Large-Scale Domain Adaptation Via Teacher-Student Learning
18TH ANNUAL CONFERENCE OF THE INTERNATIONAL SPEECH COMMUNICATION ASSOCIATION (INTERSPEECH 2017), VOLS 1-6: SITUATED INTERACTION(2017)
摘要
High accuracy speech recognition requires a large amount of transcribed data for supervised training. In the absence of such data, domain adaptation of a well-trained acoustic model can be performed, but even here, high accuracy usually requires significant labeled data from the target domain. In this work, we propose an approach to domain adaptation that does not require transcriptions but instead uses a corpus of unlabeled parallel data, consisting of pairs of samples from the source domain of the well-trained model and the desired target domain. To perform adaptation, we employ teacher/student (T/S) learning, in which the posterior probabilities generated by the source-domain model can be used in lieu of labels to train the target-domain model. We evaluate the proposed approach in two scenarios, adapting a clean acoustic model to noisy speech and adapting an adults' speech acoustic model to children's speech. Significant improvements in accuracy are obtained, with reductions in word error rate of up to 44% over the original source model without the need for transcribed data in the target domain. Moreover, we show that increasing the amount of unlabeled data results in additional model robustness. which is particularly beneficial when using simulated training data in the target-domain.
更多查看译文
关键词
teacher-student learning, parallel unlabeled data
AI 理解论文
溯源树
样例
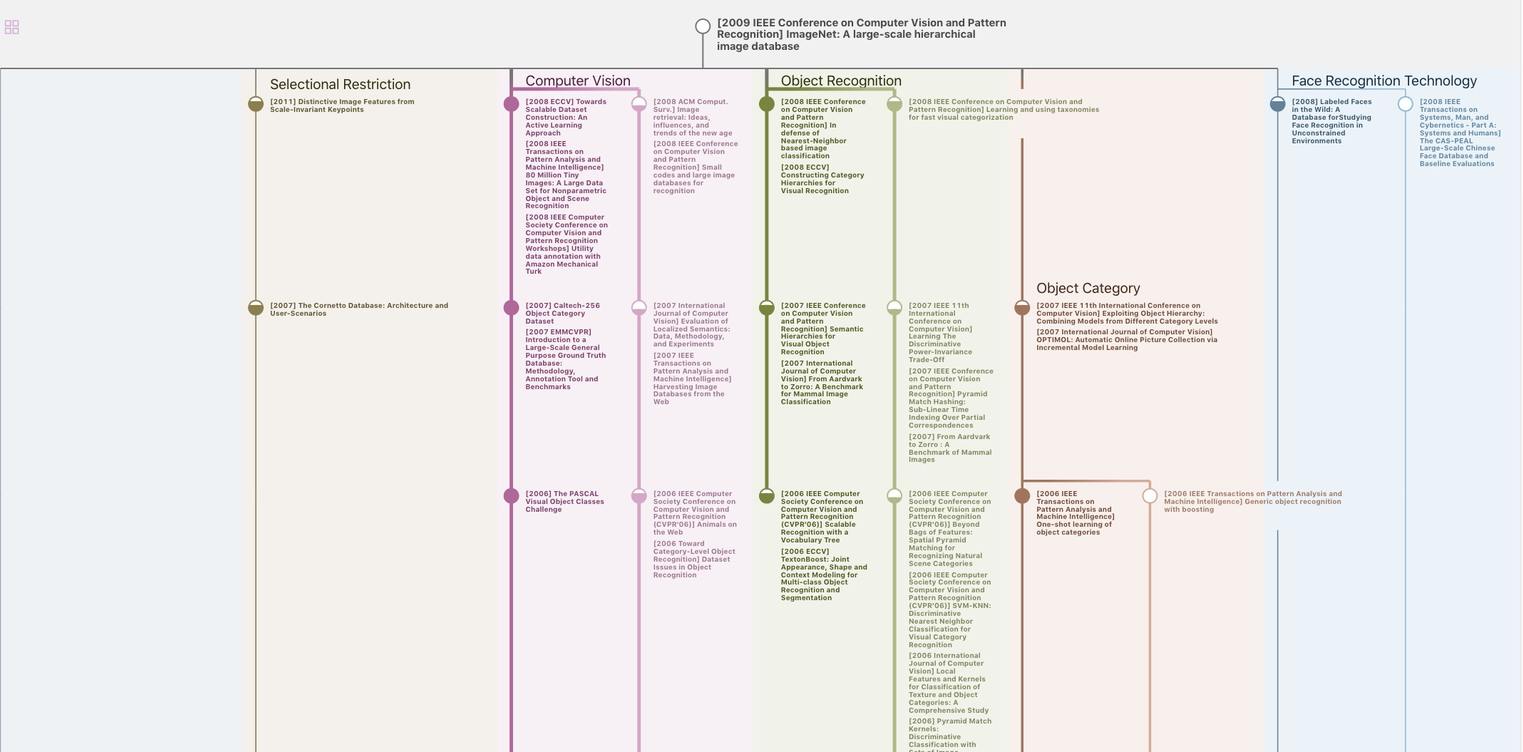
生成溯源树,研究论文发展脉络
Chat Paper
正在生成论文摘要