Improving precision in concept normalization.
Biocomputing-Pacific Symposium on Biocomputing(2018)
摘要
Most natural language processing applications exhibit a trade-off between precision and recall. In some use cases for natural language processing, there are reasons to prefer to tilt that trade-off toward high precision. Relying on the Zipfian distribution of false positive results, we describe a strategy for increasing precision, using a variety of both pre-processing and post-processing methods. They draw on both knowledge-based and frequentist approaches to modeling language. Based on an existing high-performance biomedical concept recognition pipeline and a previously published manually annotated corpus, we apply this hybrid rationalist/empiricist strategy to concept normalization for eight different ontologies. Which approaches did and did not improve precision varied widely between the ontologies.
更多查看译文
关键词
ontologies,precision medicine,natural language processing,text mining,concept normalization
AI 理解论文
溯源树
样例
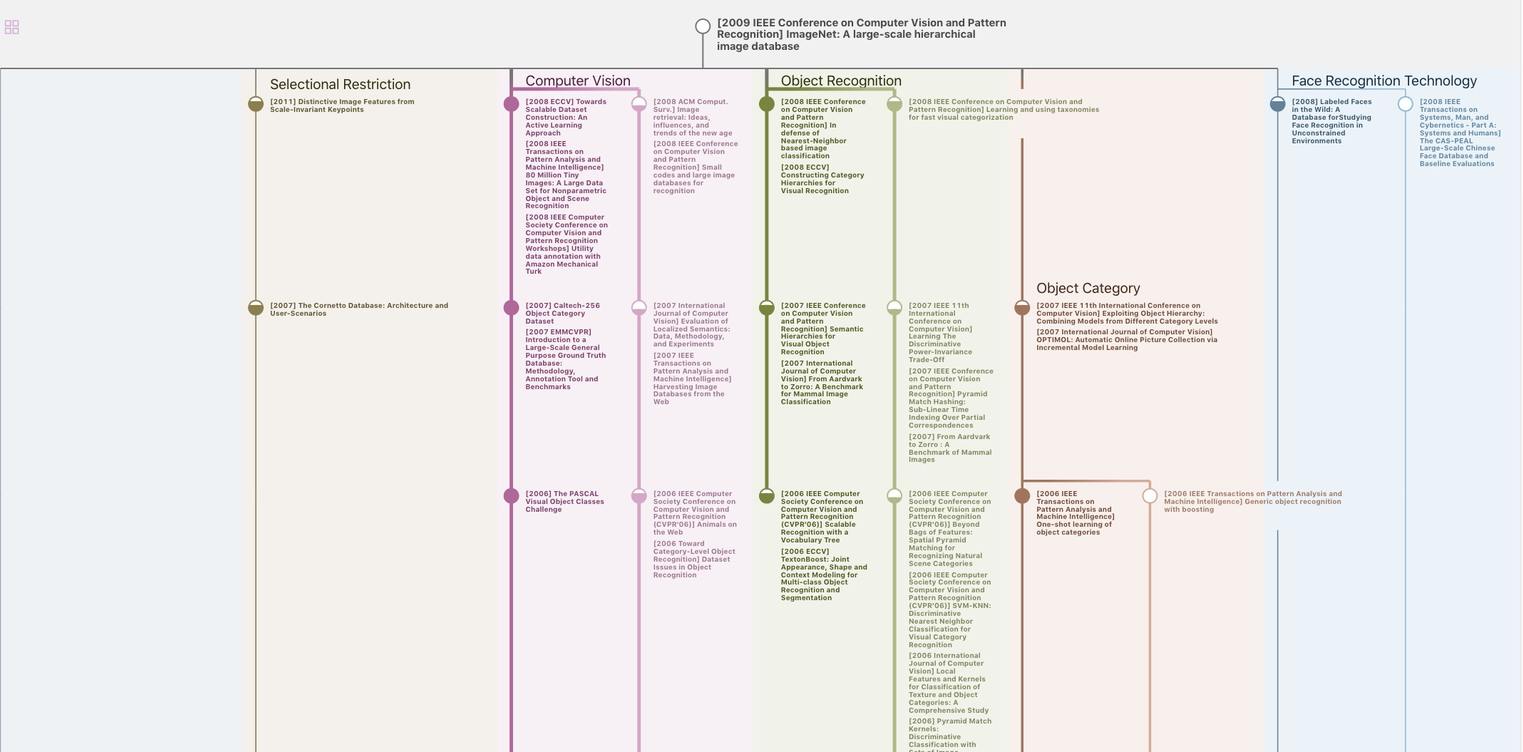
生成溯源树,研究论文发展脉络
Chat Paper
正在生成论文摘要