A mixed integer programming-based global optimization framework for analyzing gene expression data
J. Global Optimization(2017)
摘要
The analysis of high throughput gene expression patients/controls experiments is based on the determination of differentially expressed genes according to standard statistical tests. A typical bioinformatics approach to this problem is composed of two separate steps: first, a subset of genes with altered expression level is identified; then the pathways which are statistically enriched by those genes are selected, assuming they play a relevant role for the biological condition under study. Often, the set of selected pathways contains elements that are not related to the condition. This is due to the fact that the statistical significance is not sufficient for biological relevance. To overcome these problems, we propose a method based on a large mixed integer program that implements a new feature selection model to simultaneously identify the genes whose over- and under-expressions, combined together, discriminate different cancer subtypes, as well as the pathways that are enriched by these genes. The innovation in this model is the solutions are driven towards the enrichment of pathways. That may indeed introduce a bias in the search; such a bias is counter-balanced by a wide exploration of the solution space, varying the involved parameters in their feasible region, and then using a global optimization approach. The conjoint analysis of the pool of solutions obtained by this exploration should indeed provide a robust final set of genes and pathways, overcoming the potential drawbacks of relying solely on statistical significance. Experimental results on transcriptomes for different types of cancer from the Cancer Genome Atlas are presented. The method is able to identify crisp relations between the considered subtypes of cancer and few selected pathways, eventually validated by the biological analysis.
更多查看译文
关键词
Gene expression,Pathways,Statistical significance,Global optimization,MIP,Feature selection
AI 理解论文
溯源树
样例
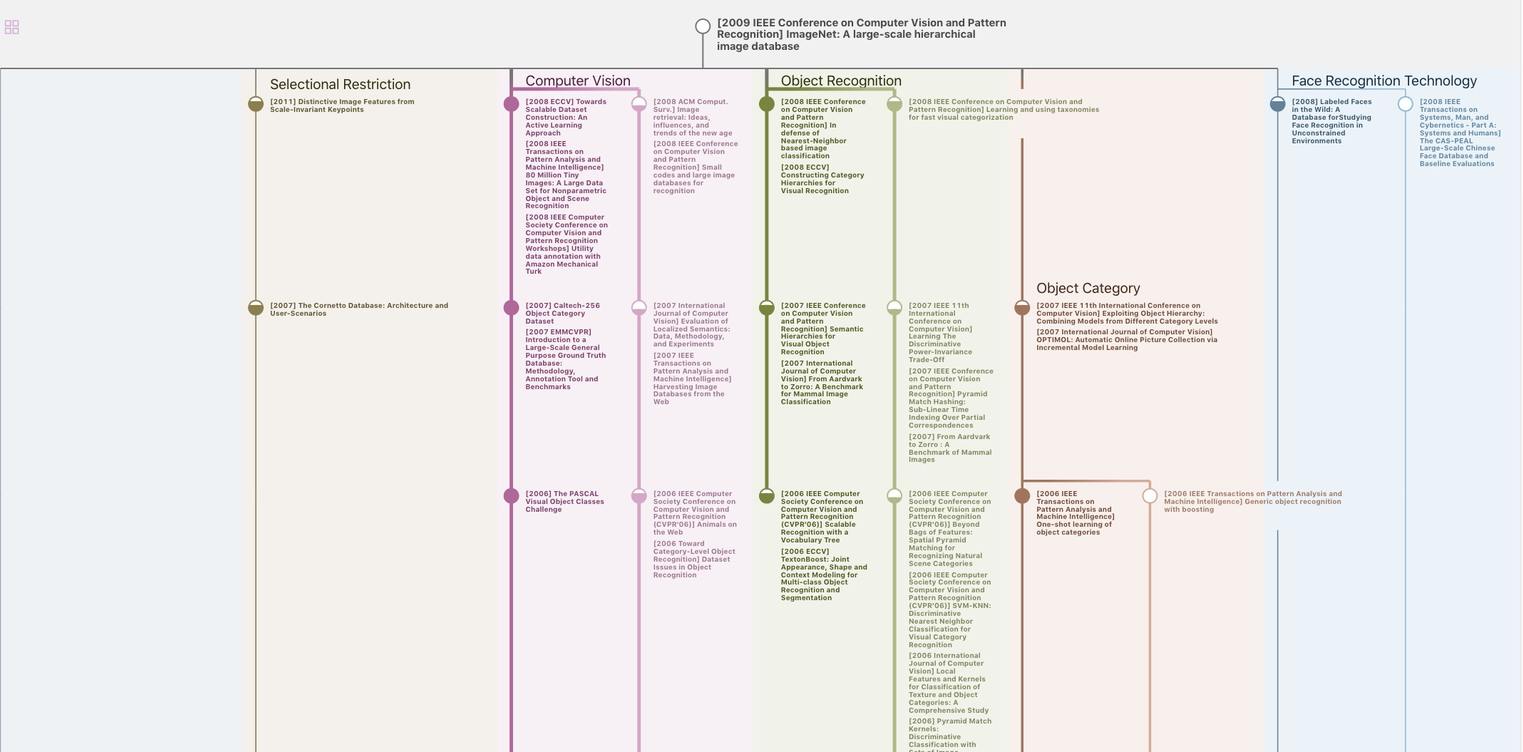
生成溯源树,研究论文发展脉络
Chat Paper
正在生成论文摘要