Deep Object-Centric Representations for Generalizable Robot Learning
2018 IEEE International Conference on Robotics and Automation (ICRA)(2017)
摘要
Robotic manipulation in complex open-world scenarios requires both reliable physical manipulation skills and effective and generalizable perception. In this paper, we propose a method where general purpose pretrained visual models serve as an object-centric prior for the perception system of a learned policy. We devise an object-level attentional mechanism that can be used to determine relevant objects from a few trajectories or demonstrations, and then immediately incorporate those objects into a learned policy. A task-independent meta-attention locates possible objects in the scene, and a task-specific attention identifies which objects are predictive of the trajectories. The scope of the task-specific attention is easily adjusted by showing demonstrations with distractor objects or with diverse relevant objects. Our results indicate that this approach exhibits good generalization across object instances using very few samples, and can be used to learn a variety of manipulation tasks using reinforcement learning.
更多查看译文
关键词
robotic manipulation,generalizable robot learning,object-centric representations,reinforcement learning,object-level attentional mechanism,perception system,semantic feature space
AI 理解论文
溯源树
样例
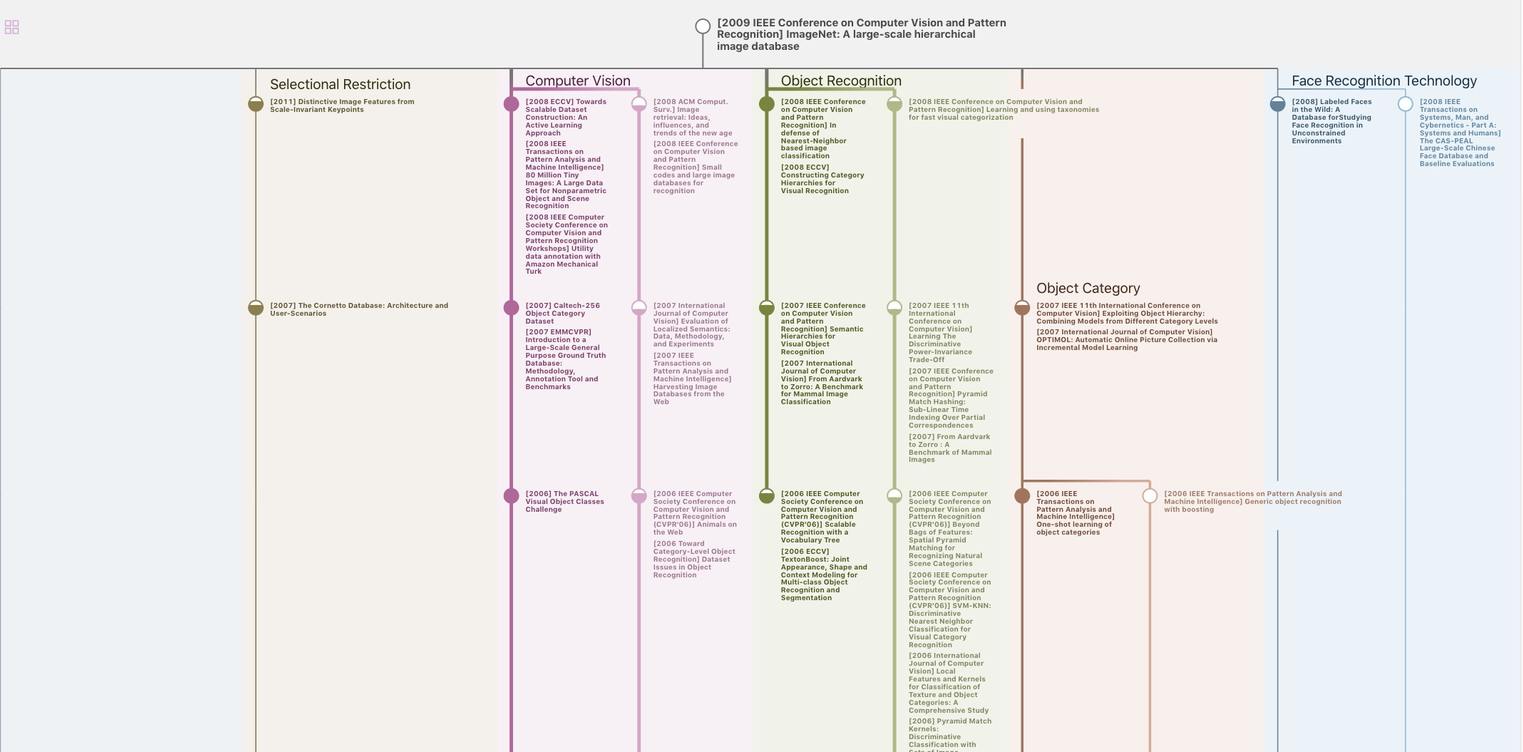
生成溯源树,研究论文发展脉络
Chat Paper
正在生成论文摘要