic classes of interest, using forward-looking cameras and state estimation. The map is continuously updated on the fly, using only onboard processing. The system couples a deep learning 2D semantic segmentation algorithm with a novel mapping method to project and aggregate the 2D semantic measurements into a global 2.5D grid map. We train and evaluate our segmentation method on a novel dataset of cars labelled in oblique aerial imagery. We also study the performance of the mapping system in isolation. Finally, we show the integrated system performing a fully autonomous car scouting mission in the field.
更多查看译文
关键词
dataset,wildlife monitoring,onboard processing,state estimation,forward-looking cameras,deep learning 2D semantic segmentation algorithm,disaster response scenarios,high-resolution data,high vantage points,data gathering,MAVs,microaerial vehicles,semantic mapping system,fully autonomous car scouting mission,integrated system,oblique aerial imagery,2.5D grid map,2D semantic measurements
AI 理解论文
溯源树
样例
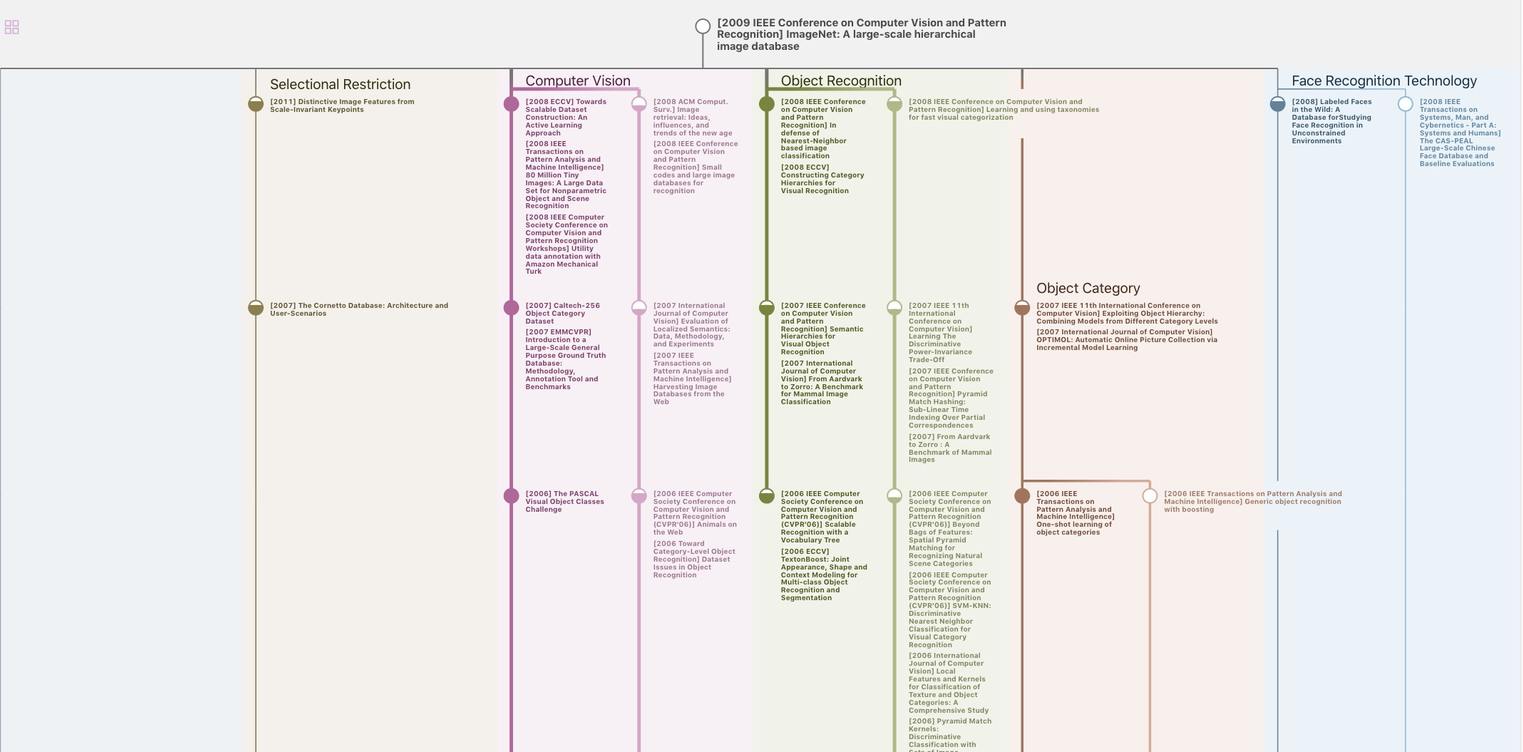
生成溯源树,研究论文发展脉络
Chat Paper
正在生成论文摘要