Quantifying the Potential Benefits of On-chip Near-Data Computing in Manycore Processors
2017 IEEE 25th International Symposium on Modeling, Analysis, and Simulation of Computer and Telecommunication Systems (MASCOTS)(2017)
摘要
Increasing data set sizes motivate for a shift of focus from computation-centric systems to data-centric systems, where data movement is treated as a first-class optimization metric. An example of this emerging paradigm is in-situ computing in largescale computing systems. Observing that data movement costs are increasing at an exponential rate even at a node level (as a node itself is fast-becoming a large manycore system), this paper provides a limit study of near-data computing within a manycore chip. Specifically, it makes the following two contributions. First, it quantifies the potential performance benefits of three incarnations of the near-data computing paradigm under the assumption of zero on-chip network latency and an infinite number of extra cores for offloading computations close to data they require. Our detailed experimental evaluation indicates that the most successful of these incarnations can boost the performance of the original execution by as much as 75%. The second contribution of this paper is an investigation of more realistic schemes that can approximate the potential savings achieved by perfect near-data computing. Our results demonstrate performance improvements ranging between 44% and 52%, over the original execution. We also discuss the pros and cons of each of these realistic schemes, and point to further research directions.
更多查看译文
关键词
Near-data Computing,Manycore processors,Processing In-Memory
AI 理解论文
溯源树
样例
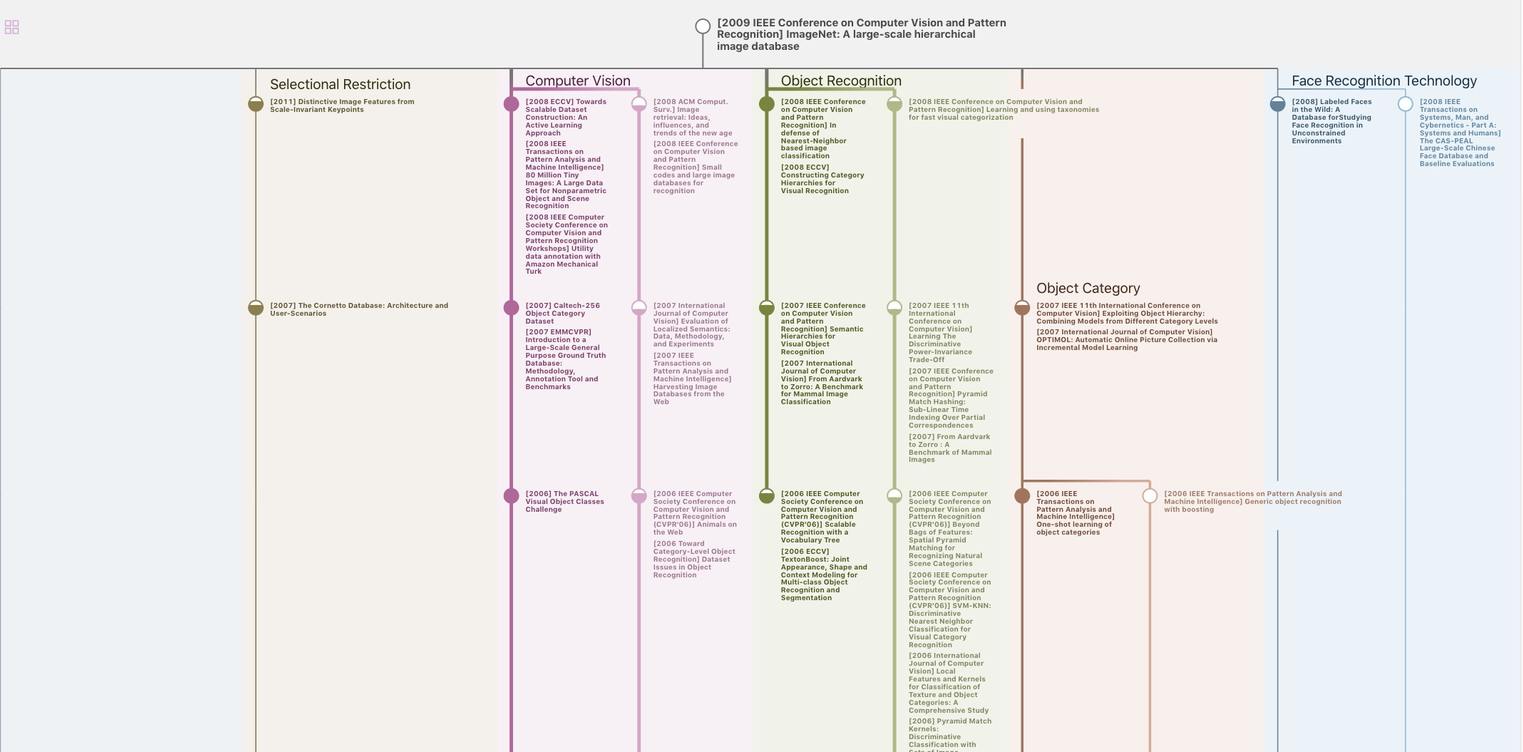
生成溯源树,研究论文发展脉络
Chat Paper
正在生成论文摘要