Automatic Sleep Stage Classification Applying Machine Learning Algorithms on EEG Recordings
2017 IEEE 30th International Symposium on Computer-Based Medical Systems (CBMS)(2017)
摘要
This paper focuses on developing a novel approach to automatic sleep stage classification based on electroencephalographic (EEG) data. The proposed methodology employs contemporary mathematical tools such as the synchronization likelihood and graph theory metrics applied on sleep EEG data. The derived features are then fitted into three different machine learning techniques, namely k-nearest neighbors, support vector machines and neural networks. The evaluation of their comparative performance is investigated according to their accuracy. Interestingly, the support vector machine achieves the maximum possible accuracy, i.e., 89.07%, which renders it as a suitable method for sleep stage classification.
更多查看译文
关键词
sleep staging,machine learning,synchronization likelihood,bio-signal processing
AI 理解论文
溯源树
样例
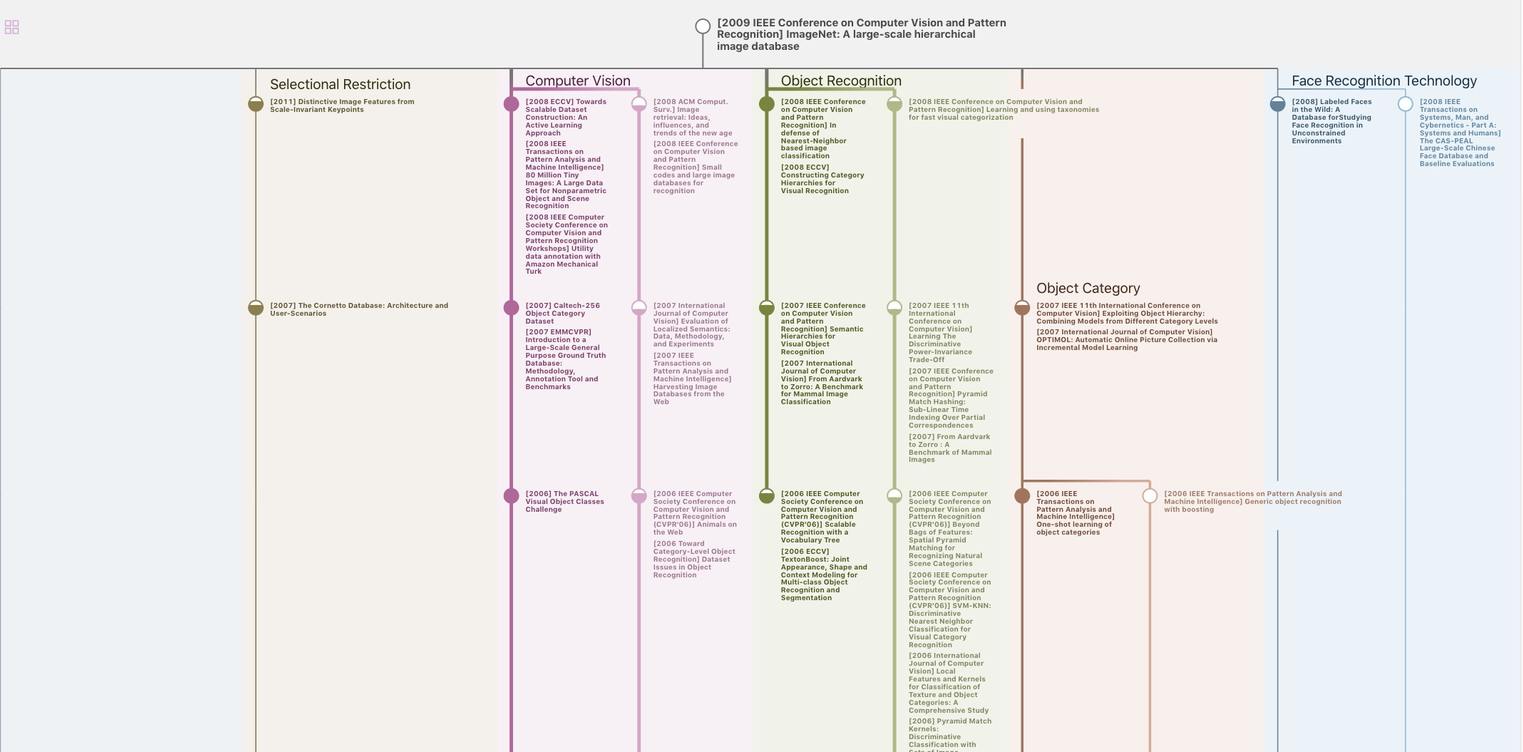
生成溯源树,研究论文发展脉络
Chat Paper
正在生成论文摘要