Terminology Translation Error Identification and Correction.
Communications in Computer and Information Science(2017)
摘要
Statistical machine translation (SMT) system requires homogeneous training data in order to get domain-sensitive terminology translations. If the data is multi-domain mixed, it is difficult for SMT system to learn translation probability of context-sensitive terminology. However, terminology translation is important for SMT. The previous work mainly focuses on integrating terminology into machine translation systems and heavily relies on domain terminology resources. In this paper, we propose a back translation based method to identify terminology translation errors from SMT outputs and automatically suggest a better translation. Our approach is simple with no external resources and can be applied to any type of SMT system. We use three metrics: tree-edit distance, sentence semantic similarity and language model perplexity to measure the quality of back translation. Experimental results illustrate that our method improves performance on both weak and strong SMT systems, yielding a precision of 0.48% and 1.51% respectively.
更多查看译文
关键词
Statistical machine translation,Domain terminology,Post-processing,Back translation
AI 理解论文
溯源树
样例
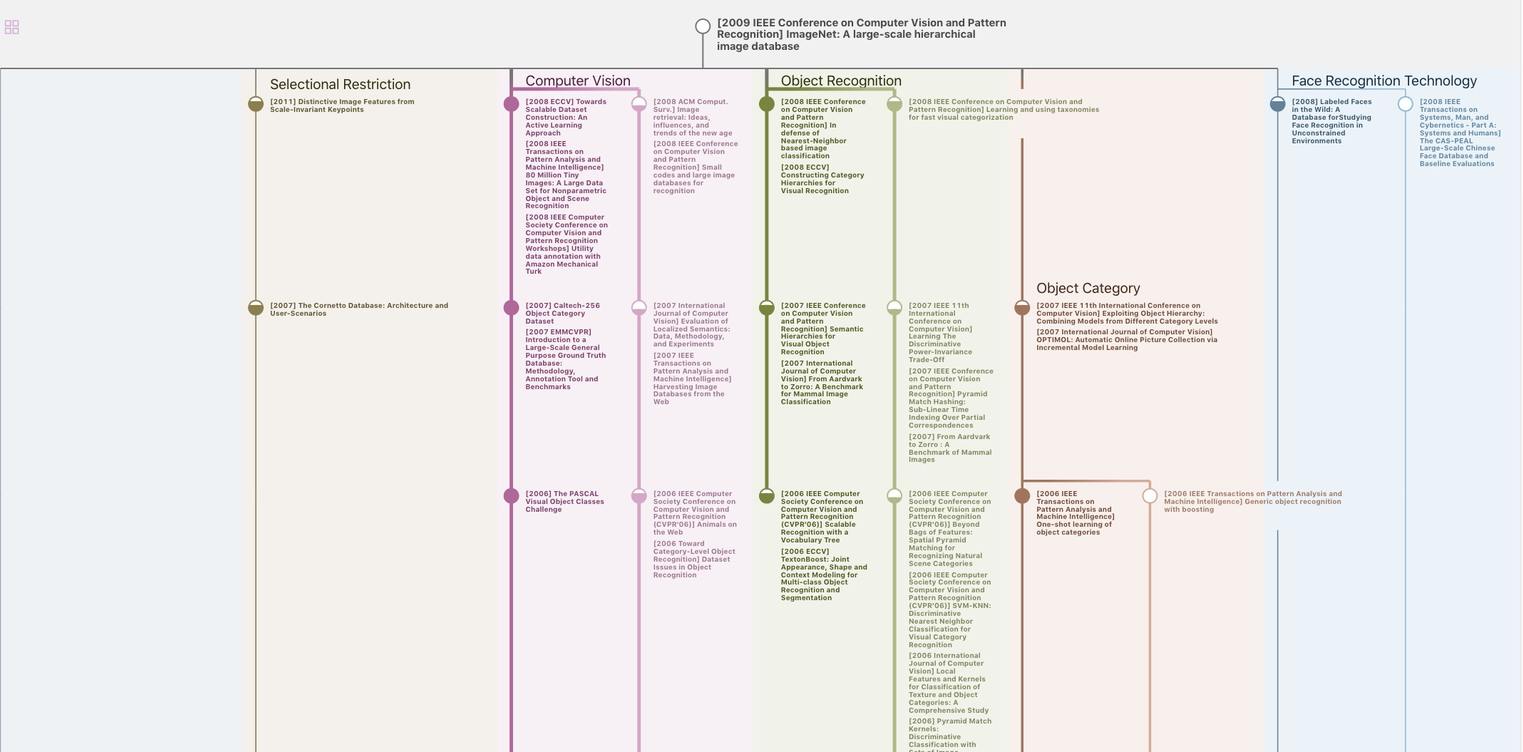
生成溯源树,研究论文发展脉络
Chat Paper
正在生成论文摘要