A New Data Source for Inverse Dynamics Learning
2017 IEEE/RSJ INTERNATIONAL CONFERENCE ON INTELLIGENT ROBOTS AND SYSTEMS (IROS)(2017)
摘要
Modern robotics is gravitating toward increasingly collaborative human robot interaction. Tools such as acceleration policies can naturally support the realization of reactive, adaptive, and compliant robots. These tools require us to model the system dynamics accurately -- a difficult task. The fundamental problem remains that simulation and reality diverge--we do not know how to accurately change a robot's state. Thus, recent research on improving inverse dynamics models has been focused on making use of machine learning techniques. Traditional learning techniques train on the actual realized accelerations, instead of the policy's desired accelerations, which is an indirect data source. Here we show how an additional training signal -- measured at the desired accelerations -- can be derived from a feedback control signal. This effectively creates a second data source for learning inverse dynamics models. Furthermore, we show how both the traditional and this new data source, can be used to train task-specific models of the inverse dynamics, when used independently or combined. We analyze the use of both data sources in simulation and demonstrate its effectiveness on a real-world robotic platform. We show that our system incrementally improves the learned inverse dynamics model, and when using both data sources combined converges more consistently and faster.
更多查看译文
关键词
modern robotics,acceleration policies,compliant robots,system dynamics,inverse dynamics models,machine learning techniques,actual realized accelerations,desired accelerations,indirect data source,additional training signal,task-specific models,real-world robotic platform,learned inverse dynamics model,collaborative human robot interaction,traditional learning techniques,feedback control signal
AI 理解论文
溯源树
样例
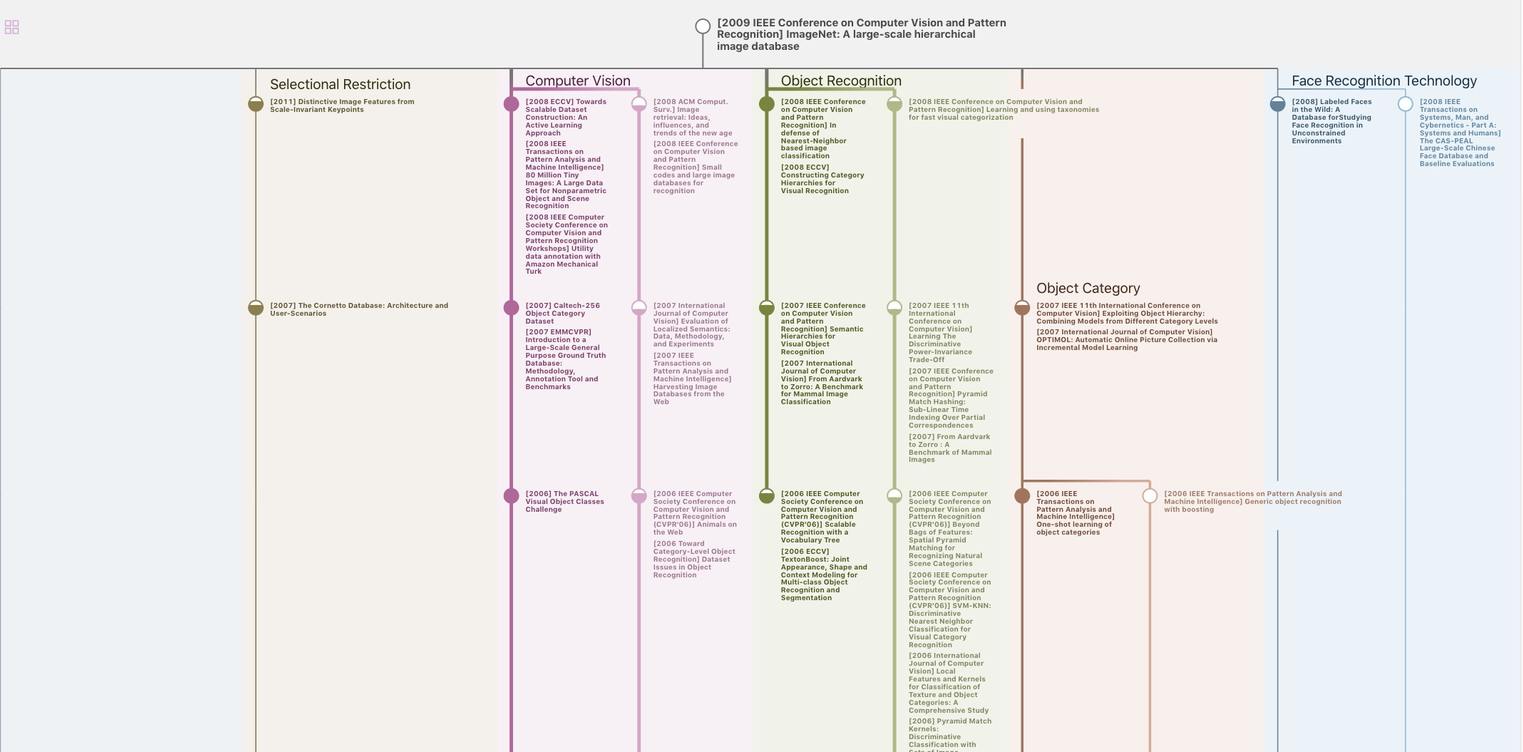
生成溯源树,研究论文发展脉络
Chat Paper
正在生成论文摘要