Parallelizable sparse inverse formulation Gaussian processes (SpInGP)
2017 IEEE 27TH INTERNATIONAL WORKSHOP ON MACHINE LEARNING FOR SIGNAL PROCESSING(2017)
摘要
We propose a parallelizable sparse inverse formulation Gaussian process (SpInGP) for temporal models. It uses a sparse precision GP formulation and sparse matrix routines to speed up the computations. Due to the state-space formulation used in the algorithm, the time complexity of the basic SpInGP is linear, and because all the computations are parallelizable, the parallel form of the algorithm is sublinear in the number of data points. We provide example algorithms to implement the sparse matrix routines and experimentally test the method using both simulated and real data.
更多查看译文
关键词
sparse matrix routines,parallelizable sparse inverse formulation Gaussian processes,SpInGP,parallelizable sparse inverse formulation Gaussian process,sparse precision GP formulation
AI 理解论文
溯源树
样例
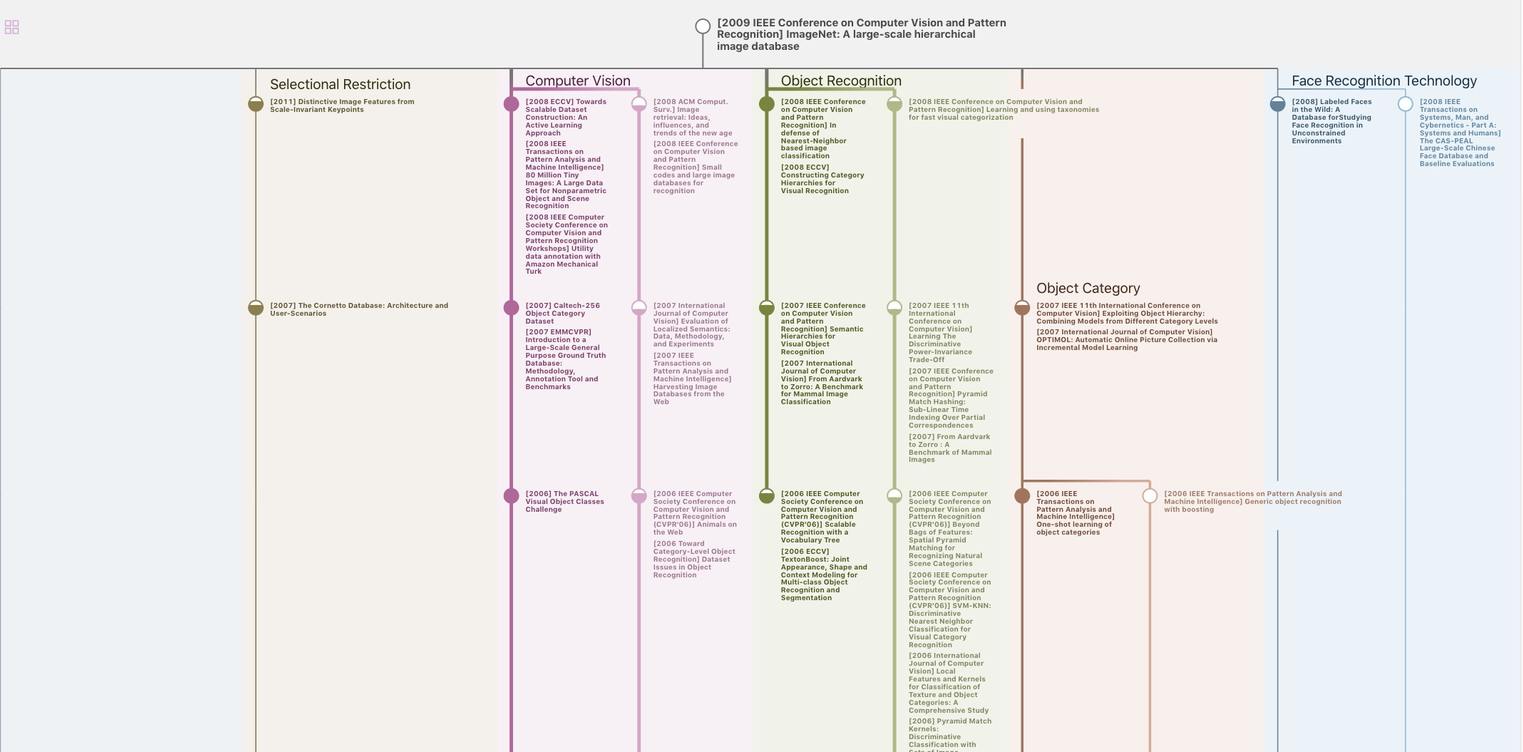
生成溯源树,研究论文发展脉络
Chat Paper
正在生成论文摘要