Quantifying The Impact Of Adversarial Evasion Attacks On Machine Learning Based Android Malware Classifiers
2017 IEEE 16TH INTERNATIONAL SYMPOSIUM ON NETWORK COMPUTING AND APPLICATIONS (NCA)(2017)
摘要
With the proliferation of Android-based devices, malicious apps have increasingly found their way to user devices. Many solutions for Android malware detection rely on machine learning; although effective, these are vulnerable to attacks from adversaries who wish to subvert these algorithms and allow malicious apps to evade detection. In this work, we present a statistical analysis of the impact of adversarial evasion attacks on various linear and non-linear classifiers, using a recently proposed Android malware classifier as a case study. We systematically explore the complete space of possible attacks varying in the adversary's knowledge about the classifier; our results show that it is possible to subvert linear classifiers (Support Vector Machines and Logistic Regression) by perturbing only a few features of malicious apps, with more knowledgeable adversaries degrading the classifier's detection rate from 100% to 0% and a completely blind adversary able to lower it to 12%. We show non-linear classifiers (Random Forest and Neural Network) to be more resilient to these attacks. We conclude our study with recommendations for designing classifiers to be more robust to the attacks presented in our work.
更多查看译文
关键词
adversarial evasion attacks,machine learning,android malware classifiers,Android-based devices,malicious apps,user devices,Android malware detection,linear classifiers,Support Vector Machines,nonlinear classifiers,Logistic Regression,Random Forest,Neural Network,statistical analysis
AI 理解论文
溯源树
样例
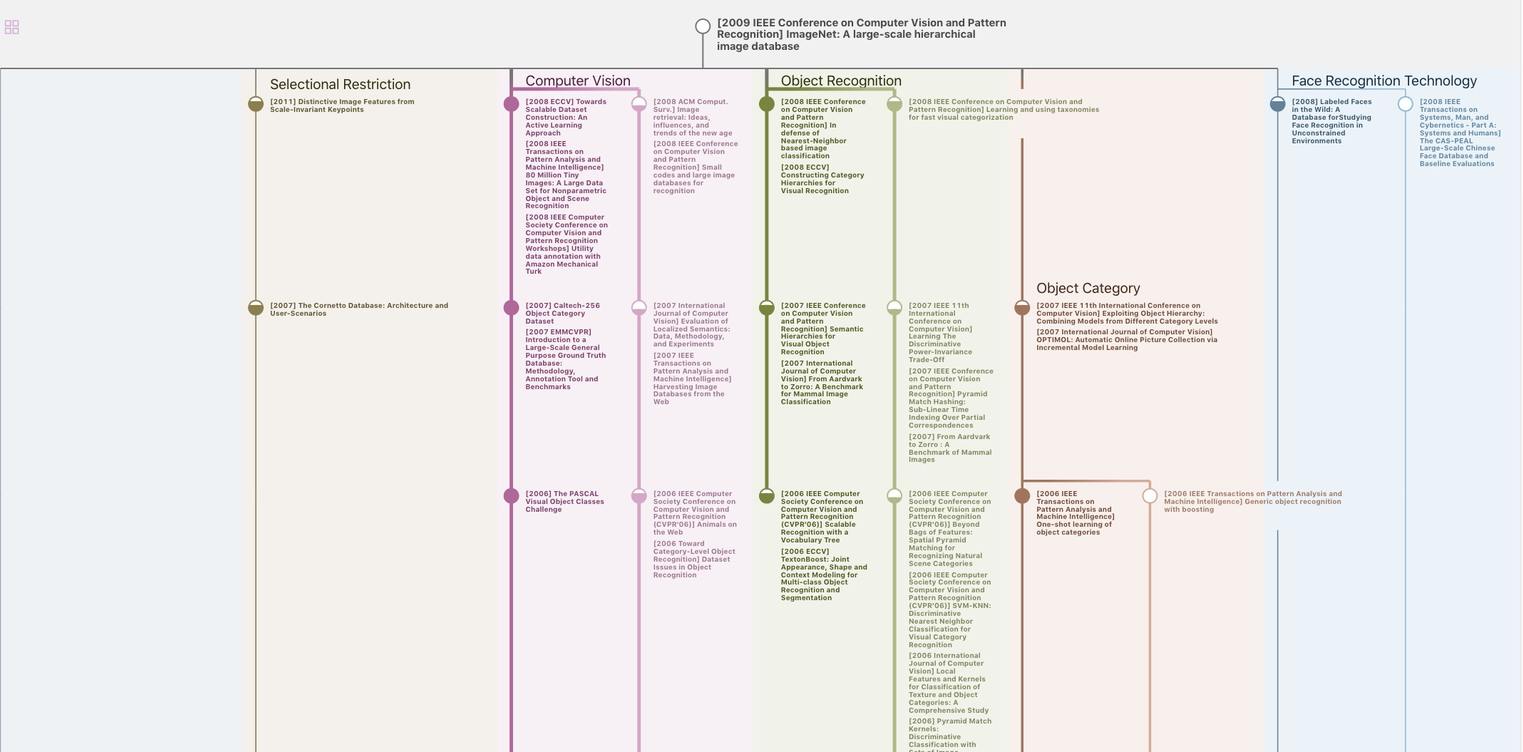
生成溯源树,研究论文发展脉络
Chat Paper
正在生成论文摘要