A data-driven perspective on the hierarchical assembly of molecular structures.
JOURNAL OF CHEMICAL THEORY AND COMPUTATION(2018)
摘要
Macromolecular systems are composed of a very large number of atomic degrees of freedom. There is strong evidence suggesting that structural changes occurring in large biomolecular systems at long time scale dynamics may be captured by models coarser than atomistic, although a suitable or optimal coarse-graining is a priori unknown. Here we propose a systematic approach to learning a coarse representation of a macromolecule from microscopic simulation data. In particular, the definition of effective coarse variables is achieved by partitioning the degrees of freedom both in the structural (physical) space and in the conformational space. The identification of groups of microscopic particles forming dynamical coherent states in different metastable states leads to a multiscale description of the system, in space and time. The application of this approach to the folding dynamics of two proteins provides a revised view of the classical idea of prestructured regions (foldons) that combine during a protein-folding process and suggests a hierarchical characterization of the assembly process of folded structures.
更多查看译文
关键词
molecular structures,hierarchical assembly,data-driven
AI 理解论文
溯源树
样例
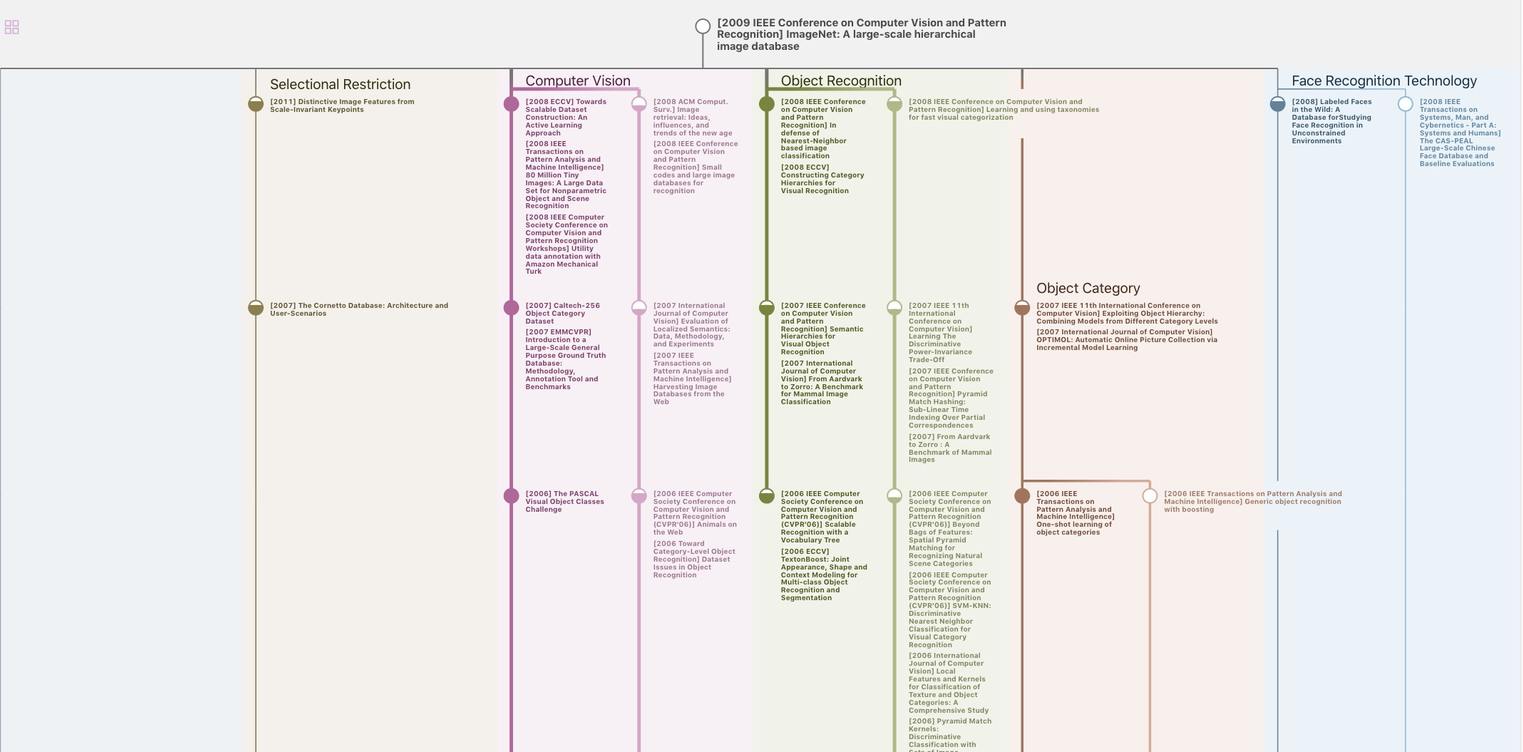
生成溯源树,研究论文发展脉络
Chat Paper
正在生成论文摘要