Session-based item recommendation in e-commerce: on short-term intents, reminders, trends and discounts
User Model. User-Adapt. Interact.(2017)
摘要
Many e-commerce sites present additional item recommendations to their visitors while they navigate the site, and ample evidence exists that such recommendations are valuable for both customers and providers. Academic research often focuses on the capability of recommender systems to help users discover items they presumably do not know yet and which match their long-term preference profiles. In reality, however, recommendations can be helpful for customers also for other reasons, for example, when they remind them of items they were recently interested in or when they point site visitors to items that are currently discounted. In this work, we first adopt a systematic statistical approach to analyze what makes recommendations effective in practice and then propose ways of operationalizing these insights into novel recommendation algorithms. Our data analysis is based on log data of a large e-commerce site. It shows that various factors should be considered in parallel when selecting items for recommendation, including their match with the customer’s shopping interests in the previous sessions, the general popularity of the items in the last few days, as well as information about discounts. Based on these analyses we propose a novel algorithm that combines a neighborhood-based scheme with a deep neural network to predict the relevance of items for a given shopping session.
更多查看译文
关键词
Recommender systems,E-commerce,Session-based Recommendation,Context-aware Recommendation
AI 理解论文
溯源树
样例
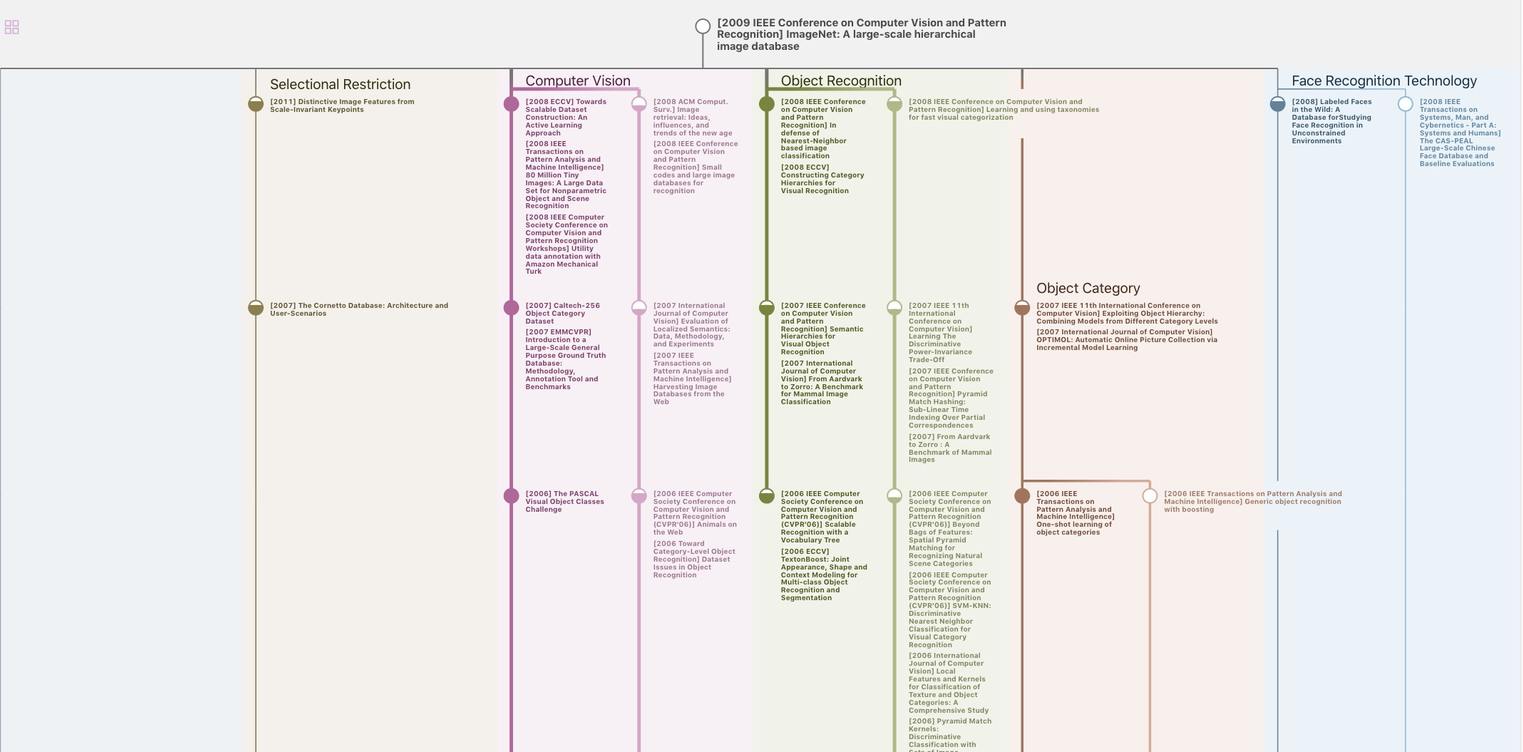
生成溯源树,研究论文发展脉络
Chat Paper
正在生成论文摘要