LOOP Descriptor: Encoding Repeated Local Patterns for Fine-grained Visual Identification of Lepidoptera.
arXiv: Computer Vision and Pattern Recognition(2017)
摘要
This letter introduces the LOOP binary descriptor (local optimal oriented pattern) that encodes rotation invariance into the main formulation itself. This makes any post processing stage for rotation invariance redundant and improves on both accuracy and time complexity. We consider fine-grained lepidoptera (moth/butterfly) species recognition as the representative problem since it involves repetition of localized patterns and textures that may be exploited for discrimination. We evaluate the performance of LOOP against its predecessors as well as few other popular descriptors. Besides experiments on standard benchmarks, we also introduce a new small image dataset on NZ Lepidoptera. Loop performs as well or better on all datasets evaluated compared to previous binary descriptors. The new dataset and demo code of the proposed method are available through the lead authoru0027s academic webpage and GitHub.
更多查看译文
AI 理解论文
溯源树
样例
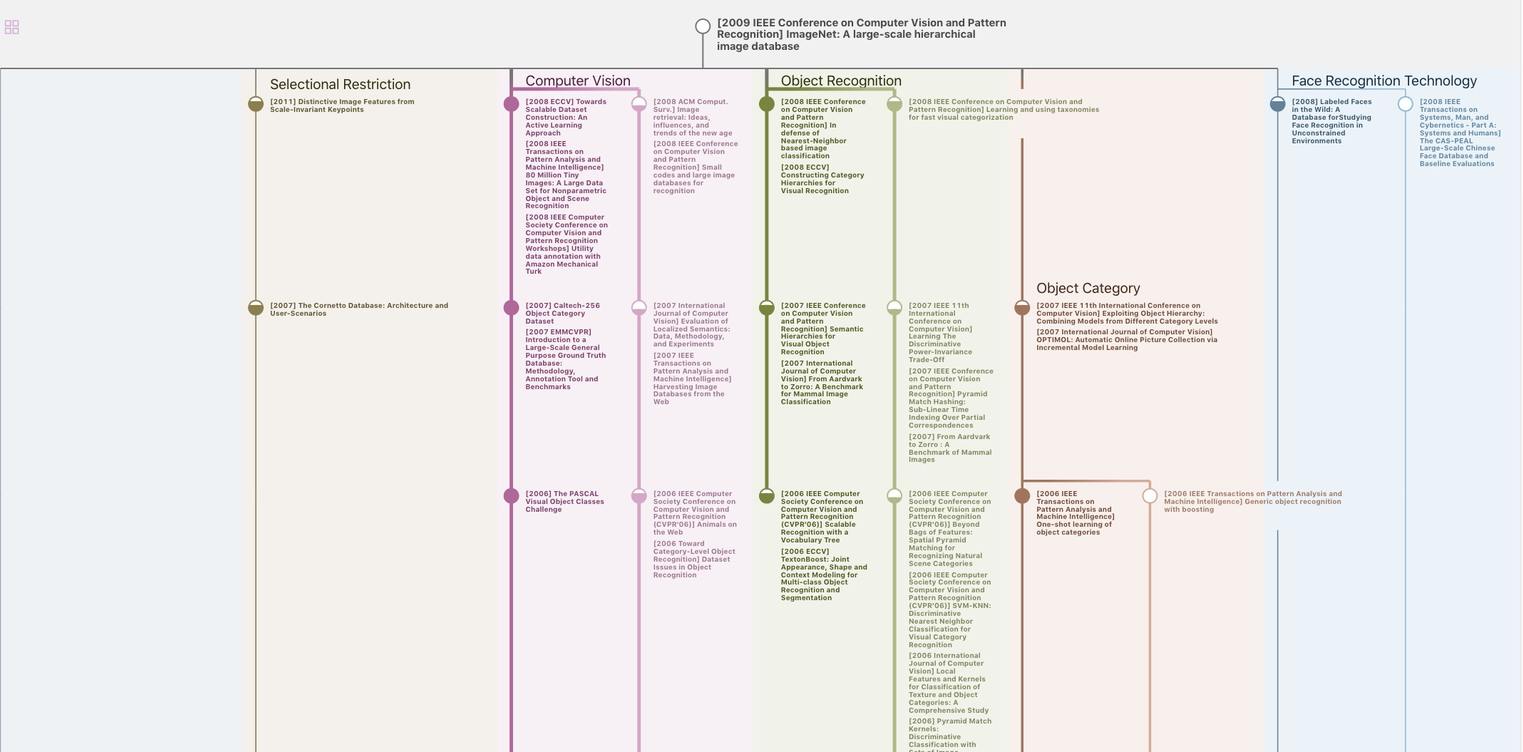
生成溯源树,研究论文发展脉络
Chat Paper
正在生成论文摘要