SeeThrough: Finding Chairs in Heavily Occluded Indoor Scenes.
arXiv: Computer Vision and Pattern Recognition(2017)
摘要
3D geometry mockups of single images of indoor scenes are useful for many applications including interior design, content creation for virtual reality, and image manipulation. Unfortunately, manually modeling a scene from a single image is tedious and requires expert knowledge. We aim to construct scene mockups from single images automatically. However, automatically inferring 3D scenes from 2D images is an ill-posed problem, as 2D images are projections of many meaningful attributes (e.g., geometry, illumination, material), with objects regularly being occluded by themselves or others. Recent systems targeting scene mockups mirror this observation and work mostly on fully visible objects. To improve the state-of-the-art we need to take into account contextual information, exploiting the fact that objects co-occur mostly in typical regular configurations. We exploit such regularity in the form of data-driven scene priors. Specifically, we first use a neural network trained on scene arrangement to extract keypoints, then feed these keypoints to a candidate object generation stage. From these candidates, an optimal selection is then made using pairwise co-occurrence statistics, discovered from a database of 3D scenes. We iterate the process allowing for candidates with low keypoint response to be detected based on the location of neighbouring objects. We demonstrate that our pipeline outperforms combinations of state-of-the-art single object methods, especially for scenes with severely occluded objects. All code, training data, and scene statistics will be available for research use.
更多查看译文
AI 理解论文
溯源树
样例
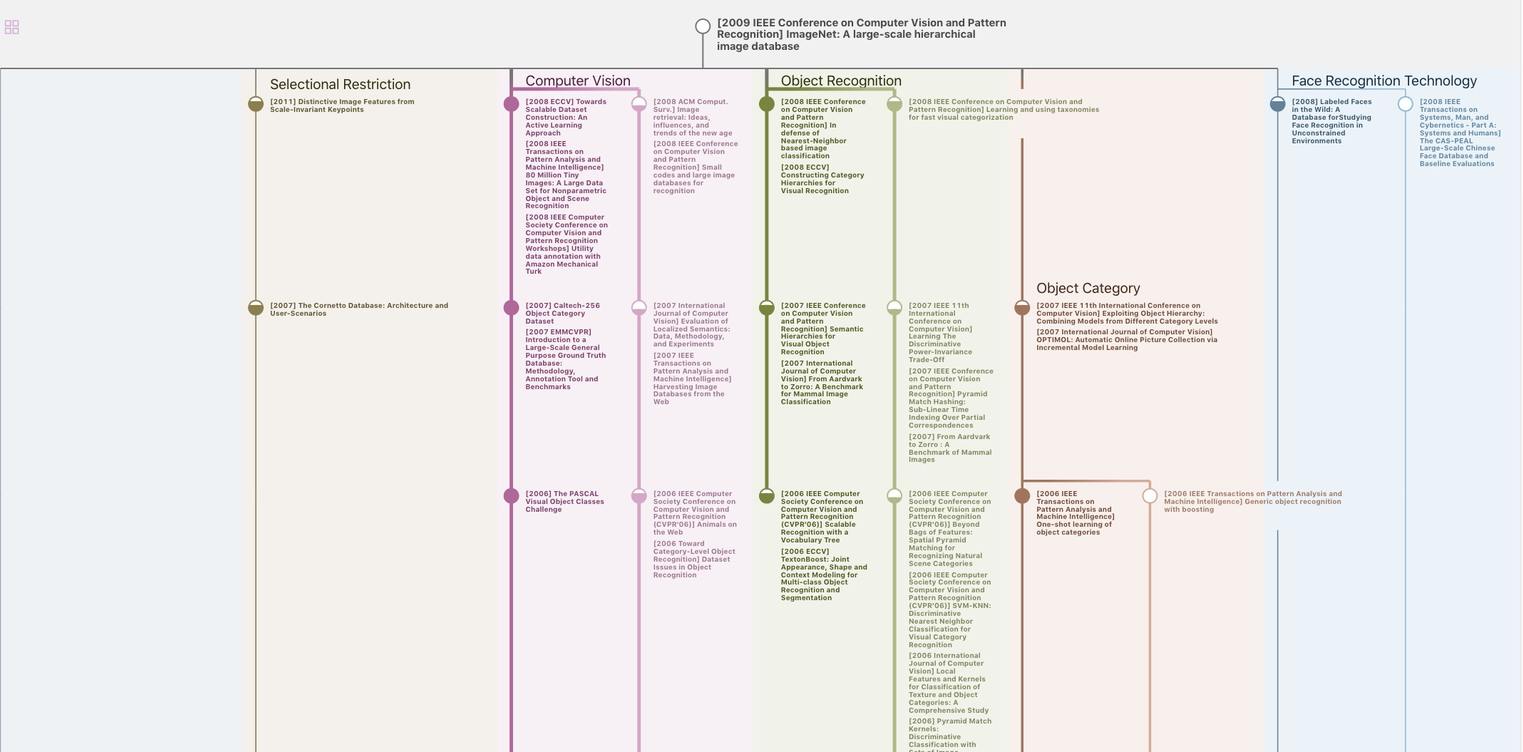
生成溯源树,研究论文发展脉络
Chat Paper
正在生成论文摘要