Context Embedding Networks
2018 IEEE/CVF Conference on Computer Vision and Pattern Recognition(2018)
摘要
Low dimensional embeddings that capture the main variations of interest in collections of data are important for many applications. One way to construct these embeddings is to acquire estimates of similarity from the crowd. However, similarity is a multi-dimensional concept that varies from individual to individual. Existing models for learning embeddings from the crowd typically make simplifying assumptions such as all individuals estimate similarity using the same criteria, the list of criteria is known in advance, or that the crowd workers are not influenced by the data that they see. To overcome these limitations we introduce Context Embedding Networks (CENs). In addition to learning interpretable embeddings from images, CENs also model worker biases for different attributes along with the visual context i.e. the visual attributes highlighted by a set of images. Experiments on two noisy crowd annotated datasets show that modeling both worker bias and visual context results in more interpretable embeddings compared to existing approaches.
更多查看译文
关键词
low dimensional embeddings,crowd workers,interpretable embeddings,model worker biases,noisy crowd,visual context,context embedding networks,CENs,data collections,crowd similarity,multi-dimensional concept,crowd embedding learning,image set
AI 理解论文
溯源树
样例
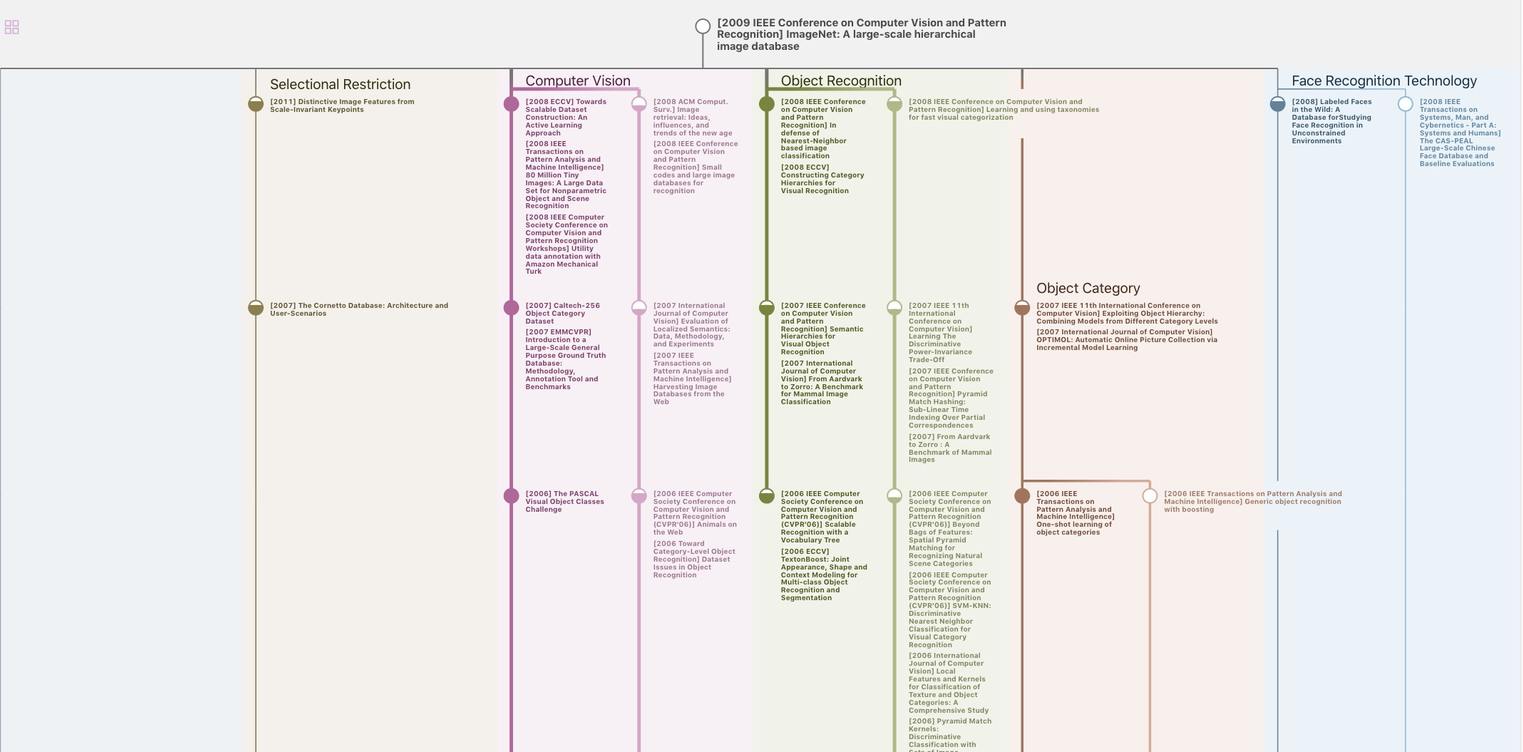
生成溯源树,研究论文发展脉络
Chat Paper
正在生成论文摘要