On the Suboptimality of Proximal Gradient Descent for ℓ^0 Sparse Approximation
CoRR(2017)
摘要
We study the proximal gradient descent (PGD) method for ℓ^0 sparse approximation problem as well as its accelerated optimization with randomized algorithms in this paper. We first offer theoretical analysis of PGD showing the bounded gap between the sub-optimal solution by PGD and the globally optimal solution for the ℓ^0 sparse approximation problem under conditions weaker than Restricted Isometry Property widely used in compressive sensing literature. Moreover, we propose randomized algorithms to accelerate the optimization by PGD using randomized low rank matrix approximation (PGD-RMA) and randomized dimension reduction (PGD-RDR). Our randomized algorithms substantially reduces the computation cost of the original PGD for the ℓ^0 sparse approximation problem, and the resultant sub-optimal solution still enjoys provable suboptimality, namely, the sub-optimal solution to the reduced problem still has bounded gap to the globally optimal solution to the original problem.
更多查看译文
AI 理解论文
溯源树
样例
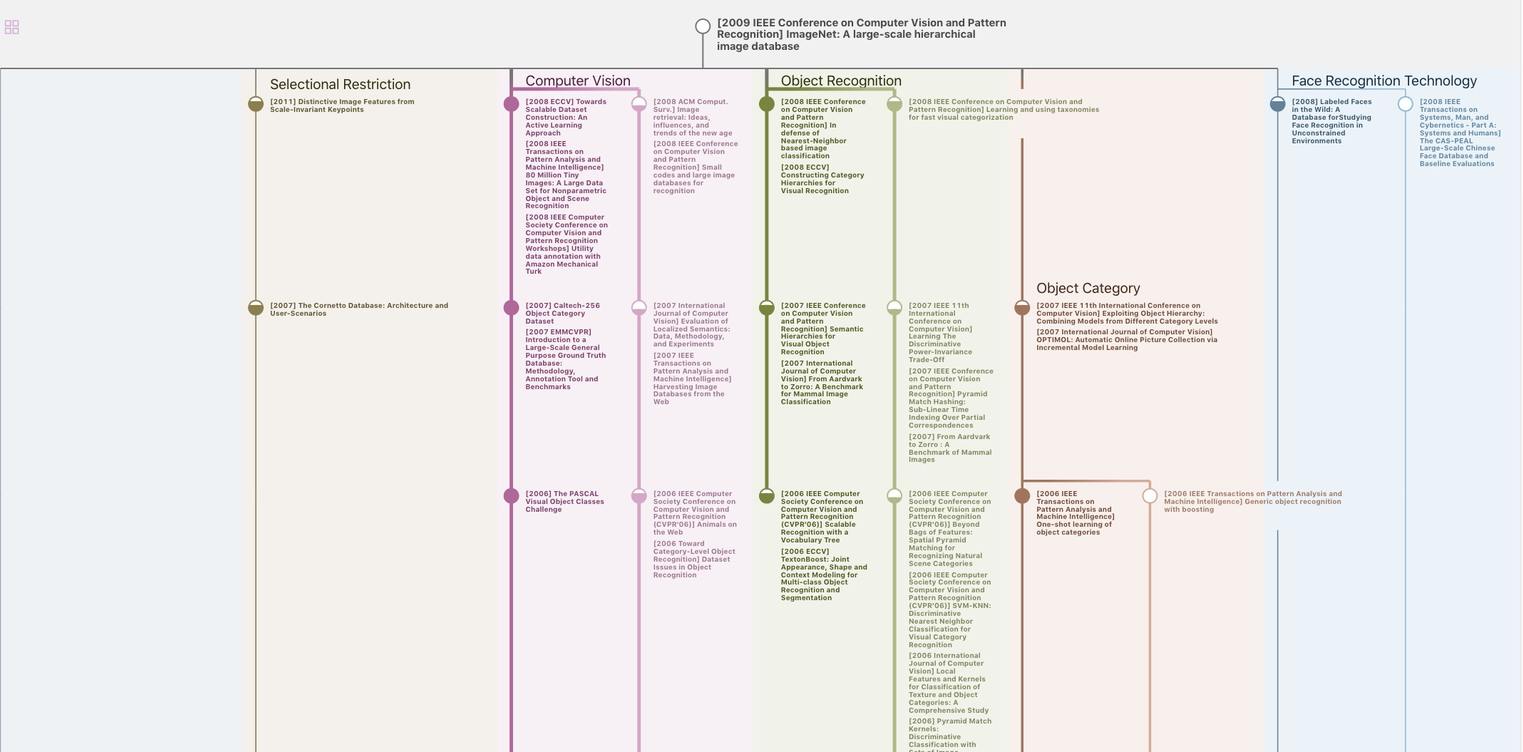
生成溯源树,研究论文发展脉络
Chat Paper
正在生成论文摘要