Building Data-driven Models with Microstructural Images: Generalization and Interpretability.
Materials Discovery(2017)
摘要
As data-driven methods rise in popularity in materials science applications, a key question is how these machine learning models can be used to understand microstructure. Given the importance of process–structure–property relations throughout materials science, it seems logical that models that can leverage microstructural data would be more capable of predicting property information. While there have been some recent attempts to use convolutional neural networks to understand microstructural images, these early studies have focused only on which featurizations yield the highest machine learning model accuracy for a single data set. This paper explores the use of convolutional neural networks for classifying microstructure with a more holistic set of objectives in mind: generalization between data sets, number of features required, and interpretability.
更多查看译文
关键词
Machine learning,Microstructure,Neural network
AI 理解论文
溯源树
样例
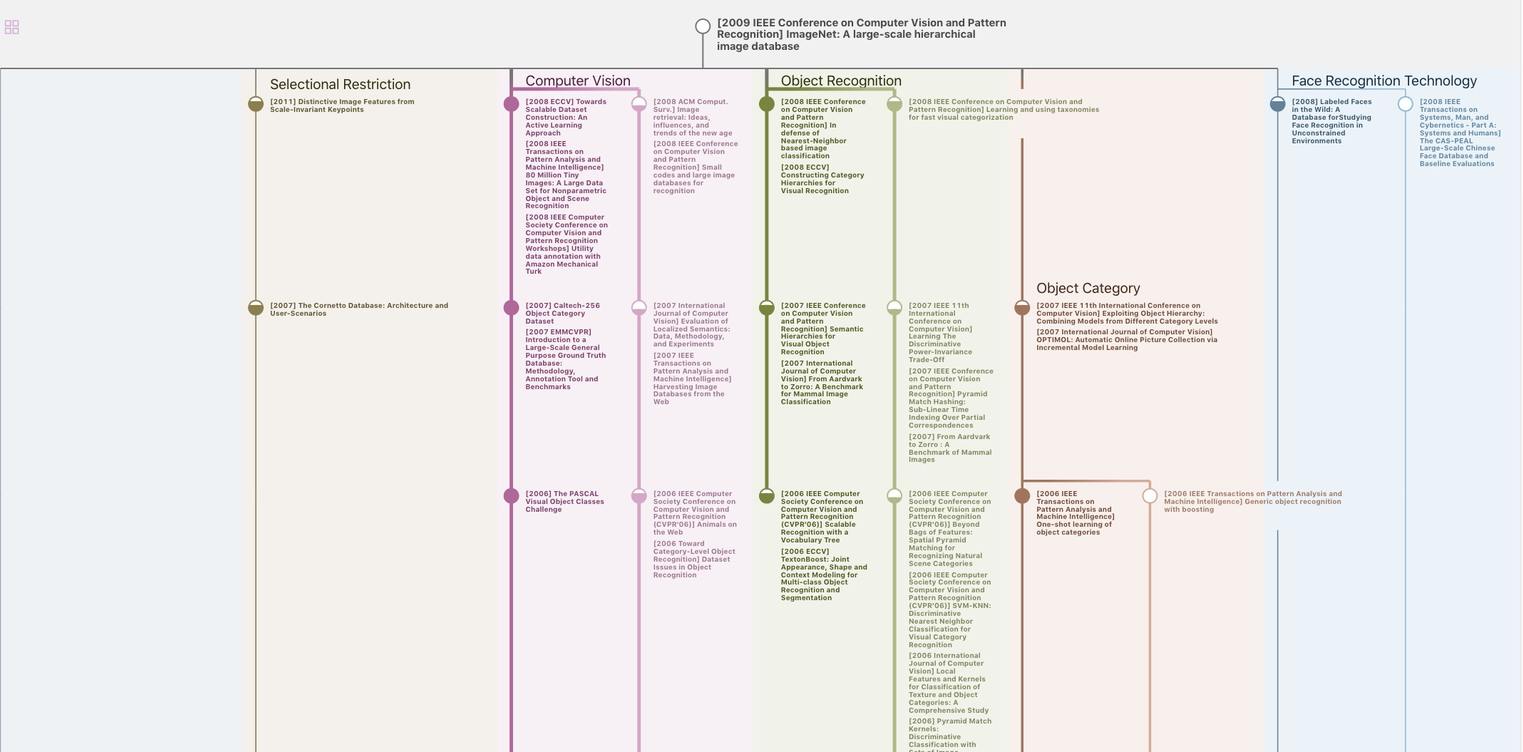
生成溯源树,研究论文发展脉络
Chat Paper
正在生成论文摘要