Cell-specific prediction and application of drug-induced gene expression profiles.
Biocomputing-Pacific Symposium on Biocomputing(2018)
摘要
Gene expression profiling of in vitro drug perturbations is useful for many biomedical discovery applications including drug repurposing and elucidation of drug mechanisms. However, limited data availability across cell types has hindered our capacity to leverage or explore the cellspecificity of these perturbations. While recent efforts have generated a large number of drug perturbation profiles across a variety of human cell types, many gaps remain in this combinatorial drug-cell space. Hence, we asked whether it is possible to fill these gaps by predicting cell-specific drug perturbation profiles using available expression data from related conditions--i.e. from other drugs and cell types. We developed a computational framework that first arranges existing profiles into a three-dimensional array (or tensor) indexed by drugs, genes, and cell types, and then uses either local (nearest-neighbors) or global (tensor completion) information to predict unmeasured profiles. We evaluate prediction accuracy using a variety of metrics, and find that the two methods have complementary performance, each superior in different regions in the drug-cell space. Predictions achieve correlations of 0.68 with true values, and maintain accurate differentially expressed genes (AUC 0.81). Finally, we demonstrate that the predicted profiles add value for making downstream associations with drug targets and therapeutic classes.
更多查看译文
关键词
Drug discovery,chemogenomics,tensor completion,gene expression,drug repurposing
AI 理解论文
溯源树
样例
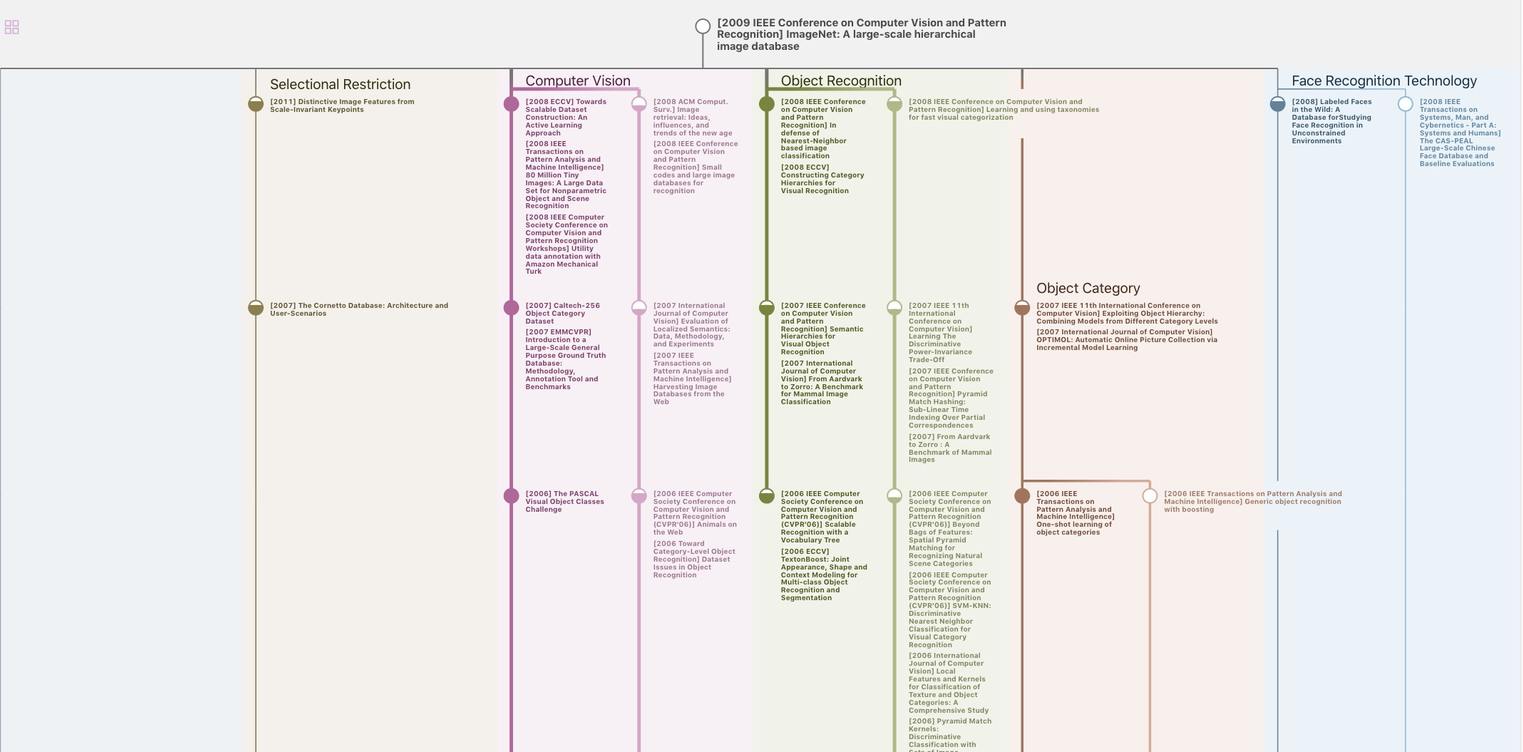
生成溯源树,研究论文发展脉络
Chat Paper
正在生成论文摘要