Adaptive activation functions in convolutional neural networks.
Neurocomputing(2018)
摘要
Activation functions play important roles in deep convolutional neural networks. This work focuses on learning activation functions via combining basic activation functions in a data-driven way. We explore three strategies to learn the activation functions, and allow the activation operation to be adaptive to inputs. We firstly explore two strategies to linearly and nonlinearly combine basic activation functions, respectively. Then we further investigate a strategy that basic activation functions are combined in a way of a hierarchical integration. Experiments demonstrate that the proposed activation functions lead to better performances than ReLU and its variants on benchmarks with various scales.
更多查看译文
关键词
Convolutional neural networks,Activation function learning,Adaptive activation,Hierarchical activation
AI 理解论文
溯源树
样例
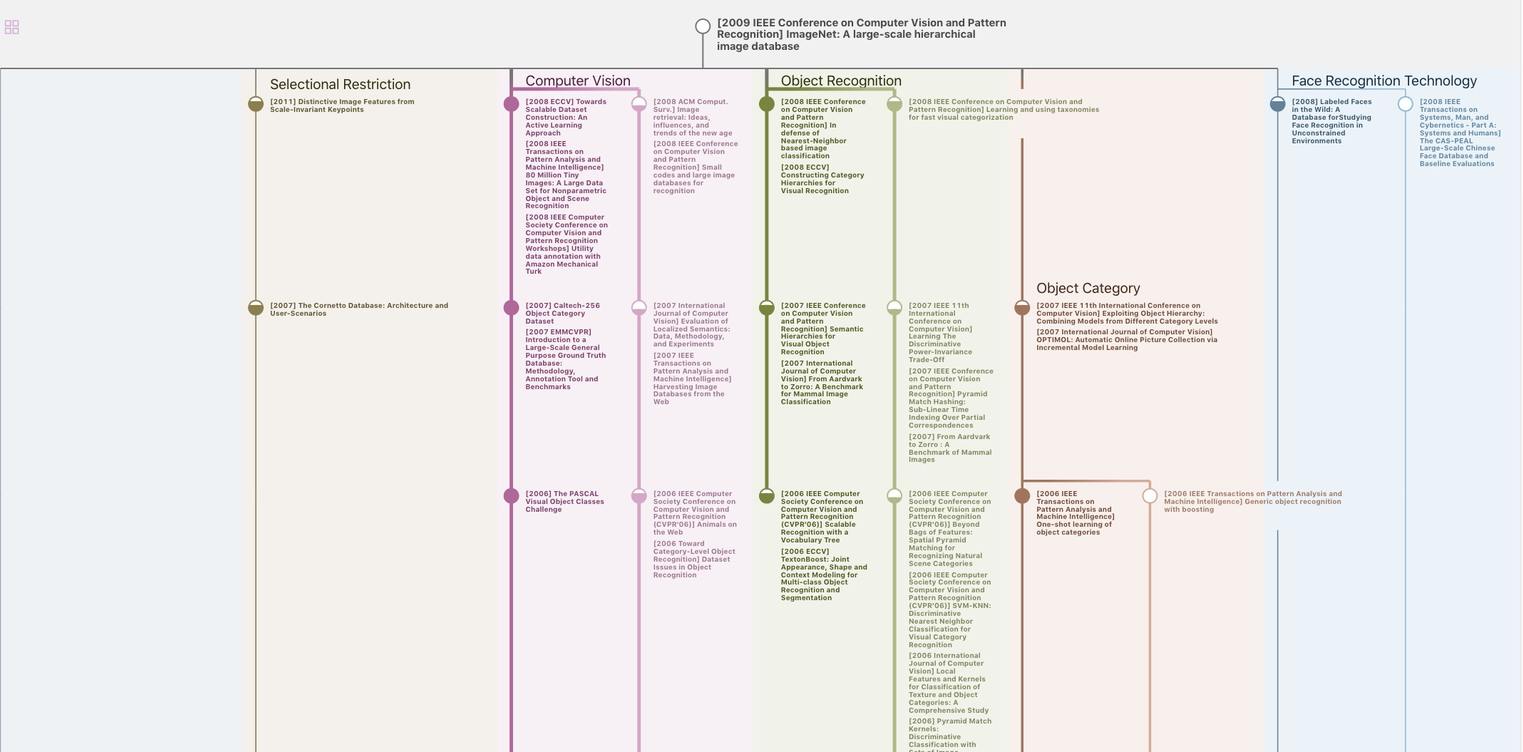
生成溯源树,研究论文发展脉络
Chat Paper
正在生成论文摘要