Dropout as a Low-Rank Regularizer for Matrix Factorization
INTERNATIONAL CONFERENCE ON ARTIFICIAL INTELLIGENCE AND STATISTICS, VOL 84(2018)
摘要
Dropout is a simple yet effective regularization technique that has been applied to various machine learning tasks, including linear classification, matrix factorization (MF) and deep learning. However, despite its solid empirical performance, the theoretical properties of dropout as a regularizer remain quite elusive. In this paper, we present a theoretical analysis of dropout for MF, where Bernoulli random variables are used to drop columns of the factors. We demonstrate the equivalence between dropout and a fully deterministic model for MF in which the factors are regularized by the sum of the product of squared Euclidean norms of the columns. Additionally, we investigate the case of a variable sized factorization and we prove that dropout is equivalent to a convex approximation problem with (squared) nuclear norm regularization. As a consequence, we conclude that dropout induces a low-rank regularizer that results in a data dependent singular-value thresholding.
更多查看译文
AI 理解论文
溯源树
样例
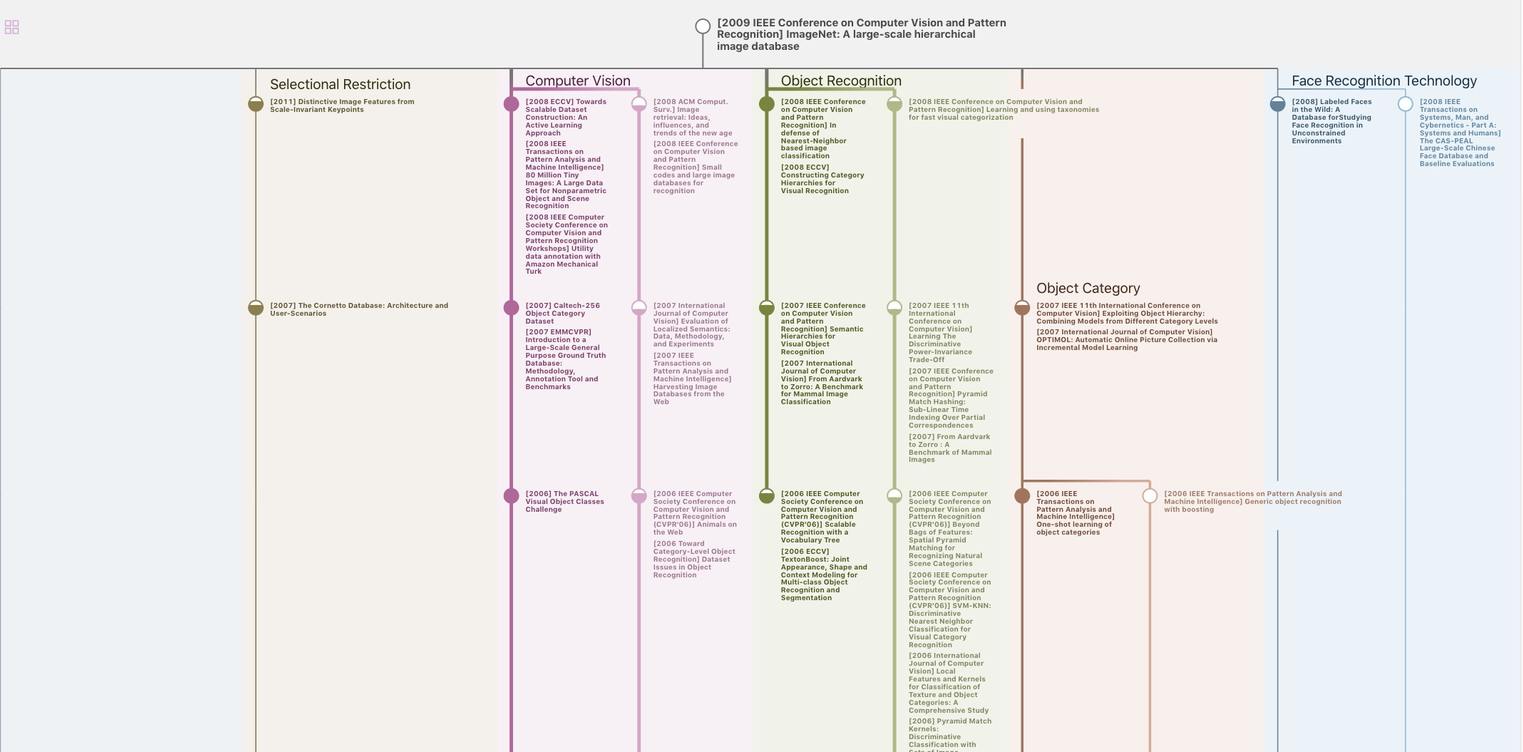
生成溯源树,研究论文发展脉络
Chat Paper
正在生成论文摘要