Efficient Document Filtering Using Vector Space Topic Expansion and Pattern-Mining: The Case of Event Detection in Microposts.
CIKM(2017)
摘要
Automatically extracting information from social media is challenging given that social content is often noisy, ambiguous, and inconsistent. However, as many stories break on social channels first before being picked up by mainstream media, developing methods to better handle social content is of utmost importance. In this paper, we propose a robust and effective approach to automatically identify microposts related to a specific topic defined by a small sample of reference documents. Our framework extracts clusters of semantically similar microposts that overlap with the reference documents, by extracting combinations of key features that define those clusters through frequent pattern mining. This allows us to construct compact and interpretable representations of the topic, dramatically decreasing the computational burden compared to classical clustering and k-NN-based machine learning techniques and producing highly-competitive results even with small training sets (less than 1'000 training objects). Our method is efficient and scales gracefully with large sets of incoming microposts. We experimentally validate our approach on a large corpus of over 60M microposts, showing that it significantly outperforms state-of-the-art techniques.
更多查看译文
关键词
Event detection, microposts, frequent patterns mining, semantic attributes
AI 理解论文
溯源树
样例
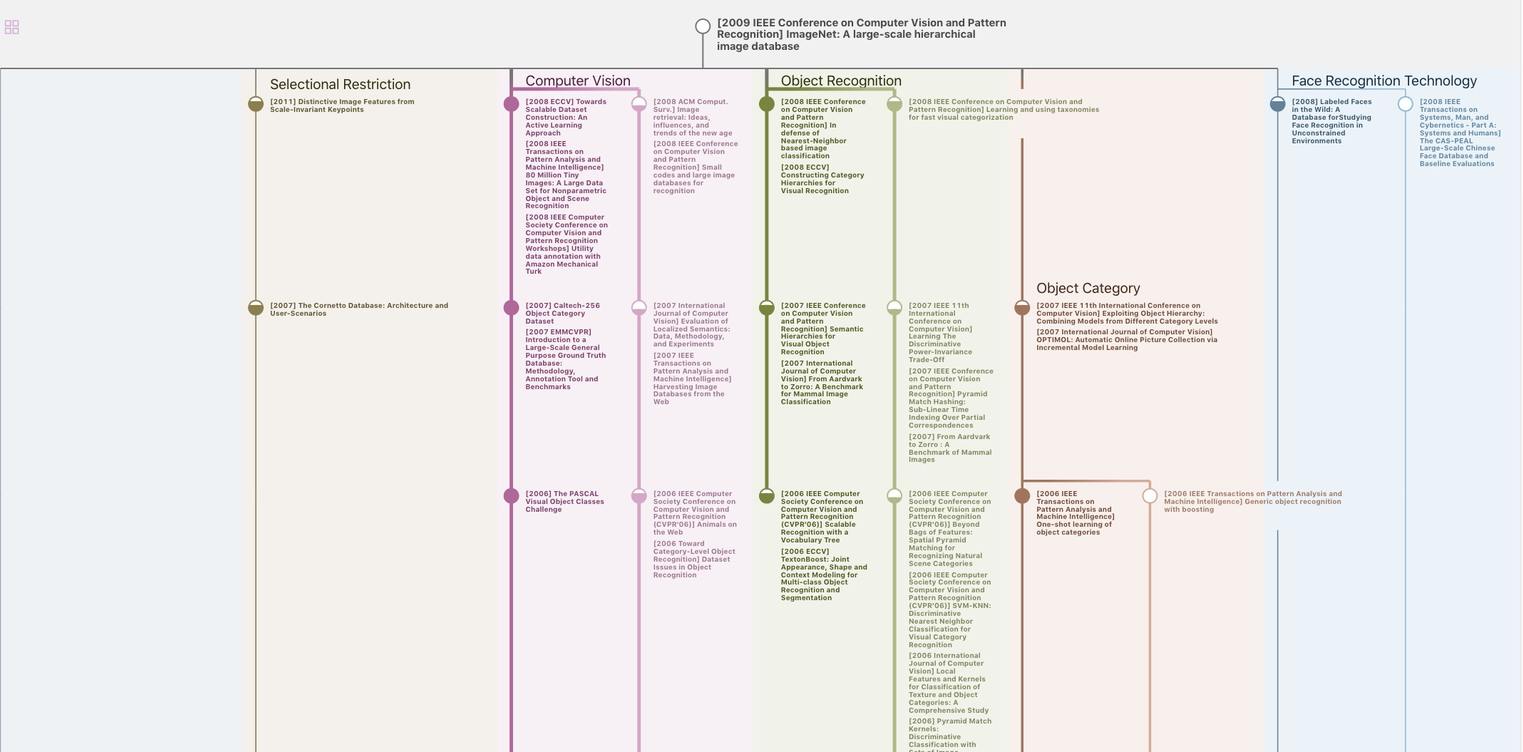
生成溯源树,研究论文发展脉络
Chat Paper
正在生成论文摘要