Automata-Guided Hierarchical Reinforcement Learning for Skill Composition.
arXiv: Artificial Intelligence(2017)
摘要
Skills learned through (deep) reinforcement learning often generalizes poorly across domains and re-training is necessary when presented with a new task. We present a framework that combines techniques in textit{formal methods} with textit{reinforcement learning} (RL). The methods we provide allows for convenient specification of tasks with logical expressions, learns hierarchical policies (meta-controller and low-level controllers) with well-defined intrinsic rewards, and construct new skills from existing ones with little to no additional exploration. We evaluate the proposed methods in a simple grid world simulation as well as a more complicated kitchen environment in AI2Thor
更多查看译文
AI 理解论文
溯源树
样例
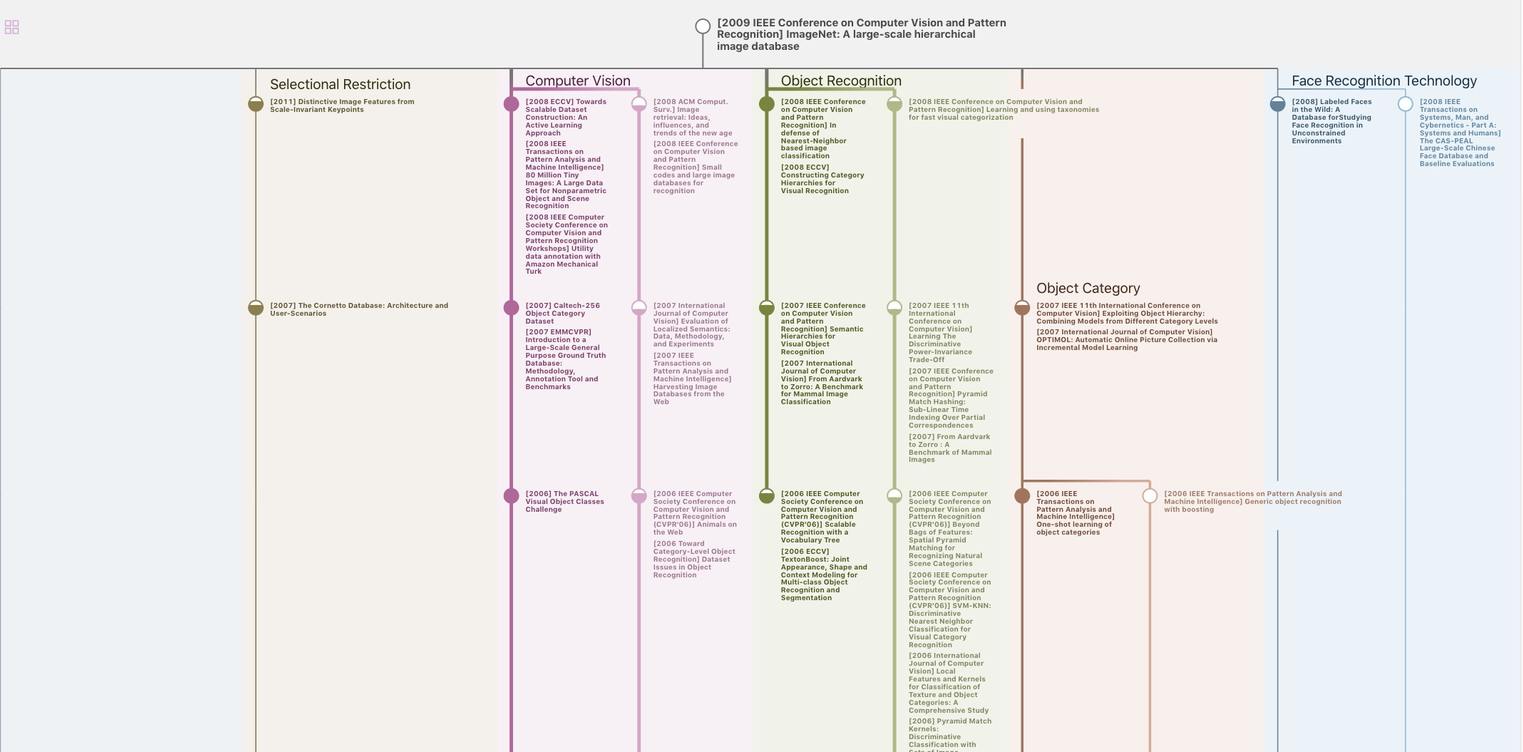
生成溯源树,研究论文发展脉络
Chat Paper
正在生成论文摘要