Sharp Bounds For Generalized Uniformity Testing
ADVANCES IN NEURAL INFORMATION PROCESSING SYSTEMS 31 (NIPS 2018)(2018)
摘要
We study the problem of generalized uniformity testing of a discrete probability distribution: Given samples from a probability distribution p over an unknown size discrete domain Omega, we want to distinguish, with probability at least 2/3, between the case that p is uniform on some subset of Omega versus epsilon-far, in total variation distance, from any such uniform distribution. We establish tight bounds on the sample complexity of generalized uniformity testing. In more detail, we present a computationally efficient tester whose sample complexity is optimal, within constant factors, and a matching worst-case information-theoretic lower bound. Specifically, we show that the sample complexity of generalized uniformity testing is Theta (1/(epsilon(4/3)parallel to p parallel to(3)) + 1/(epsilon(2)parallel to p parallel to(2))).
更多查看译文
关键词
uniform distribution,computationally efficient,sample complexity,probability distribution,discrete probability distribution,constant factors,total variation distance
AI 理解论文
溯源树
样例
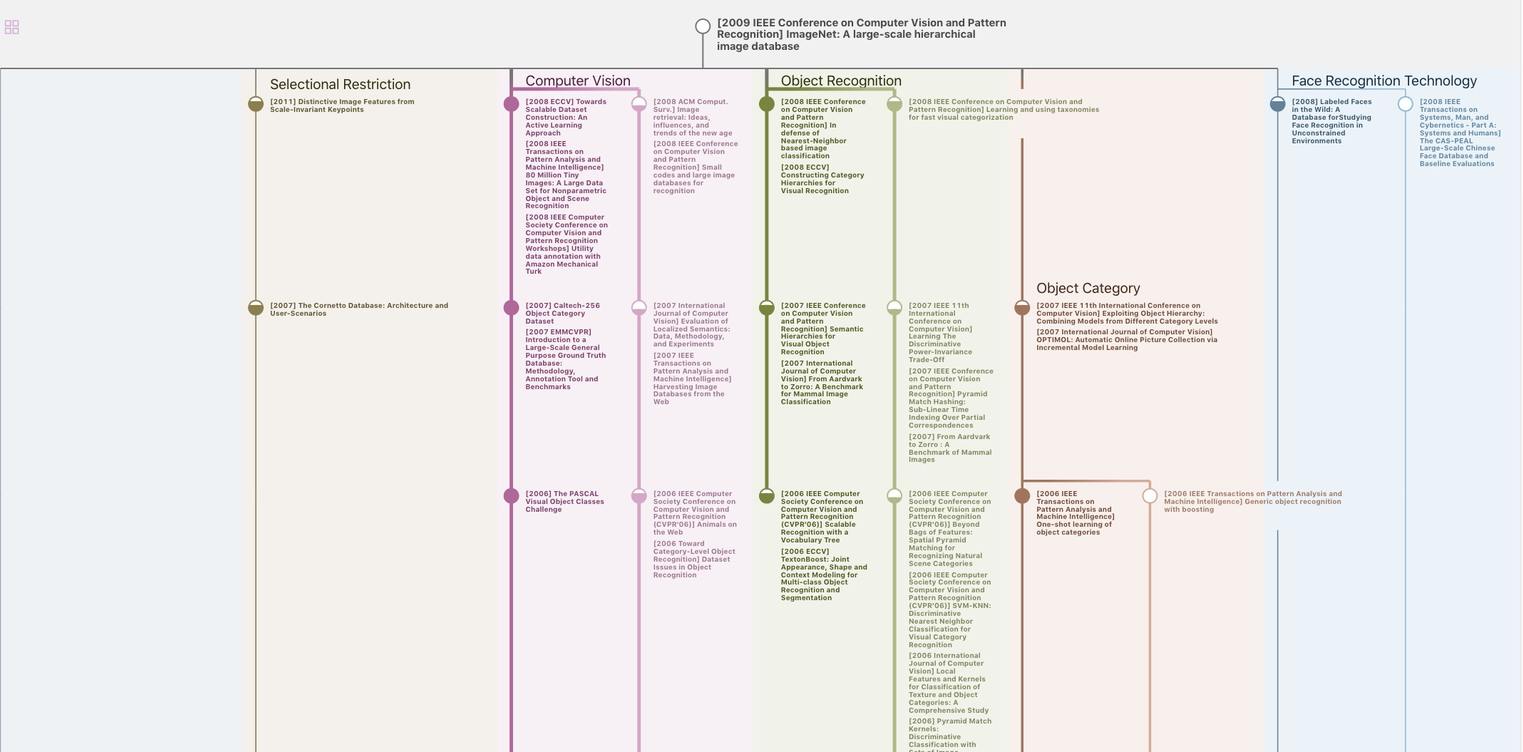
生成溯源树,研究论文发展脉络
Chat Paper
正在生成论文摘要