Locality-Based Graph Clustering of Spatially Embedded Time Series.
COMPLEX NETWORKS(2017)
摘要
The growing amount of sensor data poses significant challenges for rapid and meaningful analysis of large datasets. Across different scientific disciplines, complex networks are used to detect connections between entities of a system. The computation of similarity coefficients for complex network generation and the clustering of the same come at high computational costs. We propose a locality-based approach to analyze spatially embedded objects efficiently and reduce the computational costs of clustering. By exploiting high locality in time series datasets, we heavily reduce the search space and improve the runtime complexity from quadratic to quasi linear. We show the advantages of our approach for global and local clustering on real-world and synthetic datasets.
更多查看译文
AI 理解论文
溯源树
样例
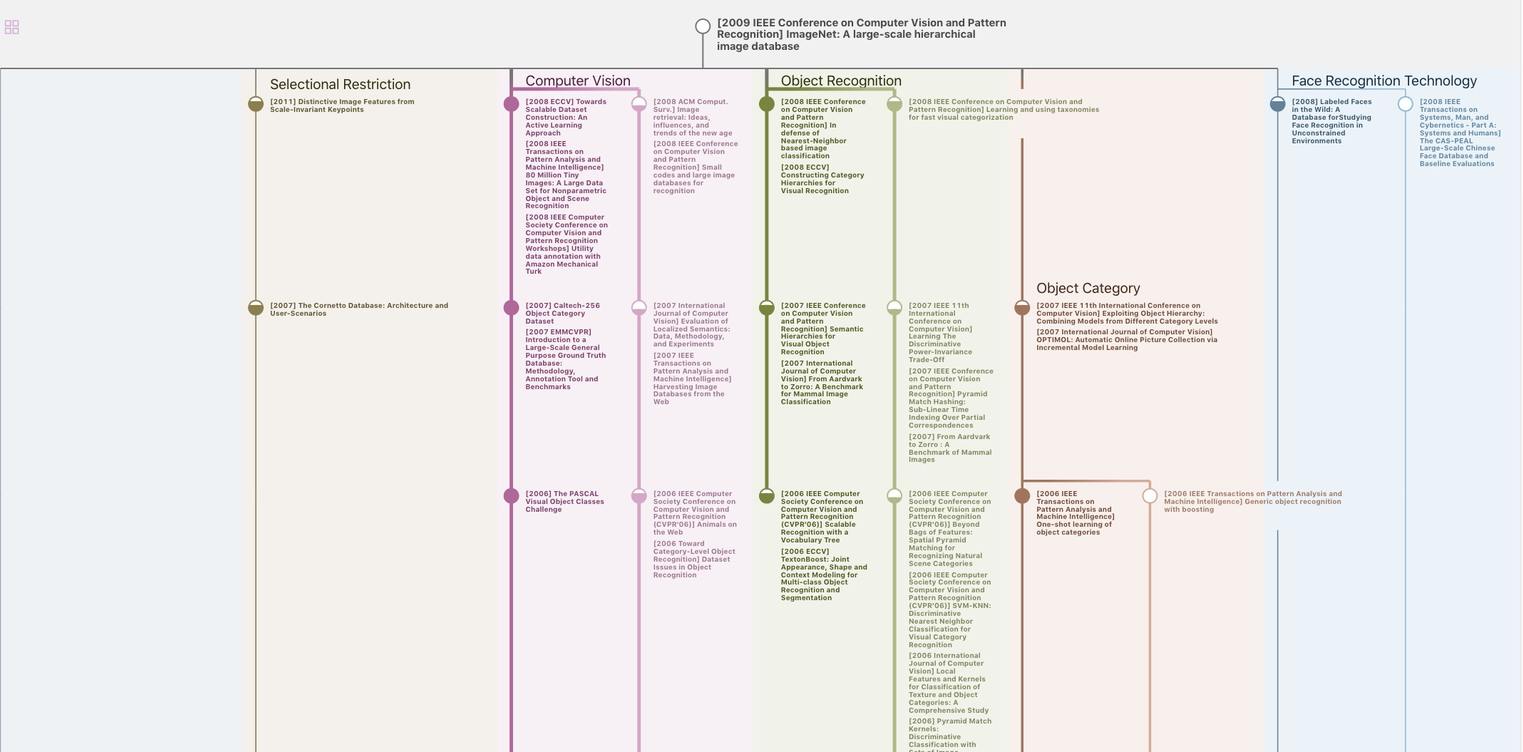
生成溯源树,研究论文发展脉络
Chat Paper
正在生成论文摘要