Locality-Constrained Transfer Coding For Heterogeneous Domain Adaptation
DATABASES THEORY AND APPLICATIONS, ADC 2017(2017)
摘要
Currently, most of widely used databases are label-wise. In other words, people organize their data with corresponding labels, e.g., class information, keywords and description, for the convenience of indexing and retrieving. However, labels of the data from a novel application usually are not available, and labeling by hand is very expensive. To address this, we propose a novel approach based on transfer learning. Specifically, we aim at tackling heterogeneous domain adaptation (HDA). HDA is a crucial topic in transfer learning. Two inevitable issues, feature discrepancy and distribution divergence, get in the way of HDA. However, due to the significant challenges of HDA, previous work commonly focus on handling one of them and neglect the other. Here we propose to deploy locality-constrained transfer coding (LCTC) to simultaneously alleviate the feature discrepancy and mitigate the distribution divergence. Our method is powered by two tactics: feature alignment and distribution alignment. The former learns new transferable feature representations by sharing-dictionary coding and the latter aligns the distribution gaps on the new feature space. By formulating the problem into a unified objective and optimizing it via an iterative fashion, the two tactics are reinforced by each other and the two domains are drawn closer under the new representations. Extensive experiments on image classification and text categorization verify the superiority of our method against several state-of-the-art approaches.
更多查看译文
关键词
Domain adaptation, Transfer learning, Knowledge discovery
AI 理解论文
溯源树
样例
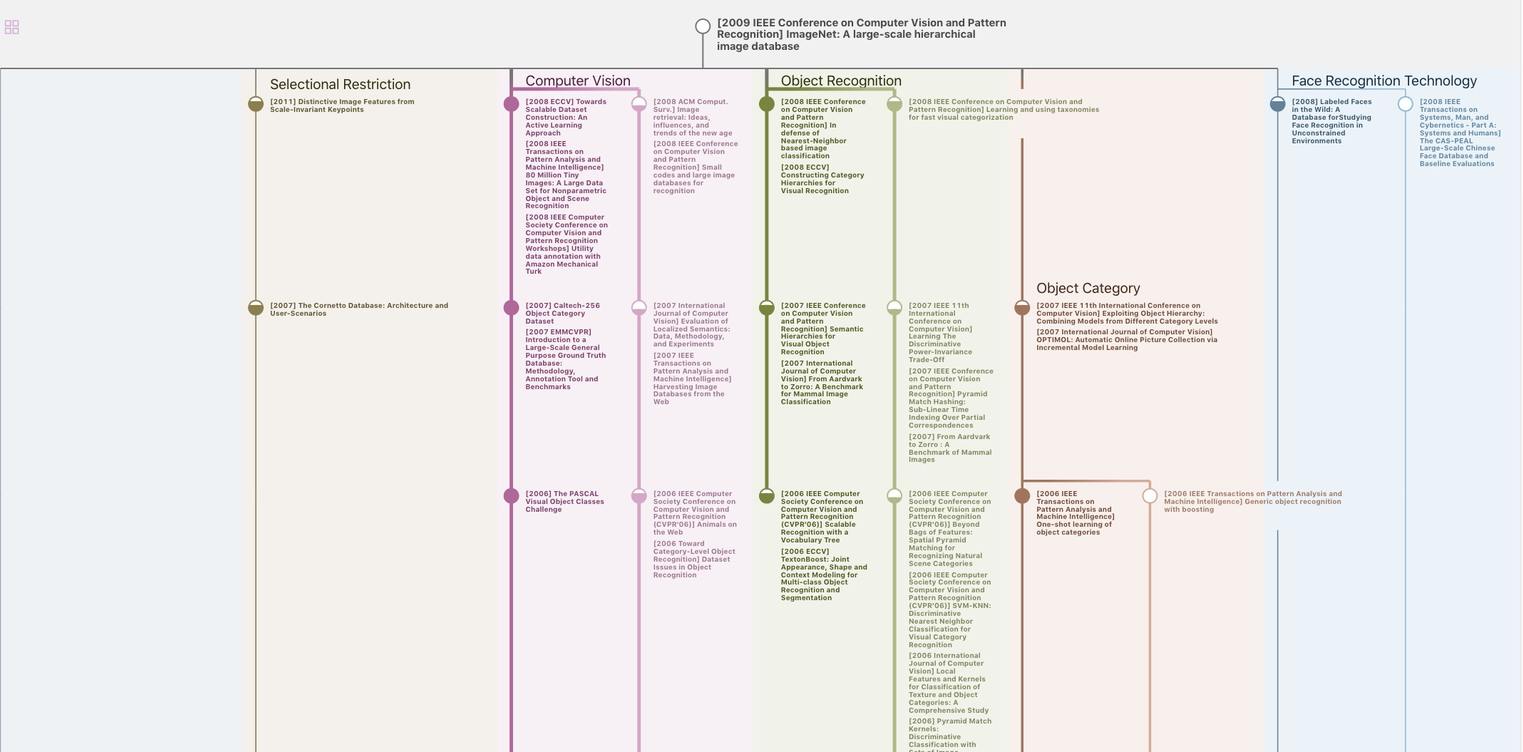
生成溯源树,研究论文发展脉络
Chat Paper
正在生成论文摘要