Improving K-Nn Graph Accuracy Using Local Intrinsic Dimensionality
SISAP(2017)
摘要
The k-nearest neighbor (k-NN) graph is an important data structure for many data mining and machine learning applications. The accuracy of k-NN graphs depends on the object feature vectors, which are usually represented in high-dimensional spaces. Selecting the most important features is essential for providing compact object representations and for improving the graph accuracy. Having a compact feature vector can reduce the storage space and the computational complexity of search and learning tasks. In this paper, we propose NNWID-Descent, a similarity graph construction method that utilizes the NNF-Descent framework while integrating a new feature selection criterion, Support-Weighted Intrinsic Dimensionality, that estimates the contribution of each feature to the overall intrinsic dimensionality. Through extensive experiments on various datasets, we show that NNWID-Descent allows a significant amount of local feature vector sparsification while still preserving a reasonable level of graph accuracy.
更多查看译文
关键词
Intrinsic dimensionality,k-nearest neighbor graph,Feature selection,Vector sparsification
AI 理解论文
溯源树
样例
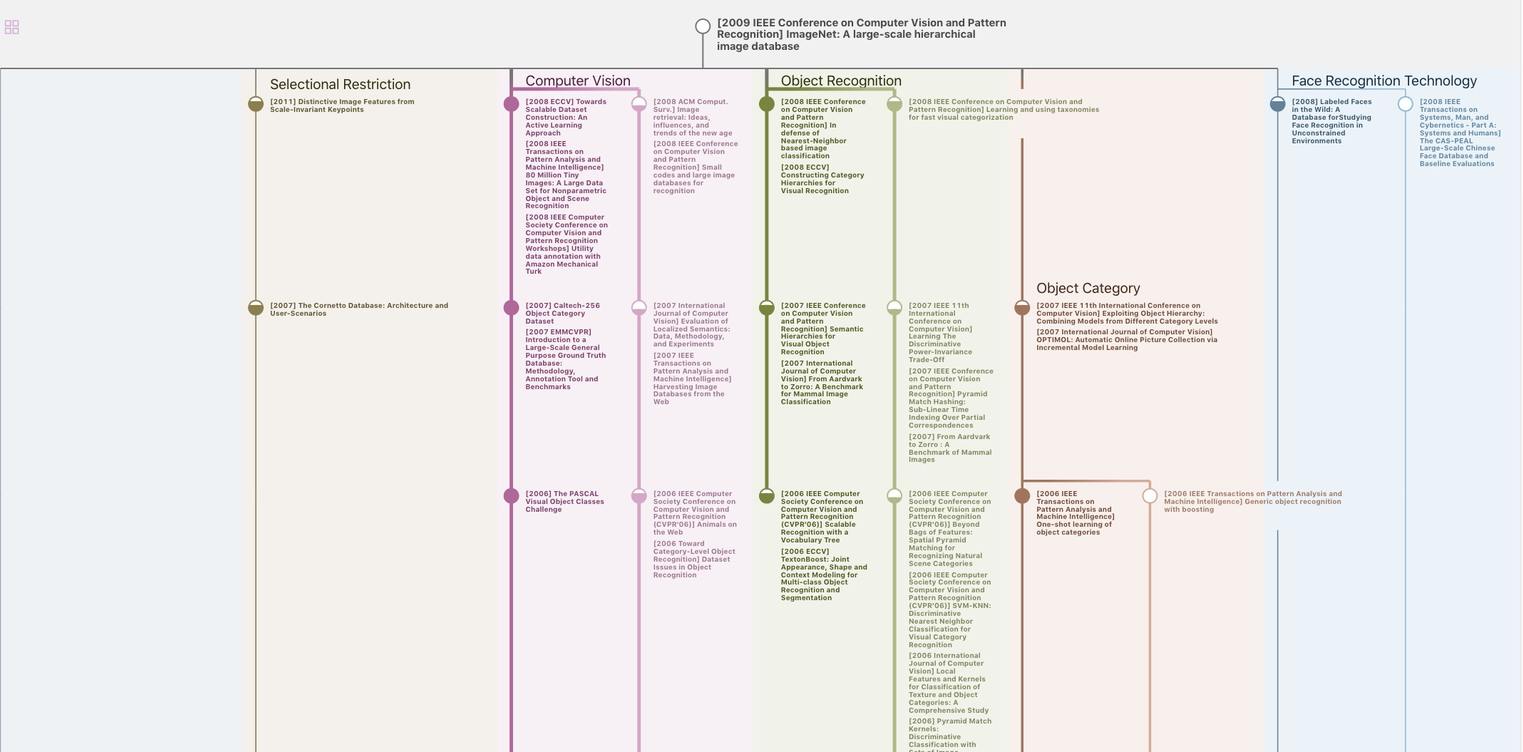
生成溯源树,研究论文发展脉络
Chat Paper
正在生成论文摘要