Decision making in health care using robust parameter design with conditions-based selection of regression estimators.
QUALITY AND RELIABILITY ENGINEERING INTERNATIONAL(2017)
摘要
Health care professionals often use regression methods to quantitatively describe the functional relationships between predictors and outcomes. However, little research investigates the appropriateness of tool application for response-surface-based design of experiments, the choice of regression estimators under different environments, and the impact of the determination of optimum conditions, or optimal factor-level settings, to achieve desirable target outcomes. Robust parameter design (RPD) is an established methodology for determining optimum conditions for a process to achieve specified process targets while minimizing variability of the outcomes. Underlying assumptions for RPD modeling and process conditions should be taken into account when selecting a regression estimator for developing fitted models. If these assumptions are incorrect, then a direct use of estimates obtained has the potential to be problematic, and the results may be potentially catastrophic, particularly when applied to the health care field. Many approaches to RPD in existing literature use ordinary least squares to obtain response functions by assuming normality and moderate variability in the underlying process. Given that some biologic processes are often highly variable and inherently asymmetric, a conditions-based approach for the selection of a regression estimate technique needs to be explored. This paper examines alternative approaches to regression estimation when the process data indicates that asymmetry or a high degree of process variability exists. The performance of select alternative regression methods is compared using Monte Carlo simulation and numerical analysis.
更多查看译文
关键词
estimators,health care,Monte Carlo simulation,optimum conditions,quality
AI 理解论文
溯源树
样例
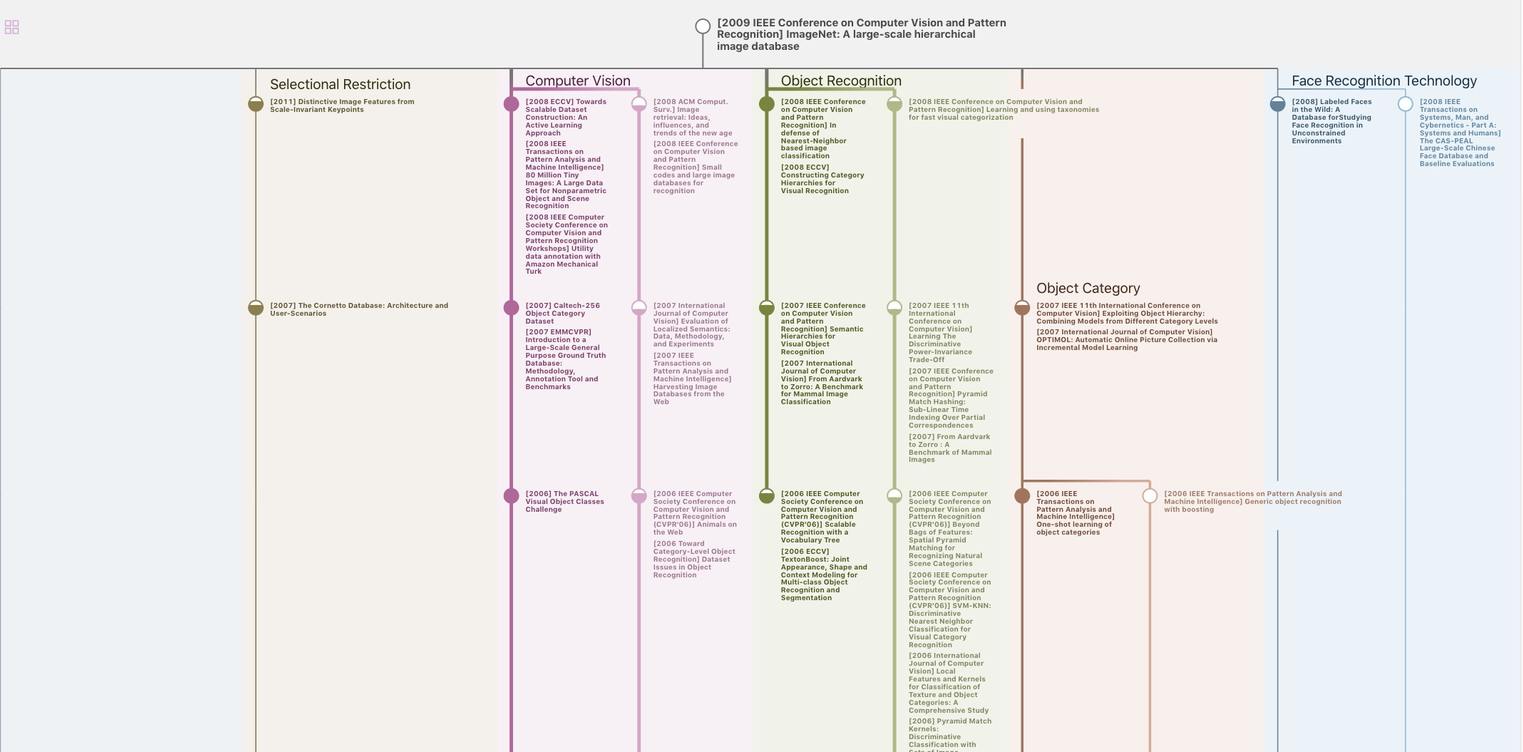
生成溯源树,研究论文发展脉络
Chat Paper
正在生成论文摘要