Medical Image Segmentation Based on Multi-Modal Convolutional Neural Network: Study on Image Fusion Schemes
2018 IEEE 15th International Symposium on Biomedical Imaging (ISBI 2018)(2017)
摘要
Image analysis using more than one modality (i.e. multi-modal) has been increasingly applied in the field of biomedical imaging. One of the challenges in performing the multimodal analysis is that there exist multiple schemes for fusing the information from different modalities, where such schemes are application-dependent and lack a unified framework to guide their designs. In this work we firstly propose a conceptual architecture for the image fusion schemes in supervised biomedical image analysis: fusing at the feature level, fusing at the classifier level, and fusing at the decision-making level. Further, motivated by the recent success in applying deep learning for natural image analysis, we implement the three image fusion schemes above based on the Convolutional Neural Network (CNN) with varied structures, and combined into a single framework. The proposed image segmentation framework is capable of analyzing the multi-modality images using different fusing schemes simultaneously. The framework is applied to detect the presence of soft tissue sarcoma from the combination of Magnetic Resonance Imaging (MRI), Computed Tomography (CT) and Positron Emission Tomography (PET) images. It is found from the results that while all the fusion schemes outperform the single-modality schemes, fusing at the feature level can generally achieve the best performance in terms of both accuracy and computational cost, but also suffers from the decreased robustness in the presence of large errors in any image modalities.
更多查看译文
关键词
multi-modal imaging analysis,deep learning,image fusion
AI 理解论文
溯源树
样例
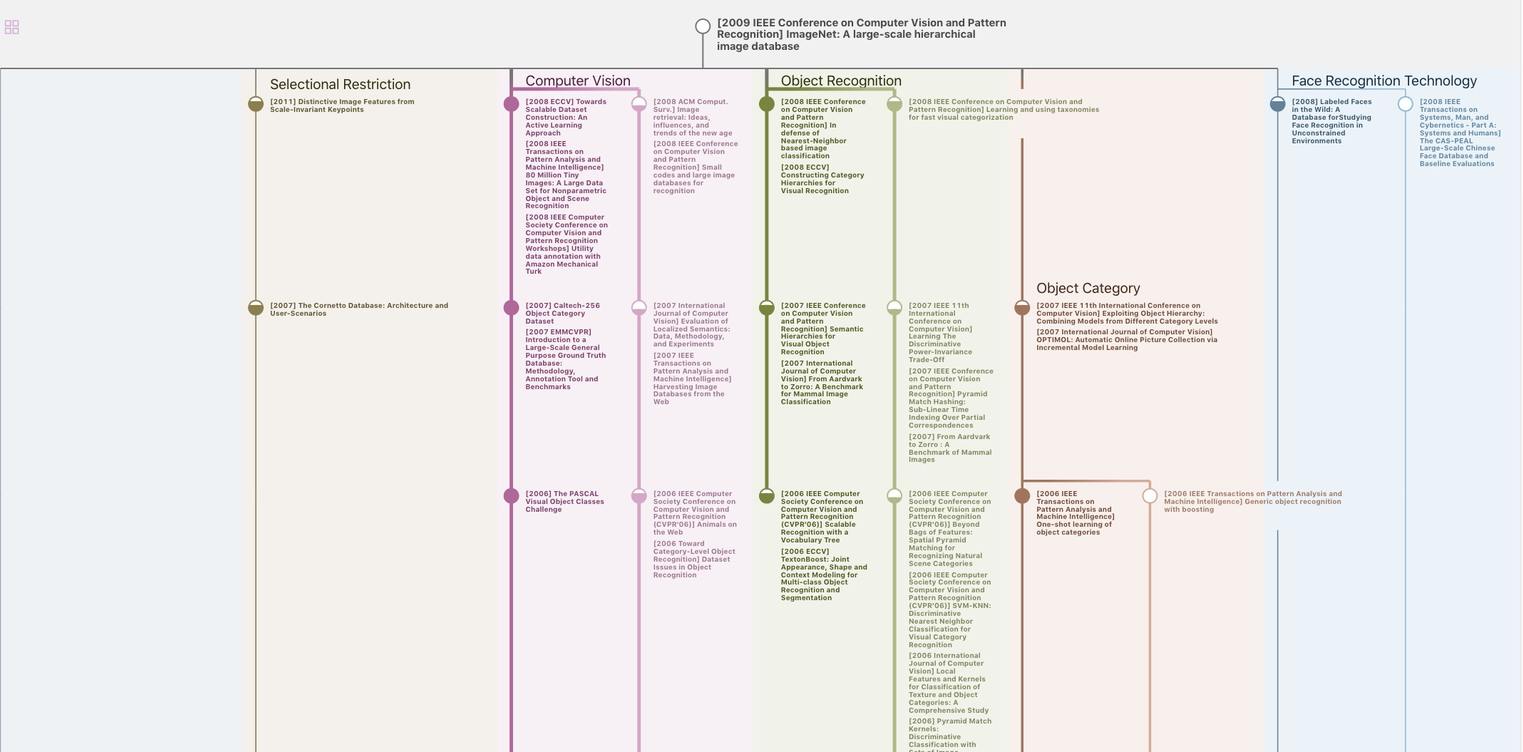
生成溯源树,研究论文发展脉络
Chat Paper
正在生成论文摘要