Spatiotemporal Modeling And Prediction In Cellular Networks: A Big Data Enabled Deep Learning Approach
IEEE INFOCOM 2017 - IEEE CONFERENCE ON COMPUTER COMMUNICATIONS(2017)
摘要
In this paper, we propose to leverage the emerging deep learning techniques for spatiotemporal modeling and prediction in cellular networks, based on big system data. First, we perform a preliminary analysis for a big dataset from China Mobile, and use traffic load as an example to show non-zero temporal autocorrelation and non-zero spatial correlation among neighboring Base Stations (BSs), which motivate us to discover both temporal and spatial dependencies in our study. Then we present a hybrid deep learning model for spatiotemporal prediction, which includes a novel autoencoder-based deep model for spatial modeling and Long Short-Term Memory units (LSTMs) for temporal modeling. The autoencoder-based model consists of a Global Stacked AutoEncoder (GSAE) and multiple Local SAEs (LSAEs), which can offer good representations for input data, reduced model size, and support for parallel and application-aware training. Moreover, we present a new algorithm for training the proposed spatial model. We conducted extensive experiments to evaluate the performance of the proposed model using the China Mobile dataset. The results show that the proposed deep model significantly improves prediction accuracy compared to two commonly used baseline methods, ARIMA and SVR. We also present some results to justify effectiveness of the autoencoder-based spatial model.
更多查看译文
关键词
Cellular Network,Big Data,Spatiotemporal Modeling,Deep Learning,Autoencoder,Recurrent Neural Network
AI 理解论文
溯源树
样例
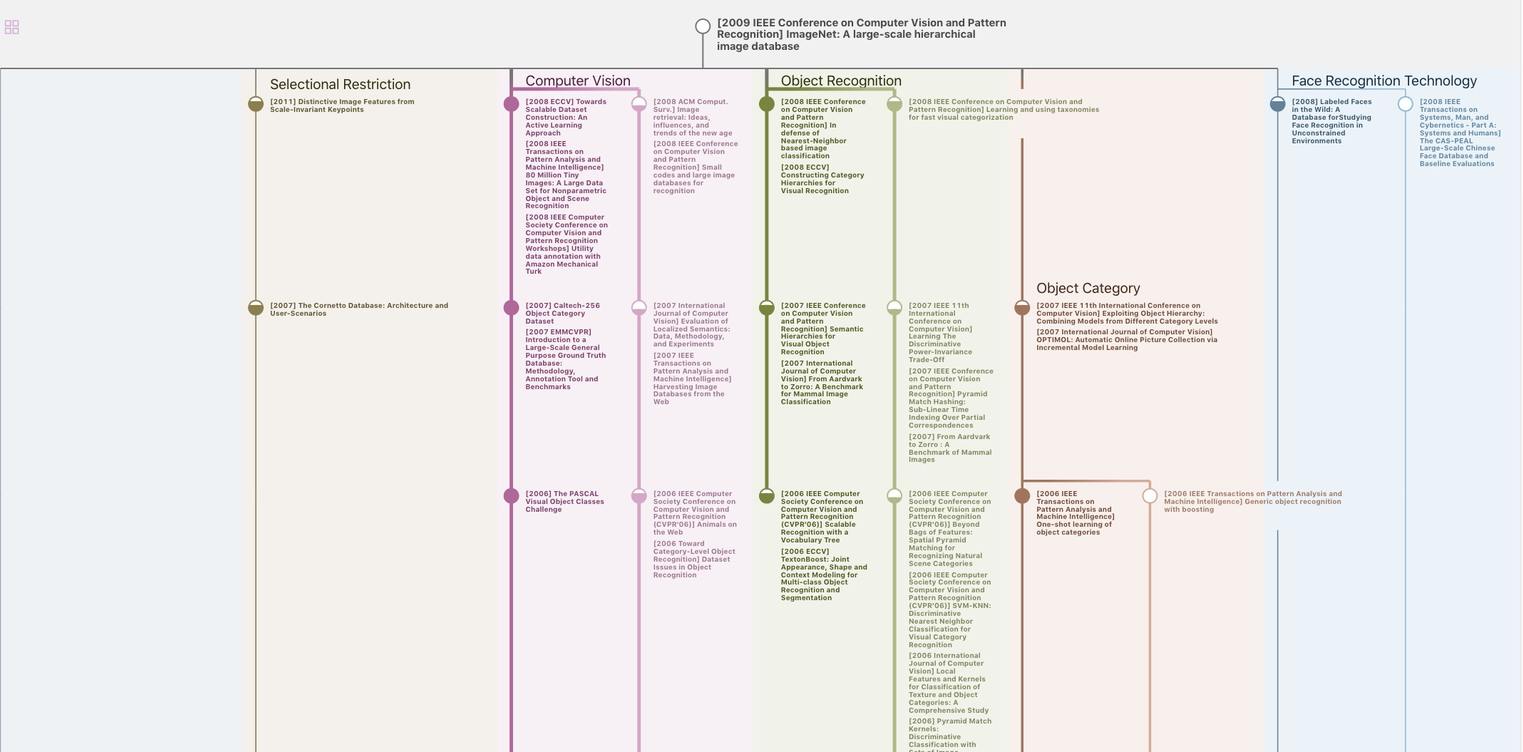
生成溯源树,研究论文发展脉络
Chat Paper
正在生成论文摘要