Multimodal Affect Recognition In The Context Of Human-Computer Interaction For Companion-Systems
COMPANION TECHNOLOGY: A PARADIGM SHIFT IN HUMAN-TECHNOLOGY INTERACTION(2017)
摘要
In general, humans interact with each other using multiple modalities. The main channels are speech, facial expressions, and gesture. But also bio-physiological data such as biopotentials can convey valuable information which can be used to interpret the communication in a dedicated way. A Companion-System can use these modalities to performan efficient human-computer interaction (HCI). To do so, the multiple sources need to be analyzed and combined in technical systems. However, so far only few studies have been published dealing with the fusion of three or even more such modalities. This chapter addresses the necessary processing steps in the development of a multimodal system applying fusion approaches.ATLAS and ikannotate are presented which are designed for the pre-analyzing of multimodal data streams and the labeling of relevant parts. ATLAS allows us to display raw data, extracted features and even outputs of pre-trained classifier modules. Further, the tool integrates annotation, transcription and an active learning module. Ikannotate can be directly used for transcription and guided step-wise emotional annotation of multimodal data. The tool includes the three mainly used annotation paradigms, namely the basic emotions, the Geneva emotion wheel and the self-assessment manikins (SAMs). Furthermore, annotators using ikannotate can assign an uncertainty to samples.Classifier architectures need to realize a fusion system in which the multiple modalities are combined. A large number of machine learning approaches were evaluated, such as data, feature, score and decision-level fusion schemes, but also temporal fusion architectures and partially supervised learning.The proposed methods are evaluated on either multimodal benchmark corpora or on the datasets of the Transregional Collaborative Research Centre SFB/TRR 62, i.e. Last Minute Corpus and the EmoRec Dataset. Furthermore, we present results which were achieved in international challenges.
更多查看译文
AI 理解论文
溯源树
样例
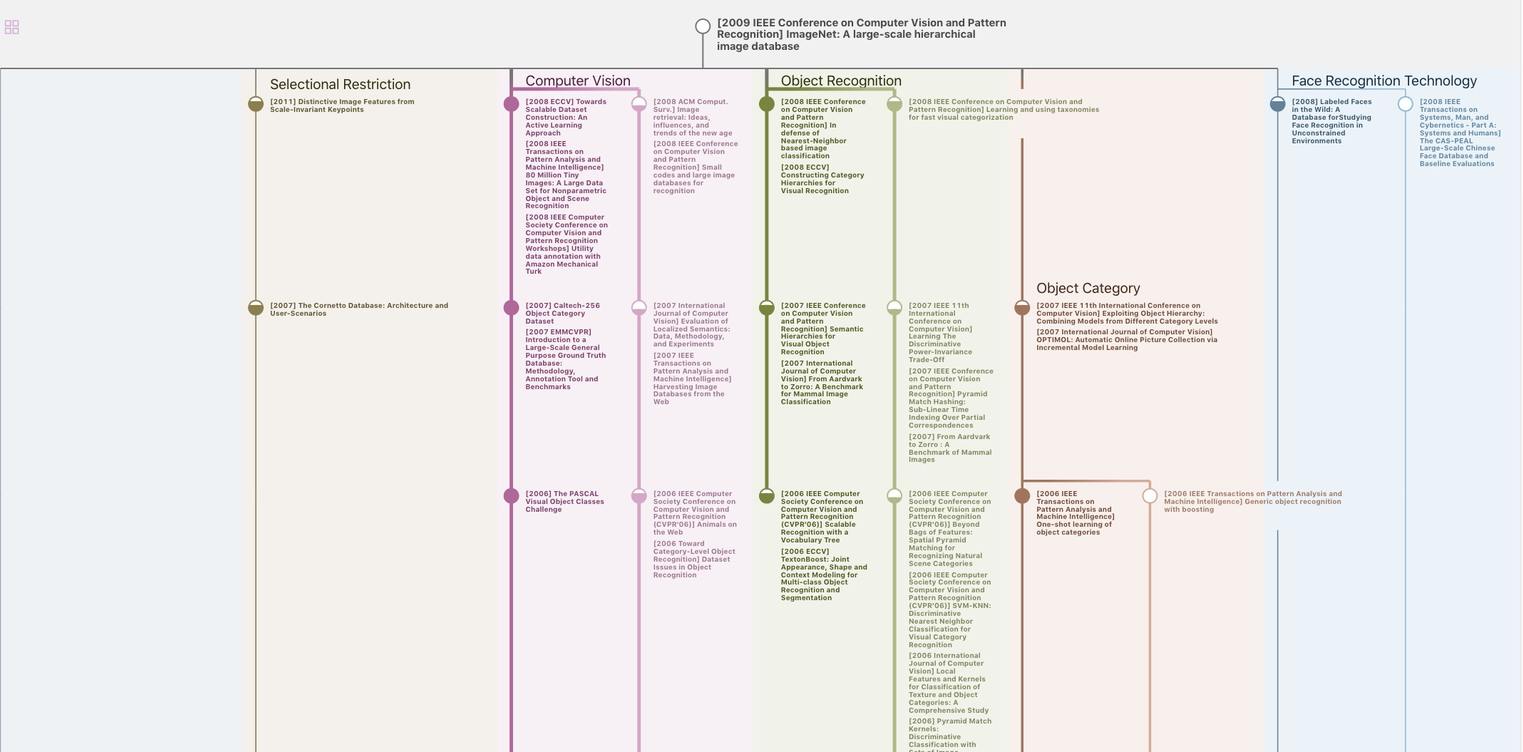
生成溯源树,研究论文发展脉络
Chat Paper
正在生成论文摘要