Stripe: Signal Selection For Runtime Power Estimation
2017 27TH INTERNATIONAL CONFERENCE ON FIELD PROGRAMMABLE LOGIC AND APPLICATIONS (FPL)(2017)
摘要
Knowledge of power consumption at a subsystem level can facilitate adaptive energy-saving techniques such as power gating, runtime task mapping and dynamic voltage and/or frequency scaling. While we have the ability to attribute power to an arbitrary hardware system's modules in real time, the selection of the particular signals to monitor for the purpose of power estimation within any given module has yet to be treated as a primary concern. In this paper, we show how the automatic analysis of circuit structure and behaviour inferred through vectored simulation can be used to produce high-quality rankings of signals' importance, with the resulting selections able to achieve lower power estimation error than those of prior work coupled with decreases in area, power and modelling complexity. In particular, by monitoring just eight signals per module (similar to 0.3% of the total) across the 15 we examined, we demonstrate how to achieve runtime module-level estimation errors 1.5-6.9 x lower than when reliant on the signal selections made in accordance with a more straightforward, previously published metric.
更多查看译文
关键词
runtime power estimation,power consumption,subsystem level,adaptive energy-saving techniques,power gating,runtime task mapping,dynamic voltage,frequency scahng,arbitrary hardware system,automatic analysis,circuit structure,vectored simulation,high-quality rankings,lower power estimation error,runtime module-level estimation errors,signal selection,STRIPE
AI 理解论文
溯源树
样例
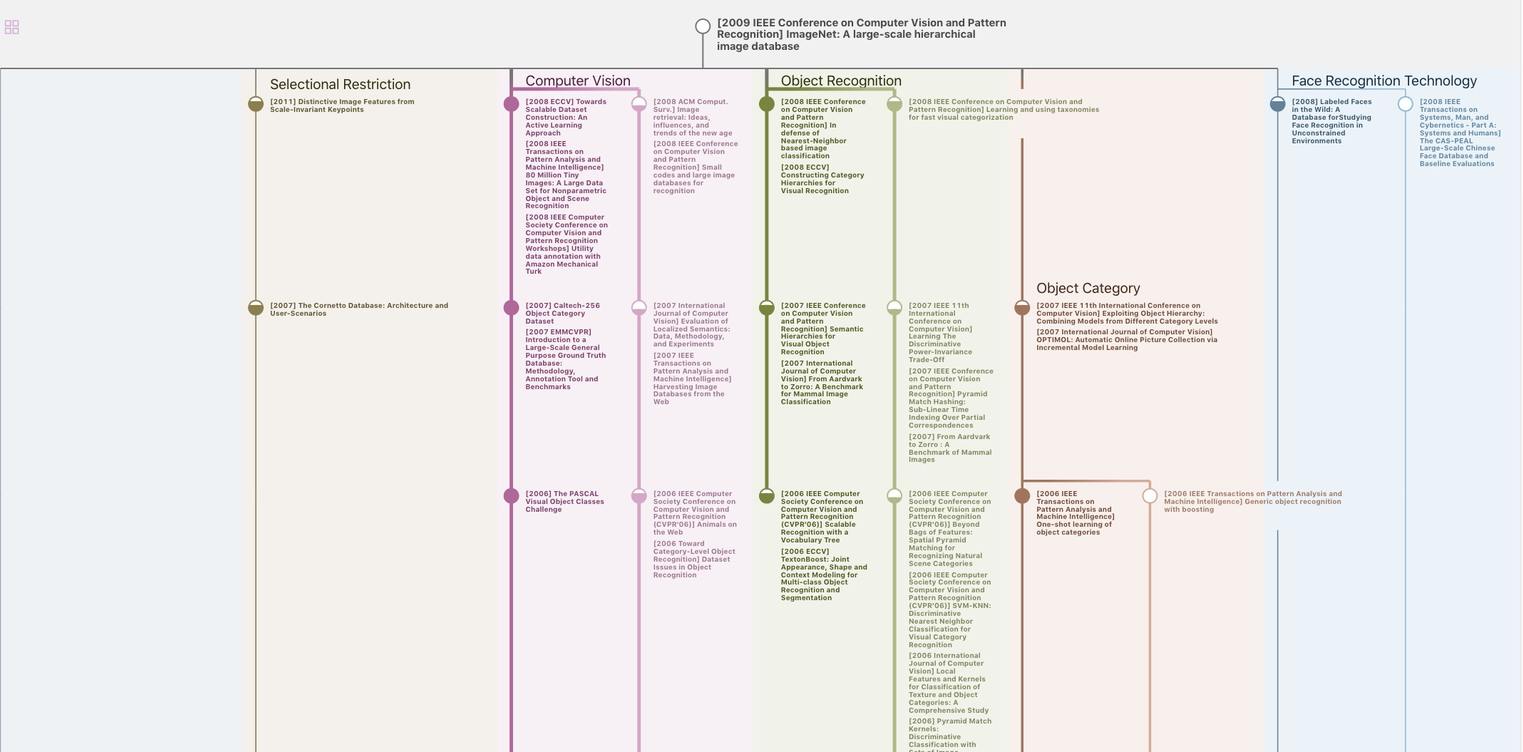
生成溯源树,研究论文发展脉络
Chat Paper
正在生成论文摘要