Community-Based Network Alignment for Large Attributed Network.
CIKM(2017)
摘要
Network alignment is becoming an active topic in network data analysis. Despite extensive research, we realize that efficient use of topological and attribute information for large attributed network alignment has not been sufficiently addressed in previous studies. In this paper, based on Stochastic Block Model (SBM) and Dirichlet-multinomial, we propose "divide-and-conquer" models CAlign that jointly consider network alignment, community discovery and community alignment in one framework for large networks with node attributes, in an effort to reduce both the computation time and memory usage while achieving better or competitive performance. It is provable that the algorithms derived from our model have sub-quadratic time complexity and linear space complexity on a network with small densification power, which is true for most real-world networks. Experiments show CAlign is superior to two recent state-of-art models in terms of accuracy, time and memory on large networks, and CAlign is capable of handling millions of nodes on a modern desktop machine.
更多查看译文
关键词
Attributed Network Alignment, Community Discovery, Large Network, Stochastic Block Model, Dirichilet-Mutinomial
AI 理解论文
溯源树
样例
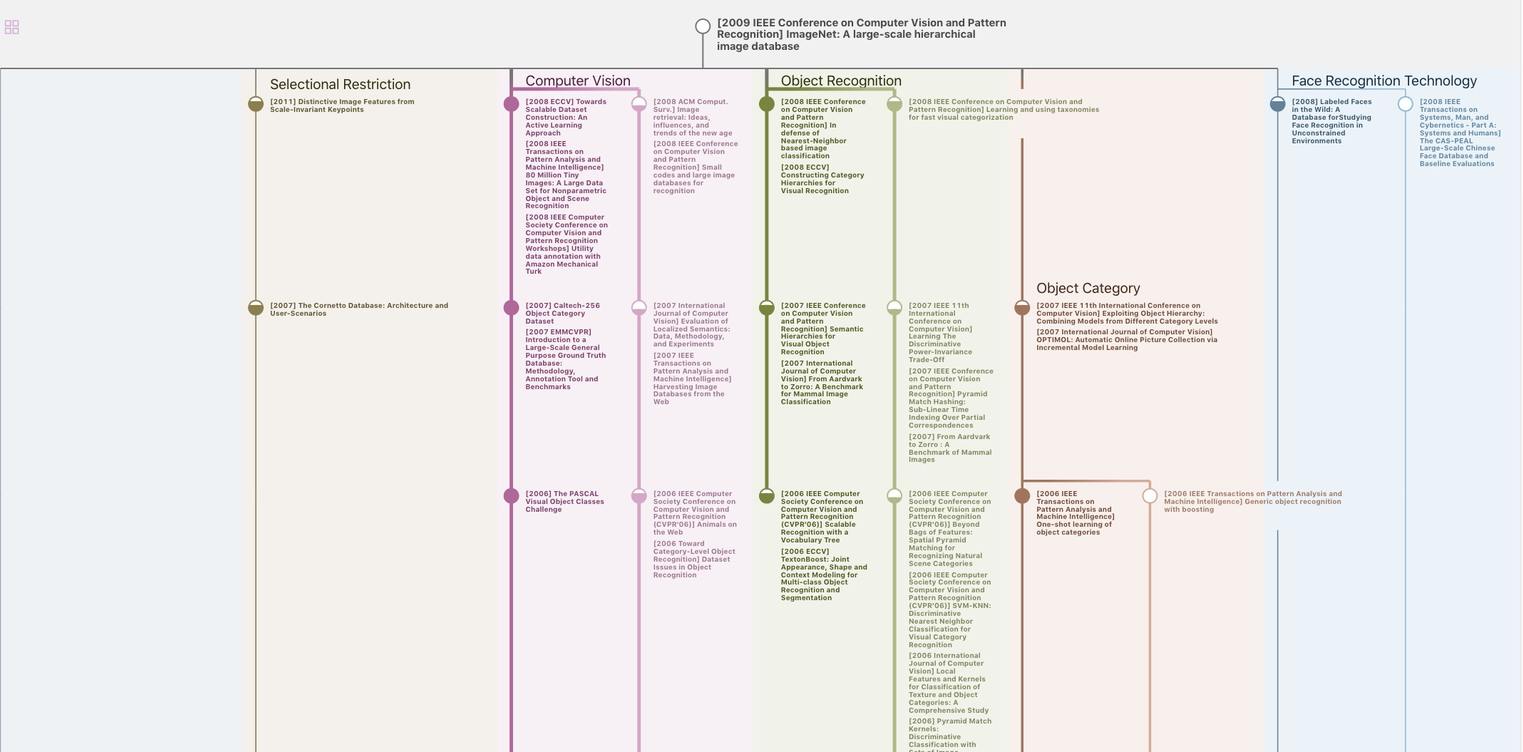
生成溯源树,研究论文发展脉络
Chat Paper
正在生成论文摘要