Efficient scalable hardware architecture for highly performant encoded neural networks
2017 IEEE International Workshop on Signal Processing Systems (SiPS)(2017)
摘要
Different neural network models have been proposed to design efficient associative memories like Hopfield networks, Boltzmann machines or Cogent confabulation. Compared to the classical models, Encoded Neural Network (ENN) is a recently introduced formalism with a proven higher efficiency. This model has been improved through different contributions like Clone-based ENN (CbNNs) or Sparse ENNs (S-ENNs) which enhance either the capacity of the original ENN or its retrieving performances. However, only very few works explored its hardware implementation for embedded applications. In this paper, we introduce a clone-based sparse neural network model (SC-ENN), that gathers the enhancements of the existing approaches in a single formal model. In addition, we present a dedicated scalable hardware architecture to implement SC-ENN. This work leads to significant complexity and area reduction without affecting neither memorizing nor retrieving performances. By only handling the most relevant information provided by the model, our proposed approach is far less expensive compared to state of the art solutions.
更多查看译文
关键词
Neuxal network,Hardware design,FPGA
AI 理解论文
溯源树
样例
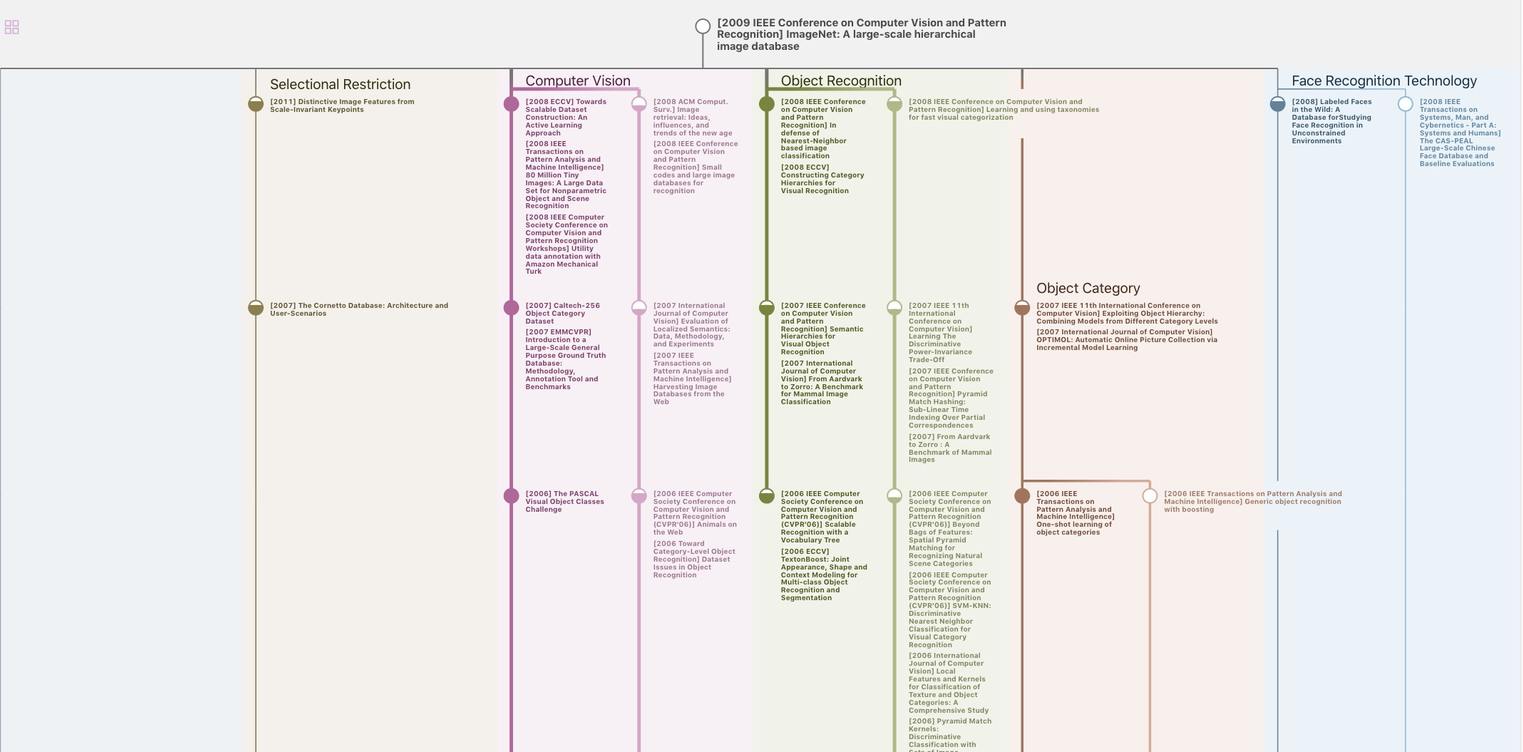
生成溯源树,研究论文发展脉络
Chat Paper
正在生成论文摘要