Dynamic Oracle for Neural Machine Translation in Decoding Phase
PROCEEDINGS OF THE ELEVENTH INTERNATIONAL CONFERENCE ON LANGUAGE RESOURCES AND EVALUATION (LREC 2018)(2017)
摘要
The past several years have witnessed the rapid progress of end-to-end Neural Machine Translation (NMT). However, there exists discrepancy between training and inference in NMT when decoding, which may lead to serious problems since the model might be in a part of the state space it has never seen during training. To address the issue, Scheduled Sampling has been proposed. However, there are certain limitations in Scheduled Sampling and we propose two dynamic oracle-based methods to improve it. We manage to mitigate the discrepancy by changing the training process towards a less guided scheme and meanwhile aggregating the oracle's demonstrations. Experimental results show that the proposed approaches improve translation quality over standard NMT system.
更多查看译文
关键词
machine translation, dynamic oracle, language model
AI 理解论文
溯源树
样例
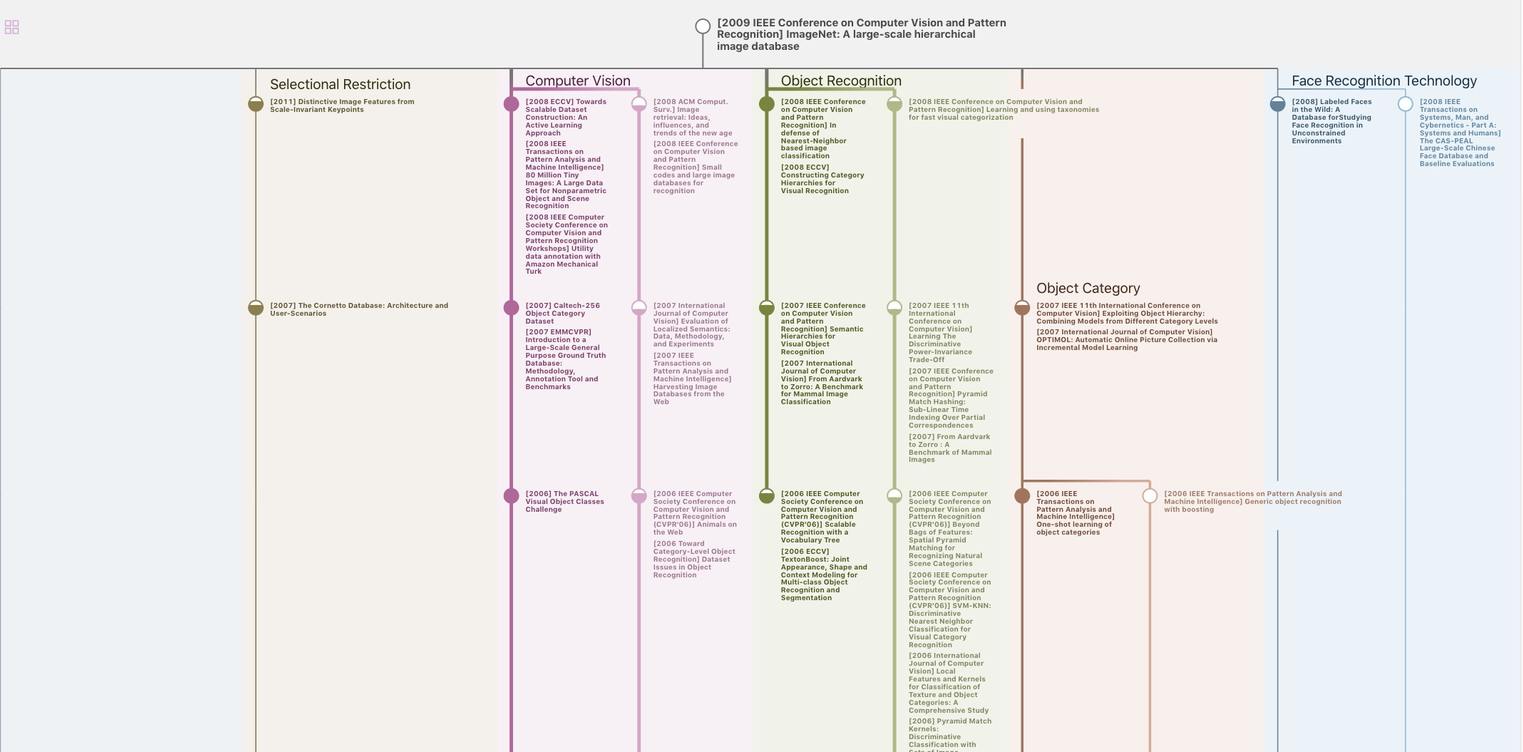
生成溯源树,研究论文发展脉络
Chat Paper
正在生成论文摘要